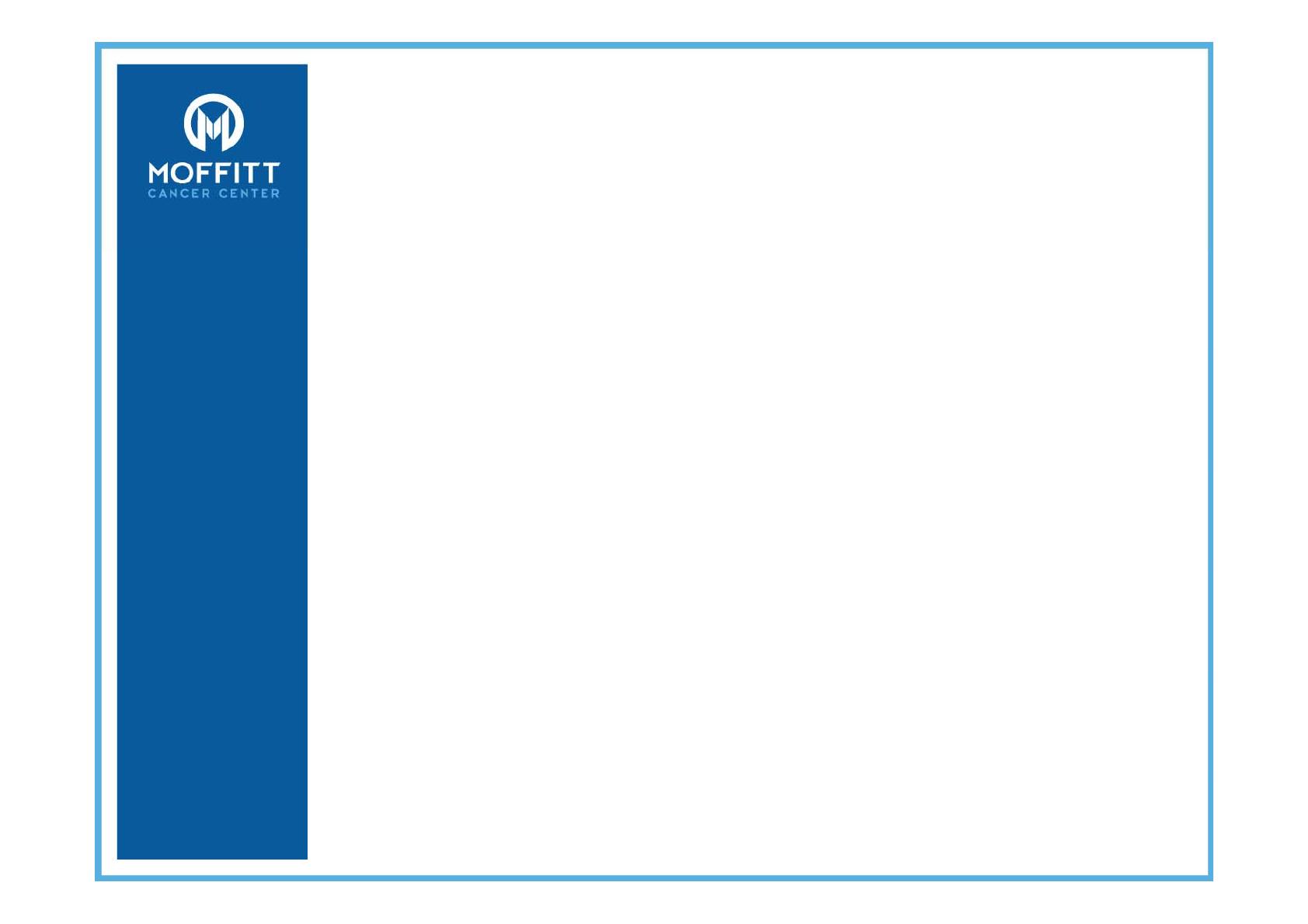
Tailored to Cancer and Cognition
1. Does cognitive performance change among
persons with cancer?
Is there variability in rate of change in
cognition across persons?
Interrelationships in behavioral change
.
4. Causes of intraindividual change
5. Causes of interindividual differences in
intraindividual chan
e
Baltes PB, Nesselroade JR: History and rationale of longitudinal research, in Nesselroade JR,
Baltes PB (eds): Longitudinal research in the study of behavior and development. New York, NY,
Academic Press, 1979, pp 1-39