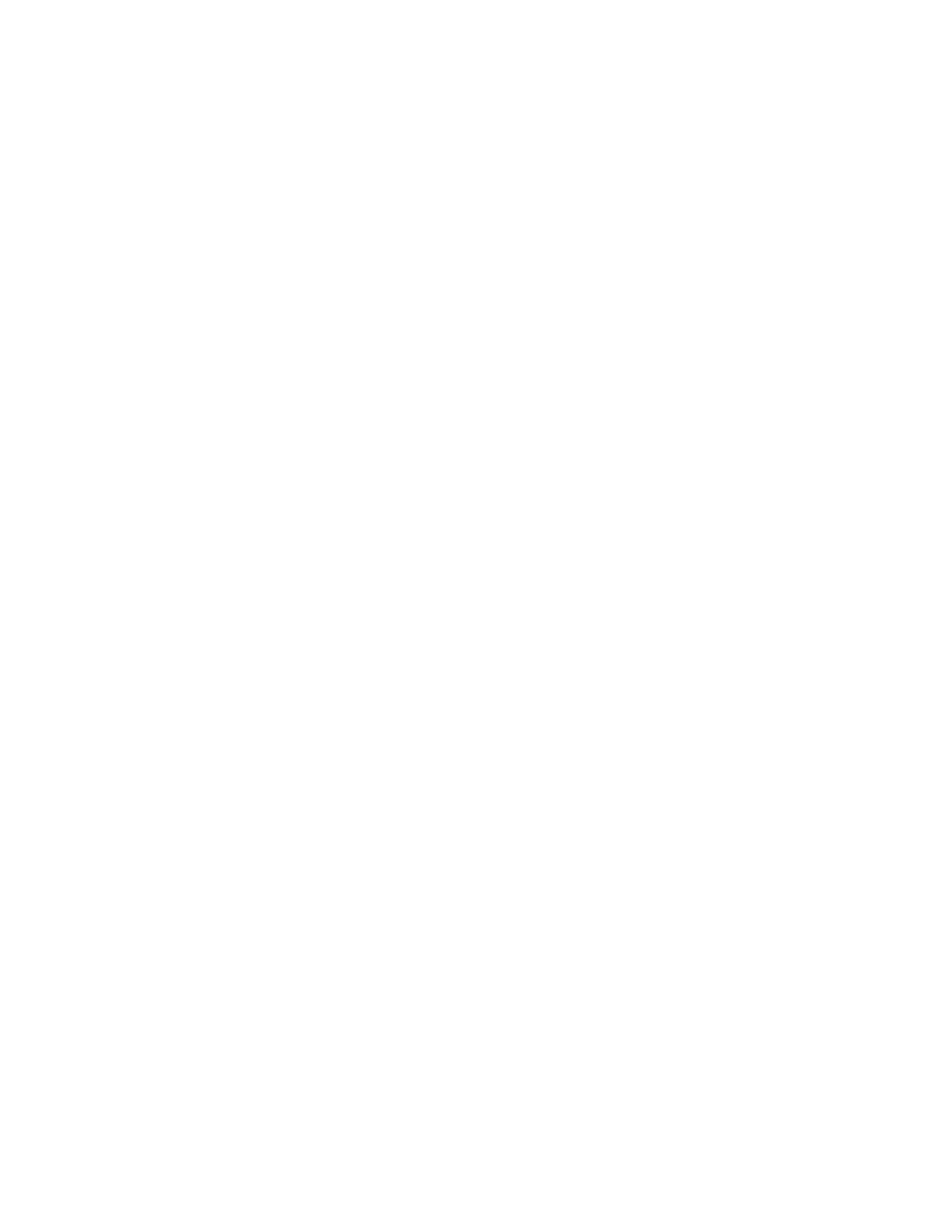
Acknowledgments
It is a great pleasure to thank Professor Giovanni Barone-Adesi, my thesis advisor, for invaluable
suggestions and discussions and for his support during the last three years.
I am grateful to Professor Paolo Vanini for his useful comments and suggestions and for accepting
to be a member of the thesis committee.
I really wish to thank Professor Marc Chesney for accepting to be co-examiner of this work.
Thanks go to my friends Francesca Bellini, Ettore Croci, Silvia Cappa, Talia Cicognani, Clizia
Lonati and Silvia Zerbeloni for making everyday life enjoyable.
My gratitude to my mom Silvana and to my brother Renato for their continuous support during
these years.
Specially thanks go to Loriano for his love and support and for always enriching my life.
ii