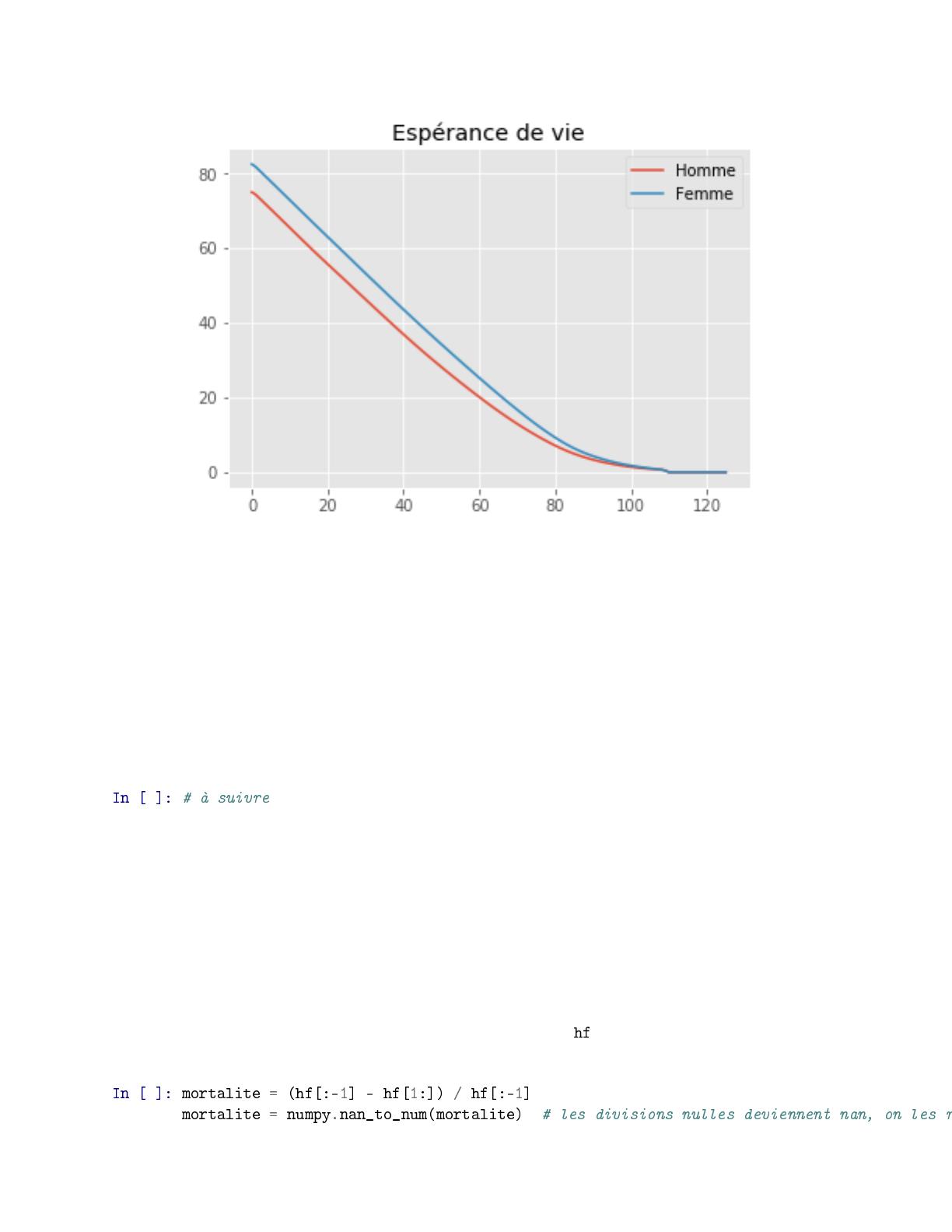
Le calcul implémenté ci-dessus n’est pas le plus efficace. On fait deux boucles imbriquées dont le
coût global est en O(n2)mais surtout on effectue les mêmes calculs plusieurs fois. Pour le réduire
à un coût linéaire O(n), il faut s’intéresser à la quantité :
Pt+1E(t+1)−PtE(t) =
∞
∑
d=1
d(Pt+d+1−Pt+d+2)−
∞
∑
d=1
d(Pt+d−Pt+d+1)
L’implémentation devra utiliser la fonction numpy.cumsum et cette astuce Pandas Dataframe
cumsum by row in reverse column order?.
numpy et pandas ont plusieurs fonction en commun dès qu’il s’agit de parcourir les données. Il
existe aussi la fonction DataFrame.cumsum.
1.0.3 Exercice 3 : simulation de la pyramide l’année suivante
L’objectif est d’estimer la population française à l’année T. Si P(a,T)désigne le nombre de per-
sonnes d’âge aen T, on peut estimer P(a,T+1)en utilisant la probabilité de mourir m(a):
P(a+1, T+1) = P(a,T)∗(1−m(a))
On commence par calculer les coefficients m(a)avec la table obtenue lors de l’exercice précé-
dent tout en gardant la même dimension (on aura besoin de la fonction nan_to_num :
5