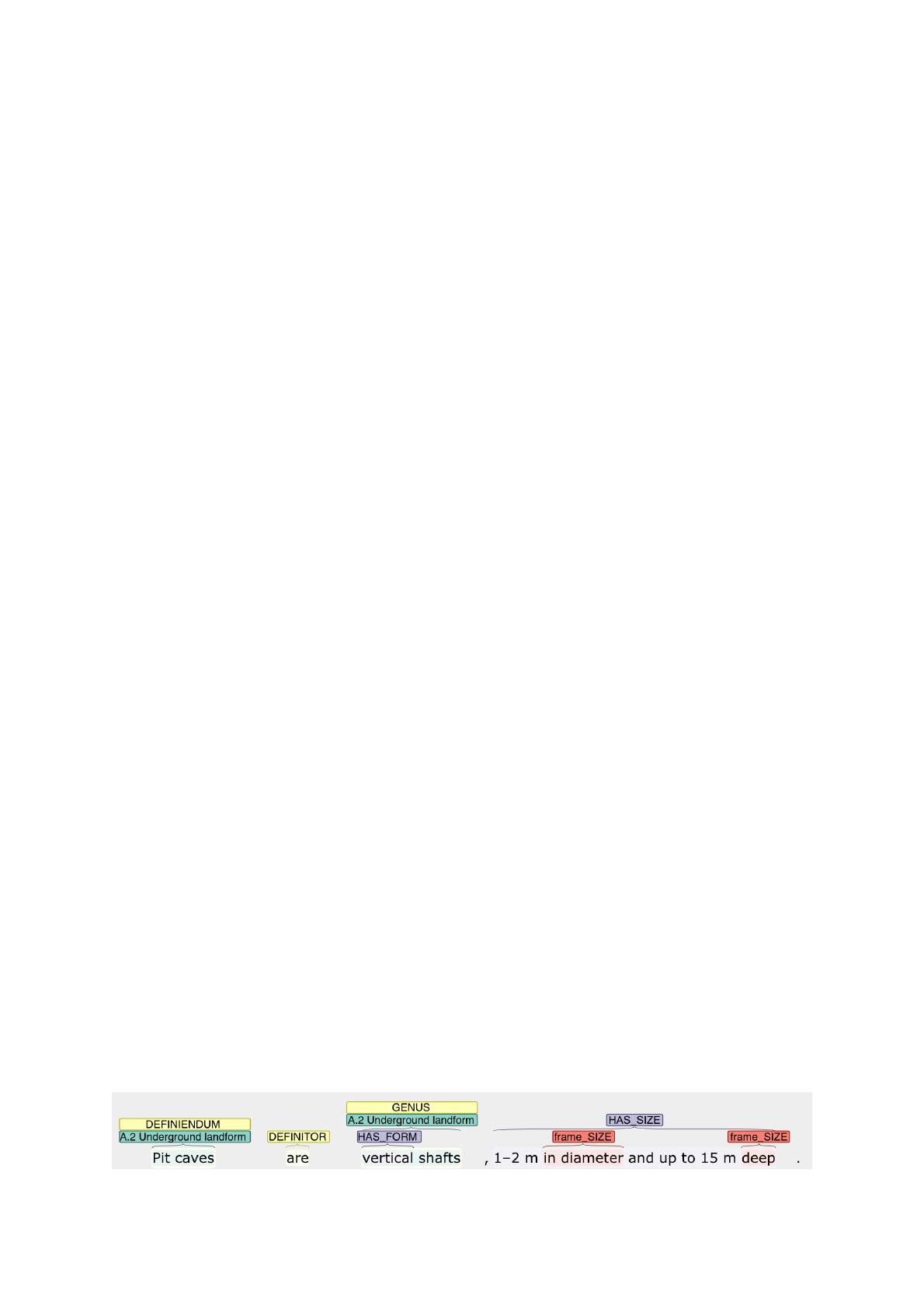
Modelling specialised knowledge with conceptual frames: the TermFrame approach to a
structured visual domain representation
Špela Vintar, Amanda Saksida, Uroš Stepišnik, Katarina Vrtovec
{spela.vintar, amanda.saksida, uros.stepisnik, katarina.vrtovec}@ff.uni-lj.si
Faculty of Arts, University of Ljubljana
Extended abstract
1. Introduction
The frame-based approach in terminology (Faber 2009; 2012) has provided a valuable new
framework for representing specialised knowledge by combining linguistic information derived from
specialised corpora with conceptual structures and by highlighting the fact that the cognitive frames
underlying specialised communication are dynamic, context- and culture dependent. The
TermFrame project reimplements, extends and adapts the above methodology to the domain of
karstology and a set of three languages, English, Slovene and Croatian, in order to propose a new
multi-purpose model of knowledge representation which can be used by linguists, terminologists,
experts and data scientists alike. We describe the TermFrame annotation framework, our annotated
corpora and an analysis of the conceptual frames which emerged from the semantically annotated
definitions. In particular we focus on cross-language differences and the use scenarios to adequately
represent and visualise complex domain-specific knowledge.
2. TermFrame corpora, definition extraction and annotation framework
For the purposes of our research, we built three corpora, Slovene, English and Croatian. The corpora
contain relevant, authentic and contemporary works on karstology and are comparable in the terms
of domain and text types included. The corpora comprise scientific texts (scientific papers, books,
articles, doctoral and master’s theses, glossaries and dictionaries) from the field of karstology, which
in itself is an interdisciplinary domain partly overlapping with geology, hydrology, speleology and
other fields.
Definition candidates were extracted automatically using ClowdFlows (Pollak et al. 2012), then
manually validated to retain only contexts with valuable explanatory information about the concept,
but not necessarily compliant with the traditional analytic definition structure. Since our domain of
interest is karstology, only definitions of concepts relevant to karst were considered.
The TermFrame annotation framework was developed within an interdisciplinary team consisting of
a domain expert, a terminologist and a cognitive linguist, and in collaboration with text mining
experts. Each definition is annotated on four layers:
- Definition element with the tags DEFINIENDUM, DEFINITOR, GENUS
- Semantic category, a taxonomically organised conceptual hierarchy adapted to karstology
with the following top-level nodes: A. Landform, B. Process, C. Geome, D. Entity and E.
Instrument/Method
- Relation, to mark the part of the definition where a specific property of the definiendum is
described; our framework defines 16 relations such as HAS_FORM, HAS_CAUSE, CONTAINS,
AFFECTS, HAS_SIZE etc.
- Relation frame, this level shall facilitate Machine Learning of knowledge patterns and marks
the phrase which introduces a specific type of relation.
Figure 1: Annotated sentence in the WebAnno tool