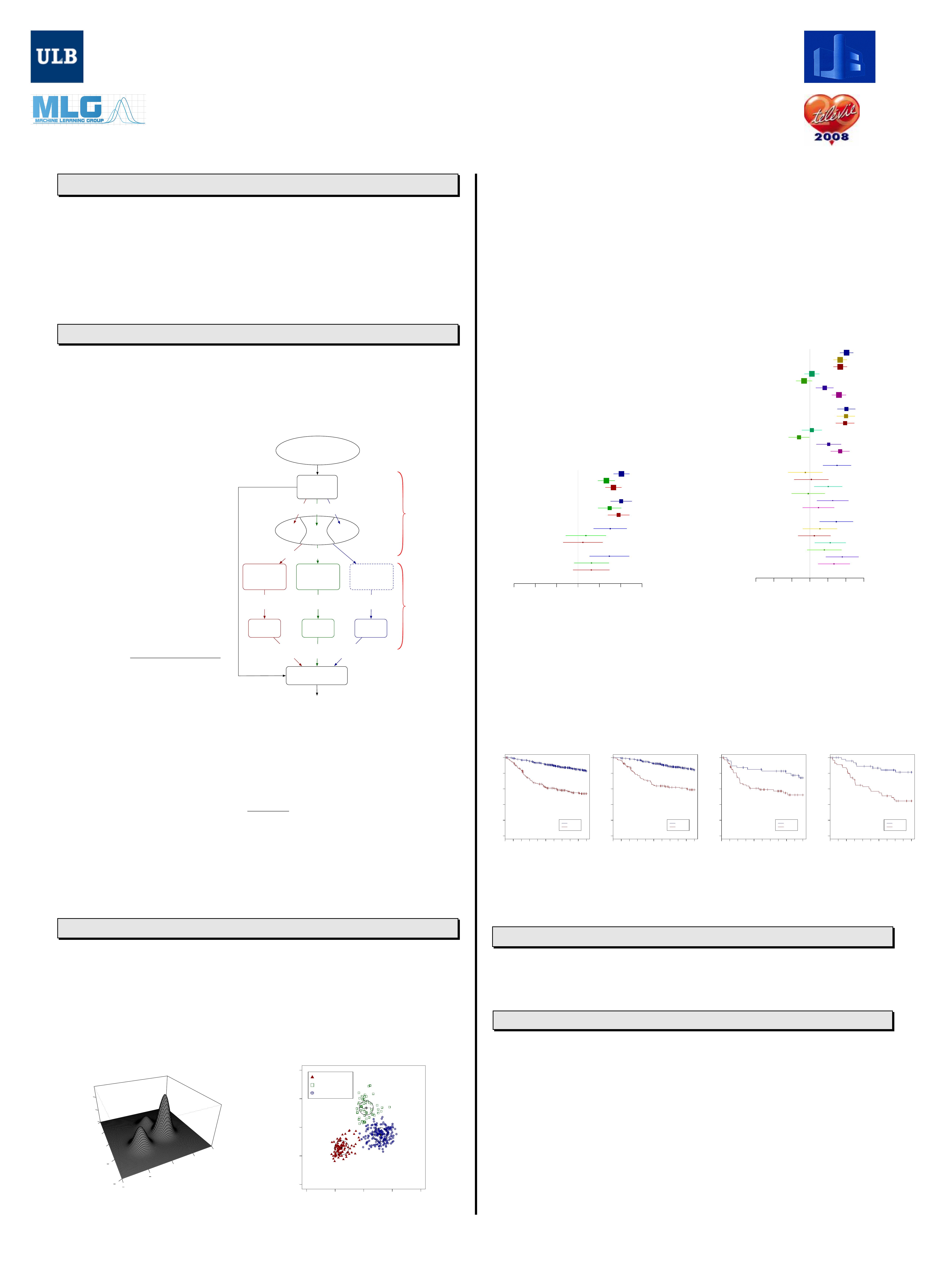
Integrating the Molecular Subtypes of Breast Cancer
into a Novel Prognostic Model
Haibe-Kains B1,2,*, Desmedt C1,*, Roth´
e F1, Piccart MJ1, Bontempi G2,#, and Sotiriou C1,#
1Functional Genomics Unit, Institut Jules Bordet, Brussels, Belgium
2Machine Learning Group, Universit´
e Libre de Bruxelles, Brussels, Belgium
*Co-first authors, #Co-last authors.
1. Background
THE early gene expression studies in breast cancer have provided a molecular classification of
these tumors into at least three clinically relevant subtypes (ER-/HER2-, HER2+ or ER+/HER2).
Our group recently introduced a robust method for subtype identification, exhibiting numerous advan-
tages compared to the hierarchical clustering used in these initial publications [7, 1].
From a prognostic point of view, several signatures have been identified in the global population of
patients. However, the majority of these only yielded good prognostic performance in the ER+/HER2-
subtype [7, 1]. Additionally, we showed that clinical and genomic prognostic factors dramatically
depend on the molecular subtype of breast tumors.
In this work, we propose a novel prognostic model which takes into the account the molecular het-
erogeneity of breast cancer.
2. Methods
WEdeveloped a two-step prognostic model called GENIUS (Gene Expression progNostic Index
Using Subtypes) as depicted in Figure 1:
1. Accurate assessment of the probabilities for a tumor to belong to each of the three breast cancer
molecular subtypes (subtype clustering).
2. Combination of these probabilities with subtype-specific prognostic signatures (subtype prognostic
gene signatures), which results in the final GENIUS risk predictions.
Subtype Clustering
Model-based clustering (mixture of Gaus-
sians) in a two-dimensional space defined
by the ESR1 and ERBB2 module scores
representing the ER and HER2 pheno-
types respectively [1]. Once fitted to the
training set, this clustering model returns
a set of probabilities of a tumor belonging
to each subtype, denoted by ρjwhere j∈
{1=ER-/HER2-,2=HER2+,3=ER+/HER2-}
are the subtypes.
Subtype Prognostic Gene Signatures
Stability-based feature ranking method [2]
using a weighted version of the concor-
dance index [3] as scoring function:
Cwted(xi, y, ρj) = Pk,l∈Ωwkl1{xki > xli}
Pk,l∈Ωwkl
where xiare the expressions of gene
i,yare the survival data and wkl =
ρj(xk)ρj(xl)is the weight for the pair of
comparable patients {k, l}with ρj(x)be-
ing the probability for a patient’s tumor x
to belong to the subtype j.
Subtypes
identification
Identification
of prognostic
genes
subtype
signature
subtype
signature
subtype
signature
Model
building
Model
building
Model
building
subtype
risk score
subtype
risk score
subtype
risk score
Combination
{ρ1, ρ2, ρ3}
ρ1ρ2
Identification
of prognostic
genes
AURKA
risk predictions
ER-/HER2- HER2+ ER+/HER2-
ρ1ρ2ρ3Subtype
clustering
Subtype prognostic
gene signatures
Training set
Figure 1: Design of GENIUS prognostic model.
The subtype prognostic gene signatures were therefore used to build the subtype risk prediction mod-
els such that, for a subtype j, the subtype risk score, denoted by rj, was defined as the weighted
combination of all the gene expressions in the corresponding signature:
rj=Pi∈Qwixi
nQ
where Qis the set of genes in the prognostic signature specific to subtype j,nQthe number of genes
in Qand wiis either +1 or −1depending on the concordance index of gene i.
The final risk predictions were computed by combining the three subtype risk scores (Figure 1) in a
local Model Network [4]:
r=X
j
ρjrj
3. Results
We focussed our analysis on untreated node-negative patients in order to build a prognostic model
for early stage breast cancer and to avoid any confounding factors due to the treatment effects on
survival (untreated). We used VDX [6, 5] as training set since this population contained the largest
set of untreated patients with early breast cancer.
Subtype Clustering
Once subtype clustering was fitted on the training set (see Figures 2, we were able to robustly iden-
tify three subtypes: ER-/HER2- (99), HER2+ (54) and ER+/HER2- (191) (Figure 3). We validated the
robustness of this clustering model in 21 breast cancer microarray datasets (data not shown).
ESR1
2
1
0
1
2
\end{minipage}
ERBB2
2
1
0
1
2
Density
0.0
0.5
1.0
Figure 2: The subtype clustering model is com-
posed of a mixture of three Gaussians.
−2 −1 01 2
−2 −1 01 2
ESR1
ERBB2
Figure 3: Identification of breast cancer molecu-
lar subtypes in the training set (VDX).
Subtype Prognostic Gene Signatures
Since we showed in [7, 1] that most of current gene signatures are only prognostic in the ER+/HER2-
subtype and that proliferation-related genes are their driving force, we did not generate a new prog-
nostic signature for this subtype but considered instead the proliferation module (AURKA) introduced
in [1], as subtype signature.
In contrast, very few prognostic signatures have been reported thus far in the ER-/HER2- and HER2+
subtypes. Therefore, we identified two stable signatures composed of 63 and 22 genes for the
ER-/HER2- and HER2+ subtypes respectively.
Using these subtype prognostic gene signatures, we computed the GENIUS risk predictions for a set
of 745 untreated node-negative patients retrieved from 5 public datasets.
Performance Assessment and Comparison
As sketched in Figure 5, GENIUS risk score pre-
dictions significntly outperformed current gene sig-
natures in the global population of patients (C-
index of 0.71, superiority test p-values <0.05) and
was very competitive in each subtype (C-indices of
0.7, 0.66 and 0.66 in the ER+/HER2-, ER-/HER2-
and HER2+ subtypes, respectively). GENIUS also
outperformed clinical guidelines (superiority test p-
values <0.05) in most cases (Figure 4).
ALL:
ER+/HER2!:
ER!/HER2!:
HER2+:
GENIUS
AOL
NPI
GENIUS
AOL
NPI
GENIUS
AOL
NPI
GENIUS
AOL
NPI
0.2 0.3 0.4 0.5 0.6 0.7 0.8
concordance index
Test for GENIUS
superiority
0.0013
0.02
0.043
0.19
0.037
0.03
0.082
0.077
Figure 4: Performance of risk score predictions
for GENIUS and prognostic clinical models wrt the
molecular subtype.
ALL:
ER+/HER2!:
ER!/HER2!:
HER2+:
GENIUS
AURKA
GGI
STAT1
PLAU
IRMODULE
SDPP
GENIUS
AURKA
GGI
STAT1
PLAU
IRMODULE
SDPP
GENIUS
AURKA
GGI
STAT1
PLAU
IRMODULE
SDPP
GENIUS
AURKA
GGI
STAT1
PLAU
IRMODULE
SDPP
0.2 0.3 0.4 0.5 0.6 0.7 0.8
concordance index
Test for GENIUS
superiority
0.018
5E-12
1E-4
0.015
9E-14
0.012
0.27
2E-6
0.065
0.094
5E-9
0.25
0.016
0.1
0.44
0.028
0.0037
0.0051
0.039
0.15
0.62
0.39
0.10
0.25
Figure 5: Performance of risk score predictions
for GENIUS and current prognostic gene signa-
tures wrt the molecular subtype.
The performance of the risk group predictions computed by GENIUS is illustrated in Figure 6.
We observed a significant difference between the survival curves of low- and high-risk groups for
both the global population (HR: 3.7; 95%CI [2.7,5]; p=1E-16) and all the subtypes: HRs of 3.7
(95%CI [2.5,5.5]; p=1E-10), 2.7 (95%CI [1.3,5.6]; p=7E-3) and 3.9 (95%CI [1.8,8.8]; p=8E-4) in the
ER+/HER2-, ER-/HER2- and HER2+ subtypes, respectively. The probability of distant metastasis or
relapse free survival of the low-risk group at 5 years was estimated at 91% in the global population,
and 92%, 83% and 89% in the ER+/HER2-, ER-/HER2- and HER2+ subtypes, respectively.
0.0 0.2 0.4 0.6 0.8 1.0
Probability of survival
Low
High
HR=2.6, 95%CI [1.3,5.5], p!value=9.2E!03
012345678910
Time (years)
No. At Risk
Low 48 46 42 40 39 37 37 36 31 27 21
High 68 63 50 44 40 35 32 29 23 20 16
0.0 0.2 0.4 0.6 0.8 1.0
Probability of survival
Low
High
HR=4.1, 95%CI [1.8,9.2], p!value=7.7E!04
012345678910
Time (years)
No. At Risk
Low 50 47 45 43 41 39 35 32 31 28 26
High 55 51 47 39 33 31 30 27 22 20 18
0.0 0.2 0.4 0.6 0.8 1.0
Probability of survival
Low
High
HR=3.9, 95%CI [2.6,5.8], p!value=6.6E!11
012345678910
Time (years)
No. At Risk
Low 374 370 363 354 338 323 282 247 213 191 166
High 129 125 111 97 88 79 75 66 64 62 51
0.0 0.2 0.4 0.6 0.8 1.0
Probability of survival
Low
High
HR=3.6, 95%CI [2.7,4.9], p!value=2.9E!16
012345678910
Time (years)
No. At Risk
Low 472 461 448 435 416 397 352 314 273 244 213
High 252 237 206 178 159 143 135 120 107 100 85
ALL ER+/HER2- ER-/HER2- HER2+
Figure 6: Survival curves of GENIUS risk group predictions wrt the molecular subtype.
4. Conclusions
THIS novel prognostic model, which considers the molecular heterogeneity of breast cancer, out-
performs the current clinical models and gene signatures. GENIUS was the only signature to be
highly prognostic in all the molecular subtypes. Additionally, the modular architecture of the model
allows plugging in any other gene expression signatures, potentially enlarging its usability.
References
[1] C. Desmedt, B. Haibe-Kains, P. Wirapati, et al. Biological Processes Associated with Breast Cancer Clinical Outcome
Depend on the Molecular Subtypes. Clin Cancer Res, 14(16):5158–5165, 2008. doi:10.1158/1078-0432.CCR-07-
4756.
[2] B. Haibe-Kains. Identification and Assessment of Dene Signatures in Human Breast Cancer. Ph.D. thesis, Universit´
e
Libre de Bruxelles, 2009.
[3] F. J. Harrell, K. Lee, and D. Mark. Multivariable prognostic models: issues in developing models, evaluating assump-
tions and adequacy, and measuring and reducing errors. Stat Med, 15(4):361–387, 1996. doi:10.1002/(SICI)1097-
0258(19960229)15:4¡361::AID-SIM168¿3.0.CO;2-4.
[4] T. A. Johansen and B. A. Foss. Constructing NARMAX models using ARMAX models. International Journal of Control,
58:1125–1153, 1993.
[5] A. J. Minn, G. P. Gupta, D. Padua, et al. Lung metastasis genes couple breast tumor size and metastatic spread.
Proceedings of the National Academy of Sciences, 104(16):6740–6745, 2007. doi:10.1073/pnas.0701138104.
[6] Y. Wang, J. G. Klijn, Y. Zhang, et al. Gene-expression profiles to predict distant metastasis of lymph-node-negative
primary breast cancer. Lancet, 365:671–679, 2005.
[7] P. Wirapati, C. Sotiriou, S. Kunkel, et al. Meta-analysis of gene expression profiles in breast cancer: toward a unified
understanding of breast cancer subtyping and prognosis signatures. Breast Cancer Research, 10(4):R65, 2008. ISSN
1465-5411. doi:10.1186/bcr2124.
IMPAKT (ESMO) Breast 2009