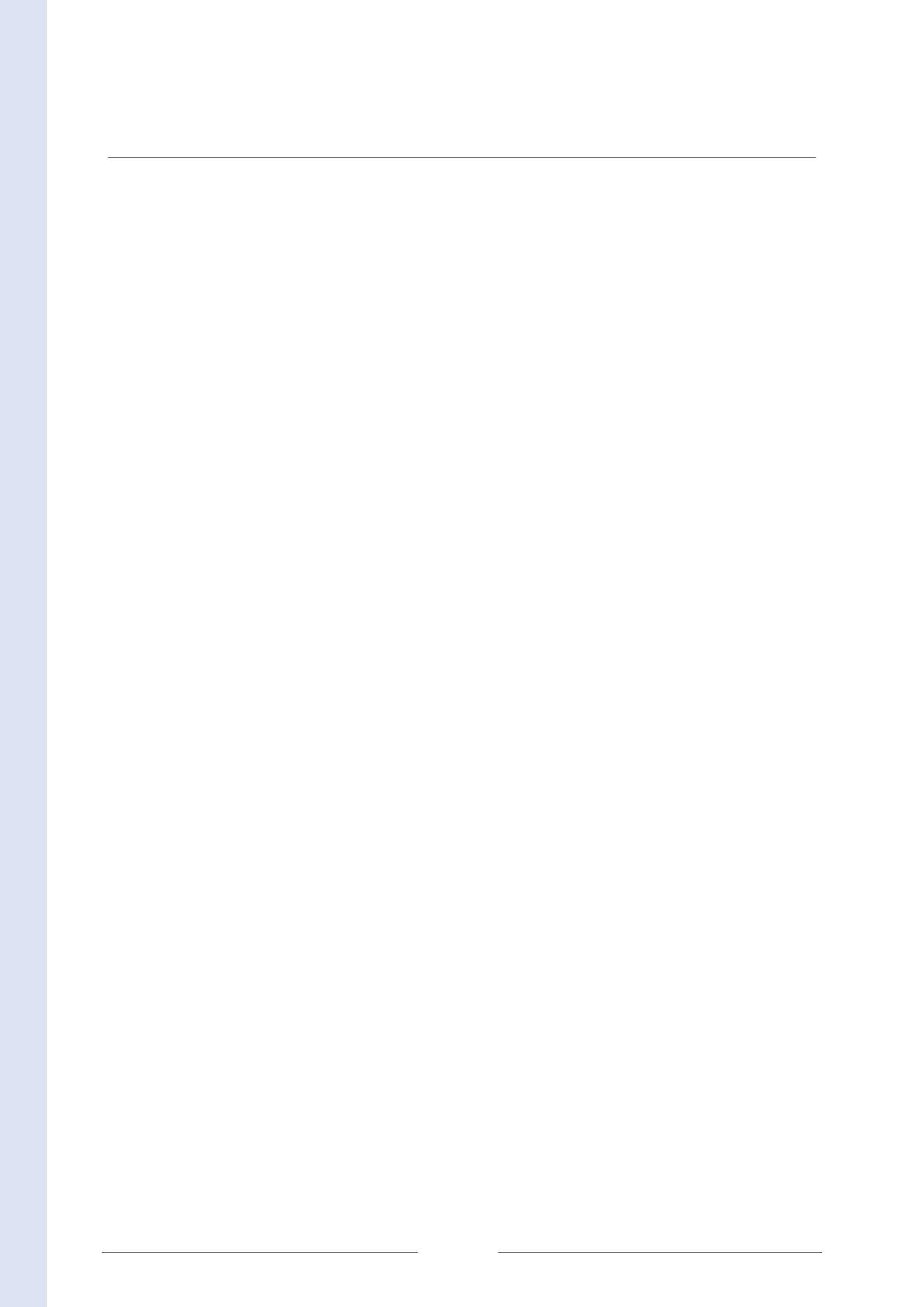
Abstract
Over 40,000 sudden cardiac deaths (SCD) occur per year in France. Implantable
patients at high risk of SCD. Unfortunately, most implantations to date appear unnecessary:
according to a recent study, 81% of the implanted ICDs were not used during the first five years
following the implantation. This result raises an important issue because of the perioperative and
postoperative risks. It has been shown in two studies that 13% to 17% of ICD-implanted
patients have undergone at least one inappropriate shock (i.e. a shock generated by the
-up periods; these shocks
are known to be detrimental to the cardiac muscle. Thus, it is important to improve the selection
of the candidates to ICD implantation in primary prevention.
Risk stratification for sudden cardiac death based on long-term electrocardiographic
(Holter) recordings has been extensively performed in the past, without resulting in a significant
improvement of the selection of candidates to ICD implantation. The present report describes a
nonlinear multivariate analysis of Holter recording indices. We computed all the descriptors
available in the Holter recordings present in our database. The latter consisted of labelled Holter
recordings of patients equipped with an ICD in primary prevention; a fraction of these patients
received at least one appropriate therapy from their ICD during a 6-month follow-up. Based on
physiological knowledge on arrhythmogenesis, feature selection was performed, and an
innovative procedure of classifier design and evaluation was proposed. The classifier is intended
to discriminate patients who are really at risk of sudden death from patients for whom ICD
implantation does not seem necessary. In addition, we designed an ad hoc classifier that
capitalizes on prior knowledge on arrhythmogenesis.
We conclude that improving prophylactic ICD-implantation candidate selection by
automatic classification from Holter recording features may be possible. Nevertheless, that
statement should be supported by the study of a more extensive and appropriate database. This is
mandatory for decreasing the rate of false negatives (i.e. the proportion of patients who are not
deemed to be at risk although they actually are), hence increasing the negative predictive value of
our method.
Keywords:
sudden cardiac death, implantable cardioverter defibrillator, primary prevention,
, machine learning, feature selection, neural network.
pastel-00939082, version 1 - 30 Jan 2014