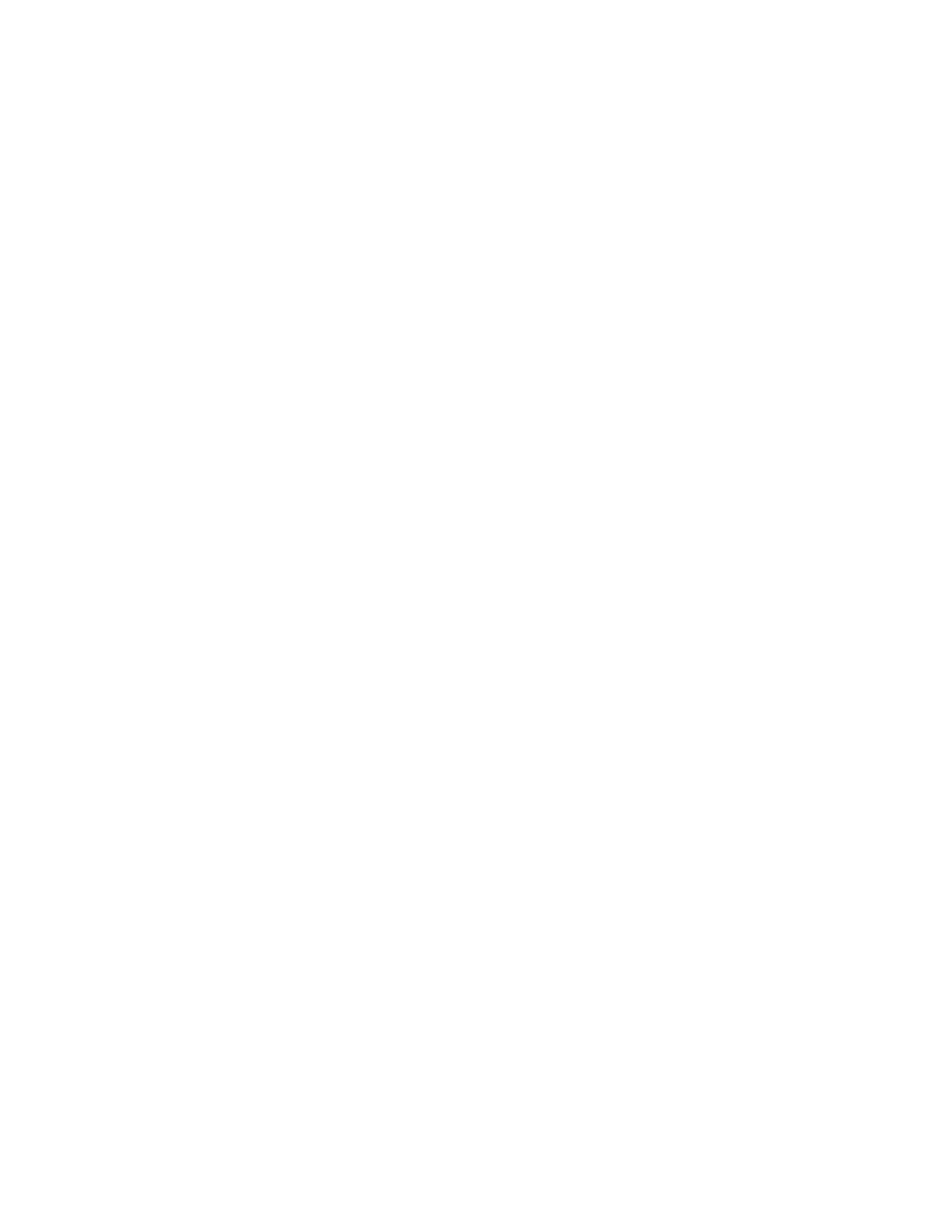
Abstract
Social media have greatly modified the way we produce, diffuse and consume
information, and have become powerful information vectors. The goal of this thesis
is to help in the understanding of the information diffusion phenomenon in social
media by providing means of modeling and analysis.
First, we propose MABED (Mention-Anomaly-Based Event Detection), a statistical
method for automatically detecting events that most interest social media users from
the stream of messages they publish. In contrast with existing methods, it doesn’t
only focus on the textual content of messages but also leverages the frequency of
social interactions that occur between users. MABED also differs from the literature in
that it dynamically estimates the period of time during which each event is discussed
rather than assuming a predefined fixed duration for all events. Secondly, we propose
T-BASIC (Time-Based ASynchronous Independent Cascades), a probabilistic model
based on the network structure underlying social media for predicting information
diffusion, more specifically the evolution of the number of users that relay a given
piece of information through time. In contrast with similar models that are also based
on the network structure, the probability that a piece of information propagate from
one user to another isn’t fixed but depends on time. We also describe a procedure
for inferring the latent parameters of that model, which we formulate as functions of
observable characteristics of social media users. Thirdly, we propose SONDY (SOcial
Network DYnamics), a free and extensible software that implements state-of-the-art
methods for mining data generated by social media, i.e. the messages published by
users and the structure of the social network that interconnects them. As opposed
to existing academic tools that either focus on analyzing messages or analyzing the
network, SONDY permits the joint analysis of these two types of data through the
analysis of influence with respect to each detected event.
The experiments, conducted on data collected on Twitter, demonstrate the rele-
vance of our proposals and shed light on some properties that give us a better un-
derstanding of the mechanisms underlying information diffusion. First, we compare
3