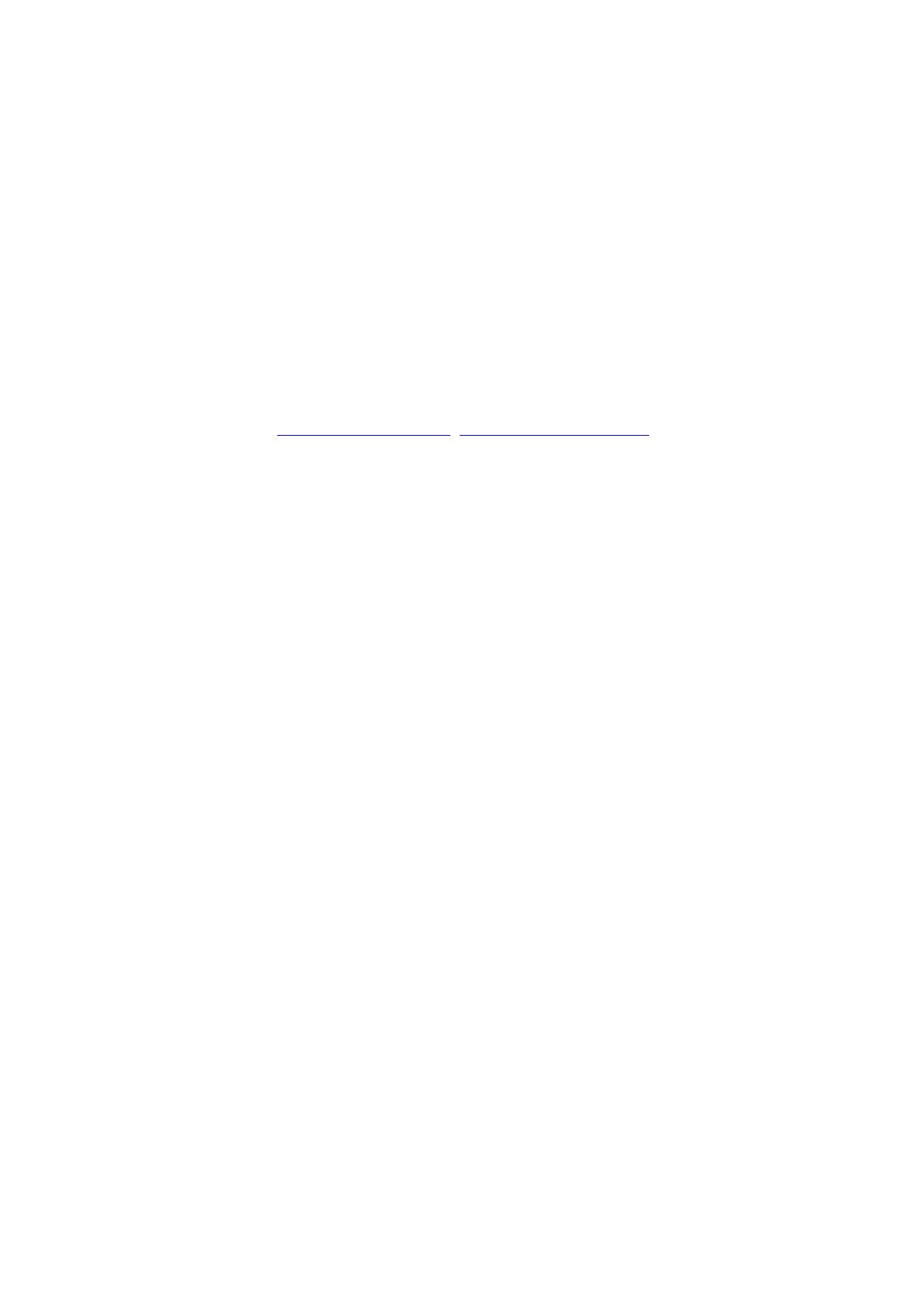
Knowledge-Guided Semantic Indexing of Breast Cancer Histopathology
Images
Adina Eunice Tutac
1,5
, Daniel Racoceanu
1,6
, Thomas Putti
2
, Wei Xiong
1.4
,
Wee-Kheng Leow
1,3
Vladimir Cretu
5
1
IPAL - UMI CNRS 2955 Singapore
2
NUH, Singapore,
3
NUS, Singapore,
4
I2R, A-STAR, Singapore,
5
Politehnica University of Timisoara, Romania,
6
University of Besançon, France
Abstract
Narrowing the semantic gap represents one of the
most outstanding challenges in medical image analysis
and indexing. This paper introduces a medical
knowledge – guided paradigm for semantic indexing of
histopathology images, applied to breast cancer
grading (BCG). Our method improves pathologists’
current manual procedures consistency by employing a
semantic indexing technique, according to a rule-based
decision system related to Nottingham BCG system.
The challenge is to move from the medical concepts/
rules related to the BCG, to the computer vision (CV)
concepts and symbolic rules, to design a future generic
framework- following Web Ontology Language
standards - for an semi- automatic generation of CV
rules. The effectiveness of this approach was
experimentally validated over six breast cancer cases
consisting of 7000 frames with domain knowledge from
experts of Singapore National University Hospital,
Pathology Department. Our method provides
pathologists a robust and consistent tool for BCG and
opens interesting perspectives for the semantic
retrieval and visual positioning.
1. I
NTRODUCTION
Within the last decade, histological grading [1] has
become widely accepted as a powerful indicator of
prognosis in breast cancer. Most grading systems
currently employed for breast cancer combine criteria in
nuclear pleomorphism, tubule formation and mitotic
counts. In general, each grading criteria is evaluated by
a score of 1 to 3 (3 being associated to the most serious
case) and the score of all three components are added
together to give the "grade”. Breast Cancer Grading
(BCG) [1] [2] requires time and attention while
classifying 100 cases/ day, each of them having around
2000 frames, as the pathologists usually do. Currently,
BCG is achieved by visual examinations of pathologists.
Such a manual work is time-consuming and
inconsistent. According to those issues, developing an
automatic grading system represents a strong medical
requirement.
Such an automatic grading system should naturally
be able to semantically index the images in line with the
medical domain knowledge, and inspired from their real
content. Content-based image indexing [3], [5] has been
subject of significant researches in the context of
medical imaging domain [4] [6]. Solving the issue of
the semantic gap [7] [8] between the low level features
[9], [10] and the high level semantic concepts [11]
represents the cutting edge research [12], [13].
In this paper, we propose a solution to meet
pathologist needs for automatic BCG. Beyond this, we
further model the BCG-related medical knowledge into
reasoning rules. These rules are embedded in semantic
indexing approaches.
The proposed method provides pathologists a robust
and consistent tool, as a second opinion, for breast
cancer grading, using the Nottingham grading system
[1] [2]. The effectiveness of the proposed approach has
been validated in experiments over six breast cancer
cases consisting of 7000 frames with domain
knowledge from pathologist experts.
The paper is organized as follows. In section 2,
domain knowledge analysis is introduced by describing
a synthesis of the breast cancer grading standard system
and showing the importance of grading in breast cancer
detection. Section 3 presents our grading approach
model with the medical image indexing inspired rules,
with the computation of local and global grading. The