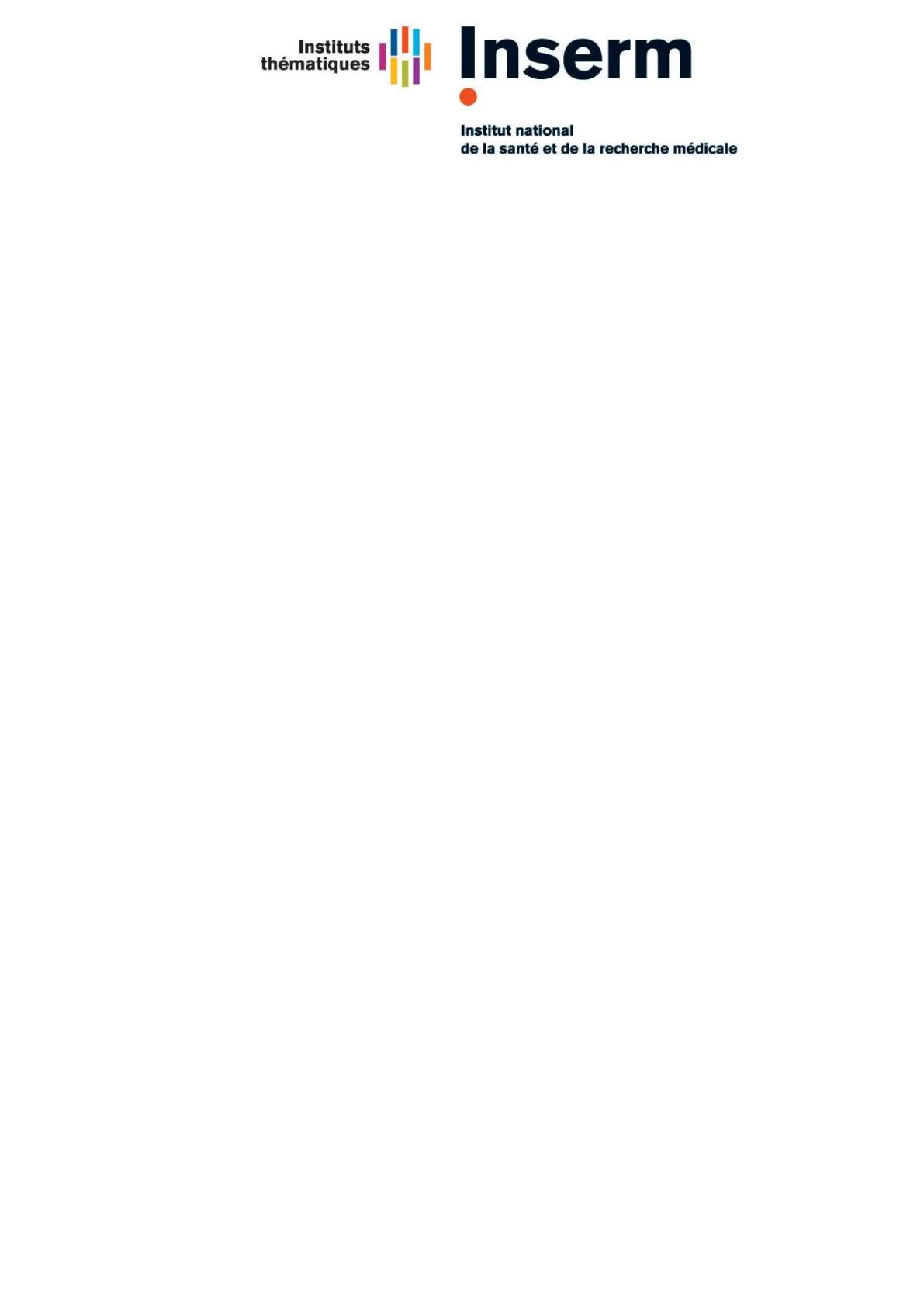
4. Buyse, M., Burzykowski, T., Carroll, K., Michiels, S., Sargent, D. J., Miller, L. L., Elfring,
G. L., et al. (2007). Progression-free survival is a surrogate for survival in advanced
colorectal cancer. Journal of Clinical Oncology, 25(33), 5218–5224.
5. Cook, R. J., & Lawless, J. F. (2001). Some comments on e ciency gains from auxiliary
information for right-censored data, 96, 191–202.
6. Faucett, C. L., Schenker, N., & Taylor, J. M. G. (2002). Survival analysis using auxiliary
variables via multiple imputation, with application to AIDS clinical trial data. Biometrics,
58(1), 37–47.
7. Fleming, T. R., Prentice, R. L., Pepe, M. S., & Glidden, D. V. (1994). Surrogate and
auxiliary endpoints in clinical trials, with potential applications in cancer and aids
research. Statistics in medicine, 13(9), 955–968.
8. Hsu, C., & Taylor, J. M. G. (2009). Nonparametric comparison of two survival functions
with dependent censoring via nonparametric multiple imputation, (November 2008),
462–475. doi:10.1002/sim
9. Huang, X., & Liu, L. (2007). A joint frailty model for survival and gap times between
recurrent events. Biometrics, 63(2), 389–397.
10. Kalbfleisch, J. D., Schaubel, D. E., Ye, Y., & Gong, Q. (2013). An Estimating Function
Approach to the Analysis of Recurrent and Terminal Events. Biometrics, 1–9.
doi:10.1111/biom.12025
11. Li, Y., Taylor, J. M. G., Elliott, M. R., & Sargent, D. J. (2011). Causal assessment of
surrogacy in a meta-analysis of colorectal cancer trials. Biostatistics (Oxford, England),
12(3), 478–92. doi:10.1093/biostatistics/kxq082
12. Mauguen, A., Rachet, B., Mathoulin-Pélissier, S., MacGrogan, G., Laurent, A., &
Rondeau, V. (2013). Dynamic prediction of risk of death using history of cancer
recurrences in joint frailty models. Statistics in medicine, 32(30), 5366–80.
doi:10.1002/sim.5980
13. Mazroui, Y., Mathoulin-Pélissier, S., Macgrogan, G., Brouste, V., & Rondeau, V. (2013).
Multivariate frailty models for two types of recurrent events with a dependent terminal
event : Application to breast cancer data. Biometrical Journal, 55(6), 886–884.
doi:10.1002/bimj.200100000
14. Mazroui, Y., Mathoulin-Pélissier, S., Soubeyran, P., & Rondeau, V. (2012). General joint
frailty model for recurrent event data with a dependent terminal event: Application to
follicular lymphoma data. Statistics in Medicine, 897(December 2010), n/a–n/a.
doi:10.1002/sim.4479
15. Michiels, S., Le Maître, A., Buyse, M., Burzykowski, T., Maillard, E., Bogaerts, J.,
Vermorken, J. B., et al. (2009). Surrogate endpoints for overall survival in locally
advanced head and neck cancer: meta-analyses of individual patient data. The lancet
oncology, 10(4), 341–50. doi:10.1016/S1470-2045(09)70023-3
16. Murray, S., & Tsiatis, a a. (2001). Using auxiliary time-dependent covariates to recover
information in nonparametric testing with censored data. Lifetime data analysis, 7(2),
125–41.
17. Pignon, J. P., le Maitre, A., Maillard, E., & Bourhis, J. (2009). Meta-analysis of
chemotherapy in head and neck cancer (MACH-NC): an update on 93 randomised trials
and 17,346 patients. Radiotherapy & Oncology, 92(1), 4–14.
18. Pignon, J.-P., le Maître, A., & Bourhis, J. (2007). Meta-Analyses of Chemotherapy in
Head and Neck Cancer (MACH-NC): an update. International journal of radiation
oncology, biology, physics, 69(2 Suppl), S112–4. doi:10.1016/j.ijrobp.2007.04.088
19. Prentice, R. L. (1989). surrogate endpoints in clinical trials: definition and operational
criteria. Statistics in medicine, 8, 431–440.
20. Proust, C., & Jacqmin-Gadda, H. (2005). Estimation of linear mixed models with a
mixture of distribution for the random effects. Computer methods and programs in
biomedicine, 78(2), 165–73. doi:10.1016/j.cmpb.2004.12.004