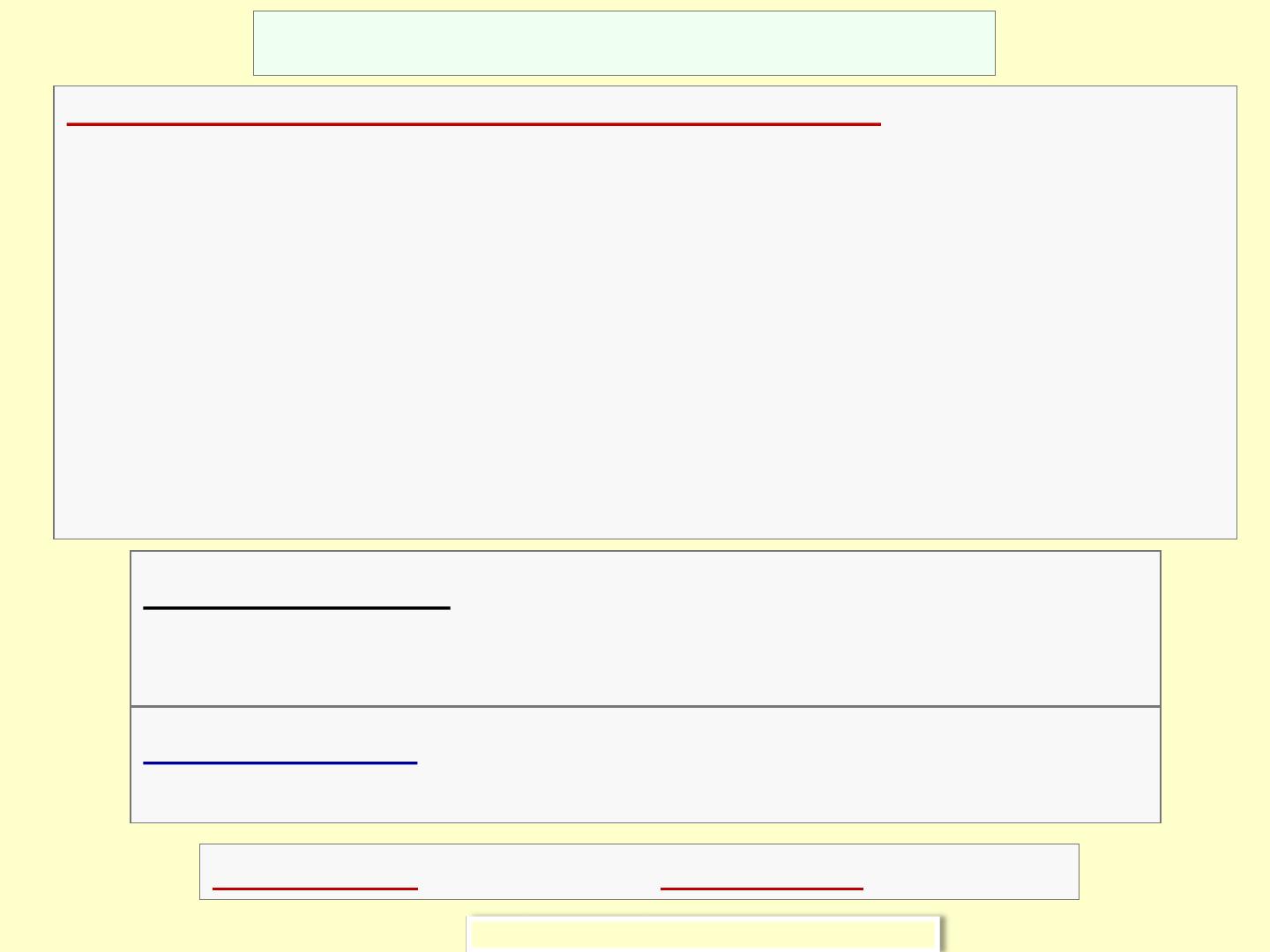
4
Lien entre la loi exponentielle et le processus de Poisson
X nombre de réalisations processus de Poisson d’intensité λ(fenêtre longueur 1)
X ~ Po (λ) λ= nombre moyen de réalisations par unité de temps (espace)
P( X = x) = λxexp (-λ) / x ! x= 0, 1, 2, 3, …
Y = nombre de réalisations processus de Poisson dans une fenêtre de longueur t
alors Y ~ Po (λt )
T = temps d’attente avant la prochaine réalisation après une réalisation
v.a sur (o, ∞)P( T > t ) = P[Y = 0 sur ( 0, t) ]= P( Y = 0 ) = exp (- λt )
alors T suit loi exponentielle de paramètre λT ~ Exp (λ)
fonction de répartition de T FT( t ) = 1 -P ( T > t )
= 0 si t < 0
= 1 - exp (-λt ) si t ≥ 0
fonction de densité de T fT(t) = (/) FT(t) = 0 si t < 0
=λexp (-λt ) si t ≥ 0
moyenne de T E(T) = 1/ λÉcart type de T ET(T) = 1/ λ
Bernard CLÉMENT, PhD
distribution EXPONENTIELLE : T ~ Exp (λ)
MTH2302 Probabilités et méthodes statistiques