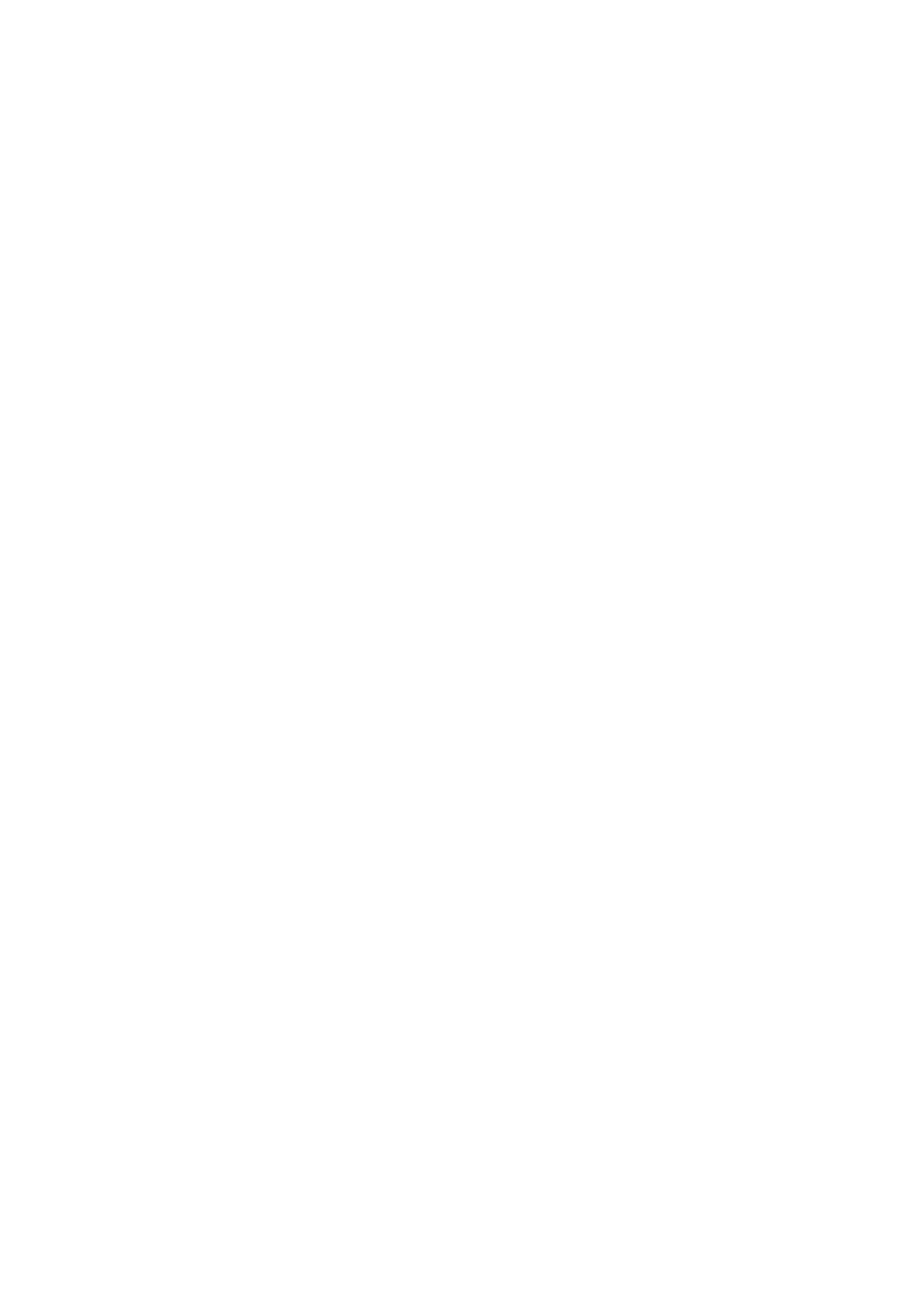
Abstract
For the last two decades, the field of Neuromorphic Engineering has attempted to emulate
the principles observed in neural systems in Very Large Scale Integration (VLSI) technology.
One endeavor of Neuromorphic Engineering is to mimic biological neural information processing
systems by implementing electronic analogs of spiking neurons as computational primitives.
Neuromorphic Engineering plays an important role in testing and validating hypotheses from the
neuroscience community while developing novel computational architectures for solving practical
problems in real-time.
Although Neuromorphic Engineering is clearly progressing from a technical point of view, practi-
cal applications are hindered by two unsolved problems: 1) the technical difficulty of configuring
the parameters of the silicon neurons to match a given theoretical model and 2) the lack of
general-purpose computational models that can be mapped onto spiking neural networks.
This thesis provides solutions to these two problems using a special neural circuit inspired by
the cortex: the Soft Winner–Take–All (sWTA). Similar to how transistors and logic gates have
become the elementary computational units of general-purpose digital processors, neuromorphic
spiking multi-neuron chips implementing sWTA have been proposed as the elementary units
which could be composed into a general-purpose neuromorphic processor. The goal of this thesis
is to define the first configuration language which can map a high-level computational model
onto VLSI neuromorphic spiking neurons, starting from their low-level parameters, and thus
raise the possibility of using multi-neuron neuromorphic chips as general-purpose processors.
To achieve this goal, I first deal with the technical difficulty of configuring the parameters of
the silicon neurons. In particular, I describe a model-based parameter translation technique
for systematically mapping the parameters of theoretical models of spiking neurons onto the
bias voltages and currents used to configure the multi-neuron chips. In addition, using Dy-
namic Parameter Estimation (DPE), a novel technique that utilizes synchronization as a tool
for dynamically coupling experimentally measured data to its corresponding model, I can use
transients in neural activity to efficiently estimate all the parameters of spiking neural networks.
These two techniques allow me to systematically measure and set the key properties of a VLSI
sWTA network.
However, configuring the properties of the individual VLSI sWTA network in a multi-sWTA
system is not sufficient to guarantee global stability. For determining boundary conditions for
which large neural systems are guaranteed to remain stable, I combine the use of contraction
theory with the Linear Threshold Unit (LTU) formalism for modeling spiking neurons. In con-
traction theory, these boundary conditions can be expressed solely in terms of the key properties
v