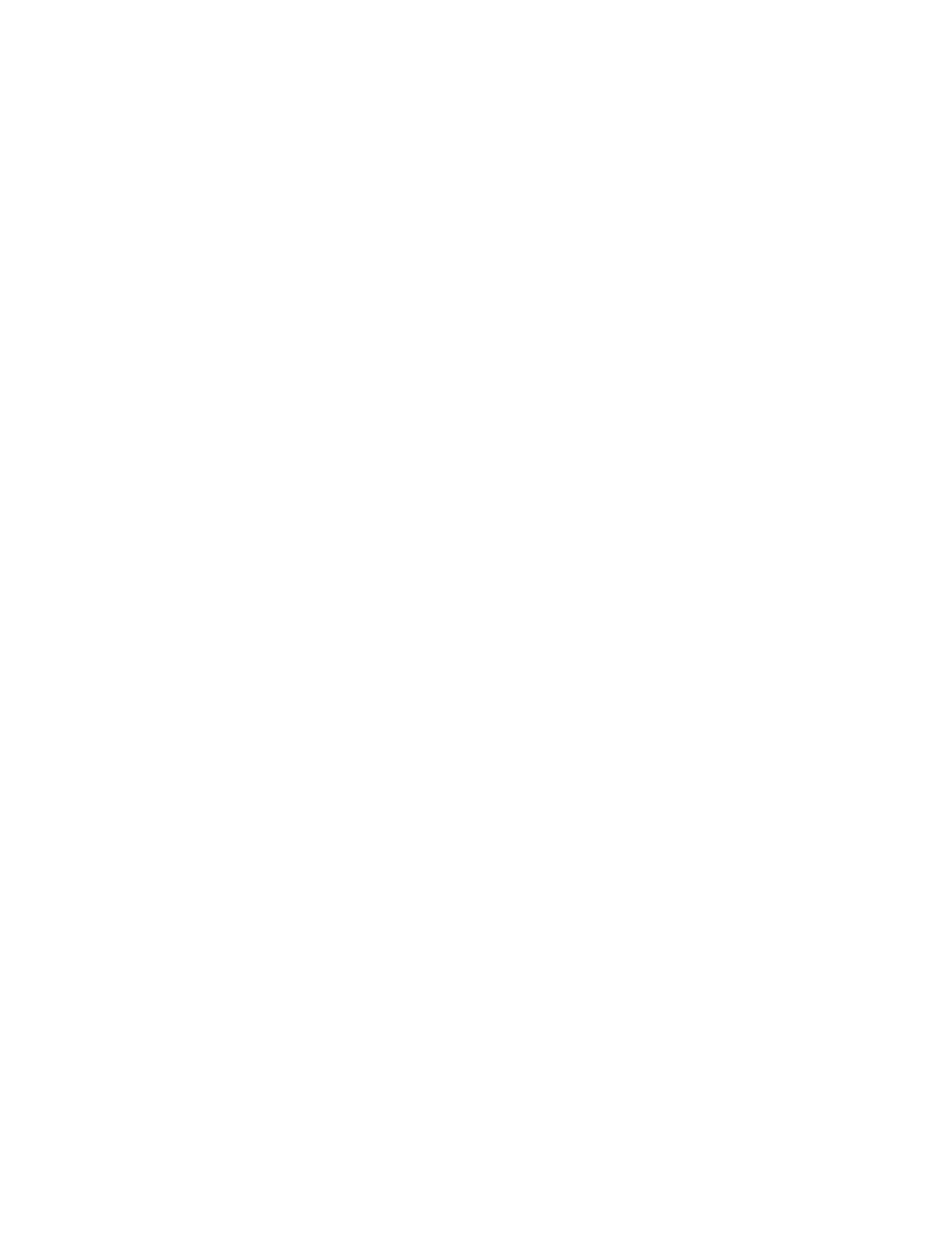
MICROARRAYS, EMPIRICAL BAYES AND THE TWO-GROUPS MODEL 3
der a classical null hypothesis,
theoretical null : zi∼N(0,1).(1.1)
Here is a brief description of the four examples, with
further information following as needed in the sequel.
EXAMPLE A [Prostate data, Singh et al. (2002)].
N=6033 genes on 102 microarrays, n1=50 healthy
males compared with n2=52 prostate cancer patients;
zi’s based on two-sample tstatistics comparing the two
categories.
EXAMPLE B [Education data, Rogosa (2003)]. N=
3748 California high schools; zi’s based on binomial
test of proportion advantaged versus proportion dis-
advantaged students passing mathematics competency
tests.
EXAMPLE C [Proteomics data, Turnbull (2006)].
N=230 ordered peaks in time-of-flight spectroscopy
study of 551 heart disease patients. Each peak’s z-value
was obtained from a Cox regression of the patients’
survival times, with the predictor variable being the
551 observed intensities at that peak.
EXAMPLE D [Imaging data, Schwartzman et al.
(2005]). N=15,445 voxels in a diffusion tensor
imaging (DTI) study comparing 6 dyslexic with six
normal children; zi’s based on two-sample tstatistics
comparing the two groups. The figure shows only a
single horizontal brain section having 655 voxels, with
“−” indicating zi<0, “+”forzi≥0, and solid circles
for zi>2.
Our four examples are enough alike to be usefully
analyzed by the two-groups model of Section 2, but
there are some striking differences, too: the theoretical
N(0,1)null (1.1) is obviously inappropriate for the ed-
ucation data of panel B; there is a hint of correlation of
z-value with peak number in panel C, especially near
the right limit; and there is substantial spatial correla-
tion appearing in the imaging data of panel D.
My plan here is to discuss a range of inference prob-
lems raised by large-scale hypothesis testing, many of
which, it seems to me, have been more or less under-
emphasized in a literature focused on controlling Type-
I errors: the choice of a null hypothesis, limitations of
permutation methods, the meaning of “null” and “non-
null” in large-scale settings, questions of power, test
of significance for groups of cases (e.g., pathways in
microarray studies), the effects of correlation, multiple
confidence statements and Bayesian competitors to the
two-groups model. The presentation is intended to be
as nontechnical as possible, many of the topics being
discussed more carefully in Efron (2004, 2005, 2006).
References will be provided as we go along, but this is
not intended as a comprehensive review. Microarrays
have stimulated a burst of creativity from the statistics
community, and I apologize in advance for this arti-
cle’s concentration on my own point of view, which
aims at minimizing the amount of statistical model-
ing required of the statistician. More model-intensive
techniques, including fully Bayesian approaches, as in
Parmigiani et al. (2002) or Lewin et al. (2006), have
their own virtues, which I hope will emerge in the Dis-
cussion.
Section 2discusses the two-groups model and false
discovery rates in an idealized Bayesian setting. Empir-
ical Bayes methods are needed to carry out these ideas
in practice, as discussed in Section 3. This discussion
assumes a “good” situation, like that of Example A,
where the theoretical null (1.1) fits the data. When it
does not, as in Example B,theempirical null methods
of Section 4come into play. These raise interpretive
questions of their own, as mentioned above, discussed
in the later sections.
We are living through a scientific revolution pow-
ered by the new generation of high-throughput obser-
vational devices. This is a wonderful opportunity for
statisticians, to redemonstrate our value to the scien-
tific world, but also to rethink basic topics in statistical
theory. Hypothesis testing is the topic here, a subject
that needs a fresh look in contexts like those of Fig-
ure 1.
2. THE TWO-GROUPS MODEL AND FALSE
DISCOVERY RATES
The two-groups model is too simple to have a single
identifiable author, but it plays an important role in the
Bayesian microarray literature, as in Lee et al. (2000),
Newton et al. (2001) and Efron et al. (2001). We sup-
pose that the Ncases (“genes” as they will be called
now in deference to microarray studies, though they
are not genes in the last three examples of Figure 1)
are each either null or nonnull with prior probability
p0or p1=1−p0, and with z-values having density
either f0(z) or f1(z),
p0=Pr{null}f0(z) density if null,
(2.1)
p1=Pr{nonnull}f1(z) density if nonnull.
The usual purpose of large-scale simultaneous test-
ing is to reduce a vast set of possibilities to a much
smaller set of scientifically interesting prospects. In