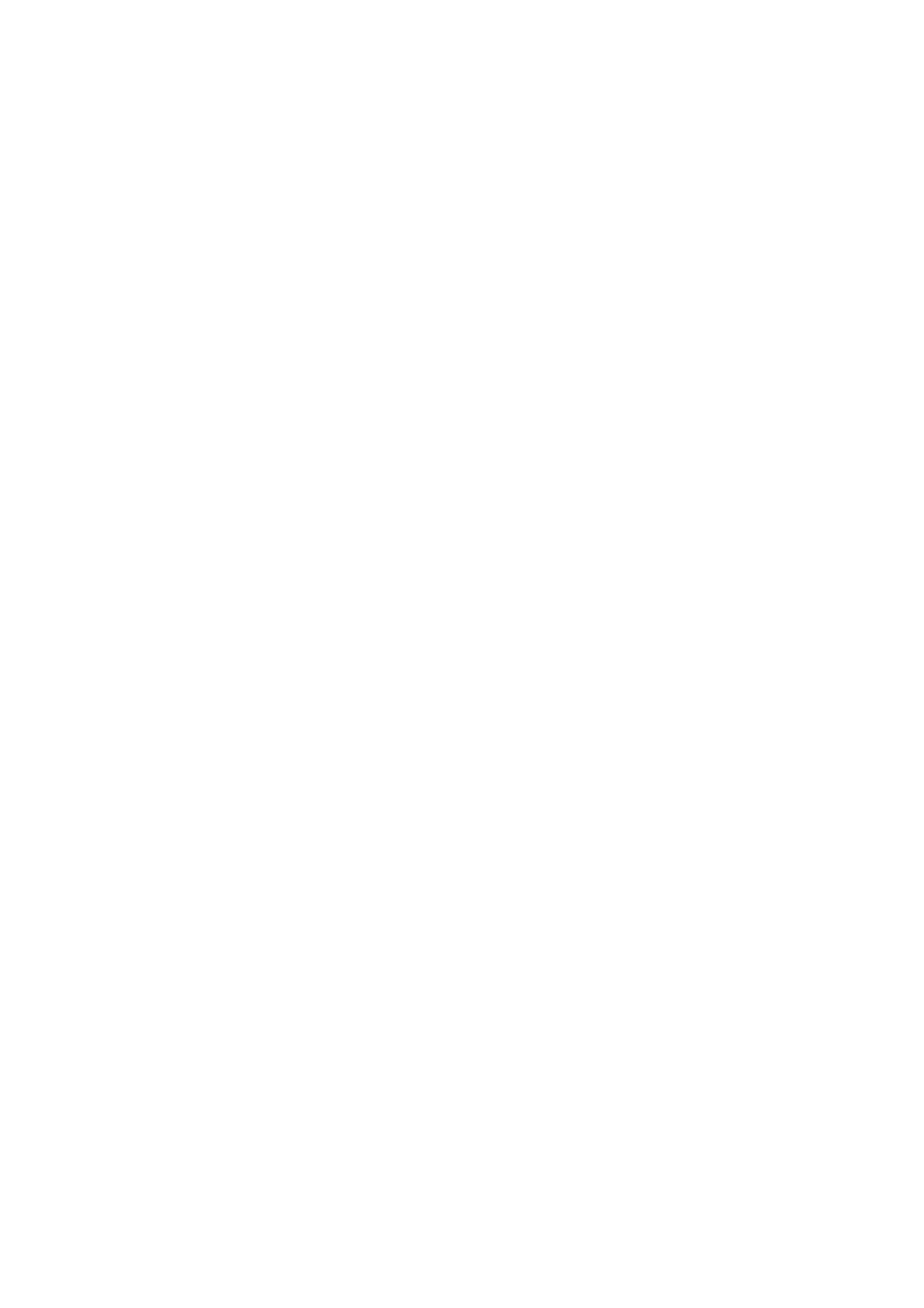
UNIVERSIDADE FEDERAL DO RIO GRANDE DO SUL
Centro de Biotecnologia da Universidade Federal do Rio Grande do Sul
Programa de Pós-Graduação em Biologia Celular e Molecular
JOEMERSON OSÓRIO ROSADO
ANÁLISE DE REDES DE INTERAÇÕES ENTRE DROGAS
QUIMIOTERÁPICAS USADAS NO TRATAMENTO DE CÂNCER GÁSTRICO:
EXPLORANDO PROTEÍNAS E PROCESSOS BIOLÓGICOS POR MEIO DE
FERRAMENTAS DE FARMACOLOGIA DE SISTEMAS
Dissertação apresentada ao Programa de
Pós-Graduação em Biologia Celular e
Molecular da Universidade Federal do
Rio Grande do Sul para obtenção do
Grau de Mestre em Biologia Celular e
Molecular.
Prof. Dr. João A. P. Henriques
Orientador
Prof. Dr. Diego Bonatto
Orientador
Porto Alegre
Outubro - 2010
1