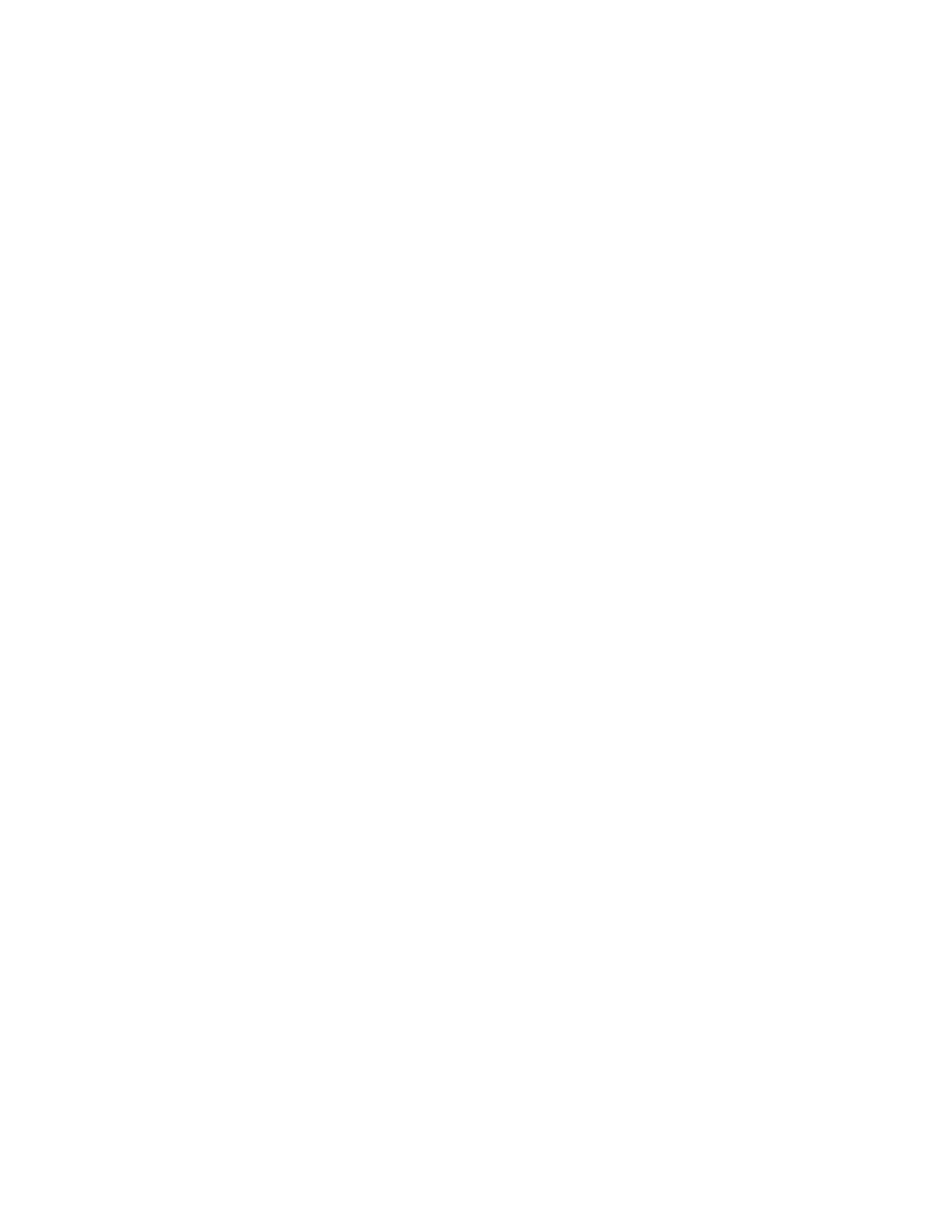
iv
RÉSUMÉ
La publicité en ligne est devenue une ressource économique importante et indispensable pour
de nombreux services en ligne. Cependant, on note que ce marché est particulièrement touché
par la fraude et notamment la fraude au clic. Ainsi, en 2015, il est estimé que, dans le monde,
les annonceurs allaient perdre plus de sept milliards de dollars américains en raison de la
fraude publicitaire.
Les méthodes de luttes actuelles contre la fraude publicitaire sont concentrées sur la détection
de logiciels malveillant et le démantèlement des réseaux de machines zombies qui y sont
associés. Bien qu’indispensables pour limiter le nombre d’infections, ces démantèlements ne
diminuent pas l’attrait pour cette fraude. Il est donc indispensable de s’attaquer en plus à
l’incitatif économique. Pour cela, nous avons d’une part essayé de mieux comprendre l’éco-
système de la fraude au clic et d’autre part évalué des possibilités de perturbations de cet
écosystème afin de diminuer l’attractivité de la fraude.
Dans un premier temps, nous avons collecté des données réseau générées par un logiciel
malveillant de fraude au clic, Boaxxe. Ces données sont des chaînes de redirection HTTP
qui montrent les liens entre les différents acheteurs et revendeurs d’une publicité, c’est-à-dire
la chaîne de valeur. Celles-ci commencent au moteur de recherche d’entrée, opéré par des
fraudeurs, passe à travers plusieurs régies publicitaires et termine sur le site d’un annonceur,
celui ayant acheté le trafic.
Dans un second temps, nous avons agrégé les données collectées afin de constituer un graphe
montrant les relations entre les différents noms de domaine et adresses IP. Ce graphe est
ensuite consolidé, grâce à des données de source ouverte, en regroupant les nœuds réseaux
appartenant au même acteur. Le graphe ainsi obtenu constitue une représentation de l’éco-
système de la fraude au clic de Boaxxe.
Dans un troisième temps, nous avons évalué différentes stratégies de perturbation de l’éco-
système. L’objectif de la perturbation est d’empêcher la monétisation de trafic généré par
Boaxxe, c’est-à-dire d’empêcher le transit du trafic du moteur de recherche vers le site de
l’annonceur. Il s’avère que la stratégie la plus adaptée à notre problème est celle utilisant la
méthode du Keyplayer. Nous avons ainsi montré qu’il était possible de protéger un nombre
important d’annonceurs en supprimant un faible nombre d’intermédiaires.
Enfin, nous discutons des possibilités de mise en pratique de l’opération de perturbation.
Nous insistons sur le fait qu’il est important de sensibiliser les annonceurs à la fraude afin