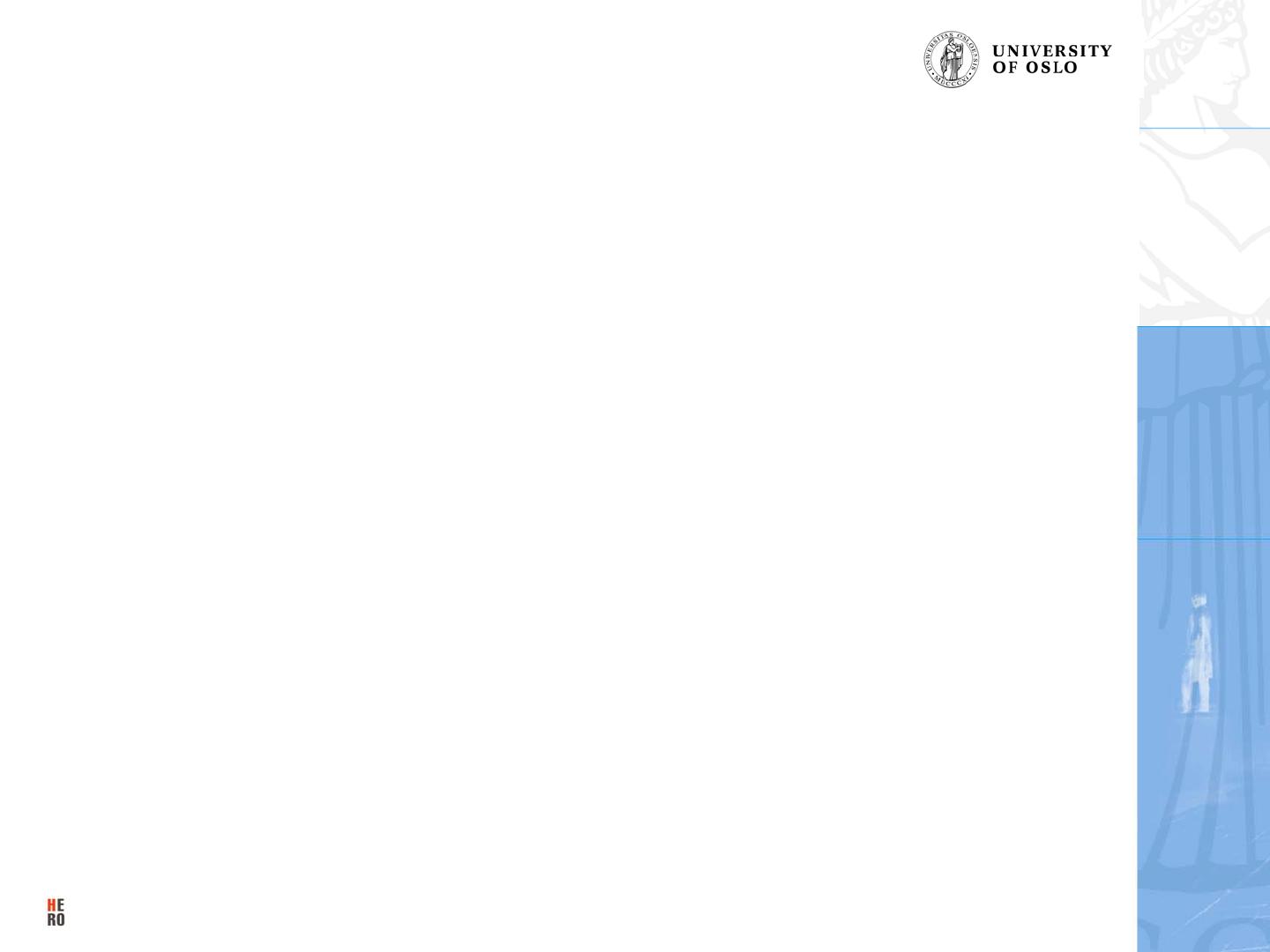
Health Economics Research Programme at the University of Oslo - HERO
Background
• In national health systems, like in the Nordic countries
and in Great Britain, there are no individual contracts
• Priority assignment of patients is determined by the
parliament
• The Act on Patient Rights:
– Severity of the disease
– Expected health gain
– Health effect relative to cost of treatment
• The Act on Regional Health Authorities
– Provide high quality specialist health care on equitable basis to
patients in need, irrespective of age, sex, place of residence,
material resources and ethnic background
• Potential conflicting goals