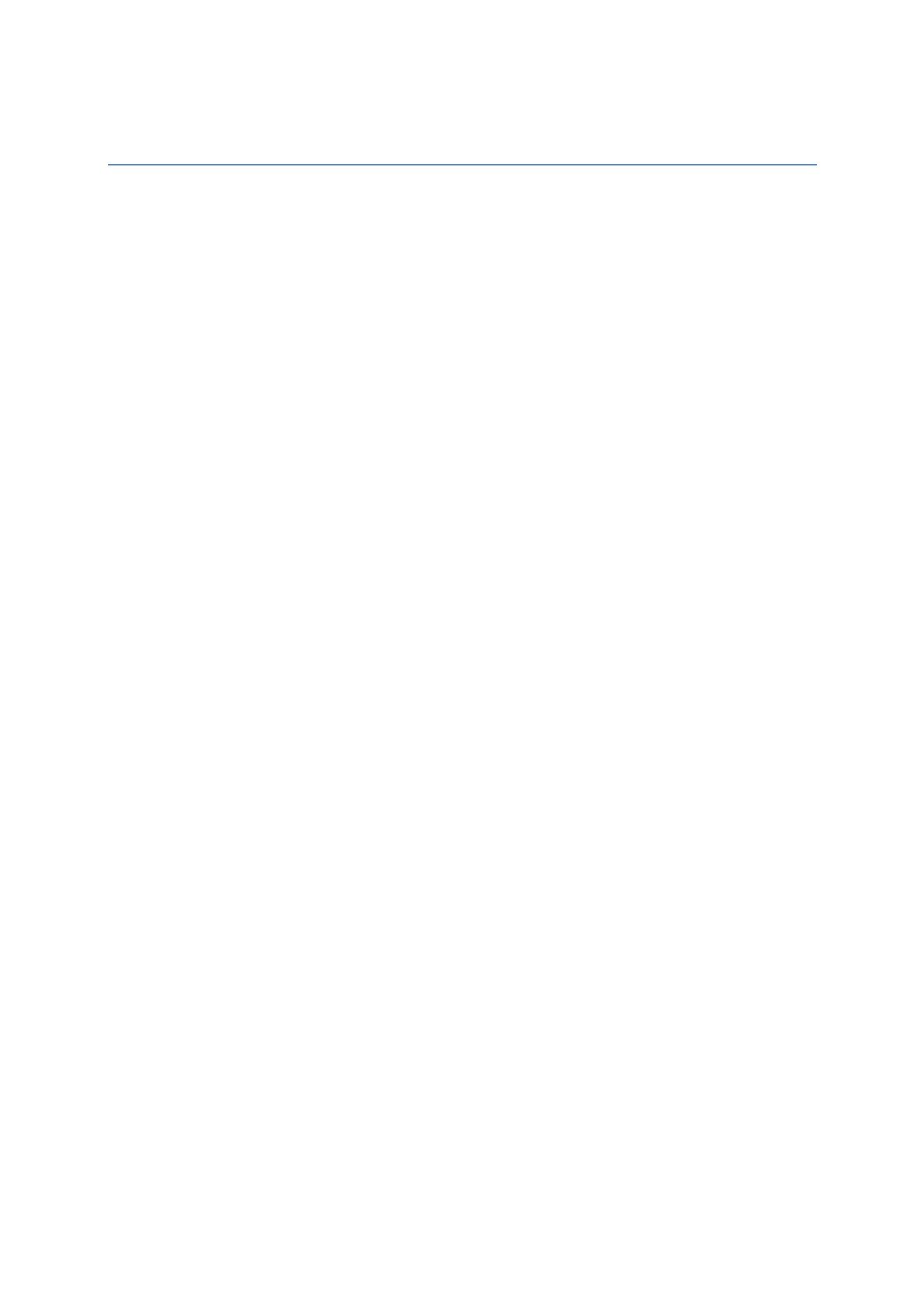
Aknowledgments
Les travaux présentés dans cette thèse ont été effectués au Laboratoire d'Analyse et
d'Architecture des Systèmes du Centre National de la Recherche Scientifique (LAAS-CNRS)
au sein du groupe Diagnostic, Supervision et Conduite (DISCO). Je tiens pour cela à
remercier les directeurs successifs du LAAS, Messieurs Raja CHATILLA, Jean-Louis
SANCHEZ et Jean ARLAT de m’avoir accueilli au LAAS-CNRS.
Je tiens à remercier aussi Madame Louise TRAVE-MASSUYES, responsable du groupe
DISCO, de m'avoir accueilli dans son groupe de recherche pendant ces années de thèse.
J’exprime toute ma gratitude à ma Directrice de thèse, Madame Marie-Véronique LE LANN,
pour avoir encadré mes travaux de recherche, et à qui cette thèse doit beaucoup. Je la remercie
pour sa disponibilité constante et efficace, son soutien et ses encouragements. Ma
reconnaissance va aussi à ma co-directrice de thèse, Madame Florence DALENC, pour ses
conseils précieux, sa patience et la confiance qu'elle m'a accordée pour mener à terme ces
travaux de recherche. Je tiens également à remercier Monsieur Joseph AGUILAR-MARTIN
pour ses remarques, ses conseils, sa disponibilité et son soutien tout au long de cette thèse.
Mes remerciements vont aussi à Madame Tatiana KEMPOWSKY-HAMON pour l’aide
qu’elle a apportée pour la réalisation de ces travaux de thèse.
Lire et juger une thèse n’est pas une tâche aisée à accomplir. Aussi, je tiens tout
particulièrement à exprimer ma reconnaissance à tous les membres du jury de ma thèse:
• Boutaib DAHHOU, professeur à l’Université Paul Sabatier, pour avoir présidé ce jury.
• Sylvie GALICHET, Professeur à l’Université de Savoie, et Sylvie CHARBONNIER,
Maître de Conférences à l’Université Joseph Fourier, pour m’avoir fait l’honneur
d’accepter la lourde tâche de rapporter sur cette thèse.
• Isabelle BLOCH, Professeur à Télécom Paris Tech, et Gille FAVRE, Professeur et
Praticien hospitalier à l’Université Paul Sabatier, d’avoir accepté de juger mes travaux
de thèses et pour leurs remarques pertinentes.
• Yijun SUN, assistant scientist (University of Florida), pour sa lecture pertinente du
manuscrit de thèse et pour m’avoir accueilli dans son laboratoire (ICBR) dans le cadre
d’un séjour de recherche.
Un GRAND merci à ma famille qui m’a soutenu pendant 28 ans de prés et de loin.
Finalement, je ne saurai conclure sans remercier mes amis pour leur soutien inconditionnel.