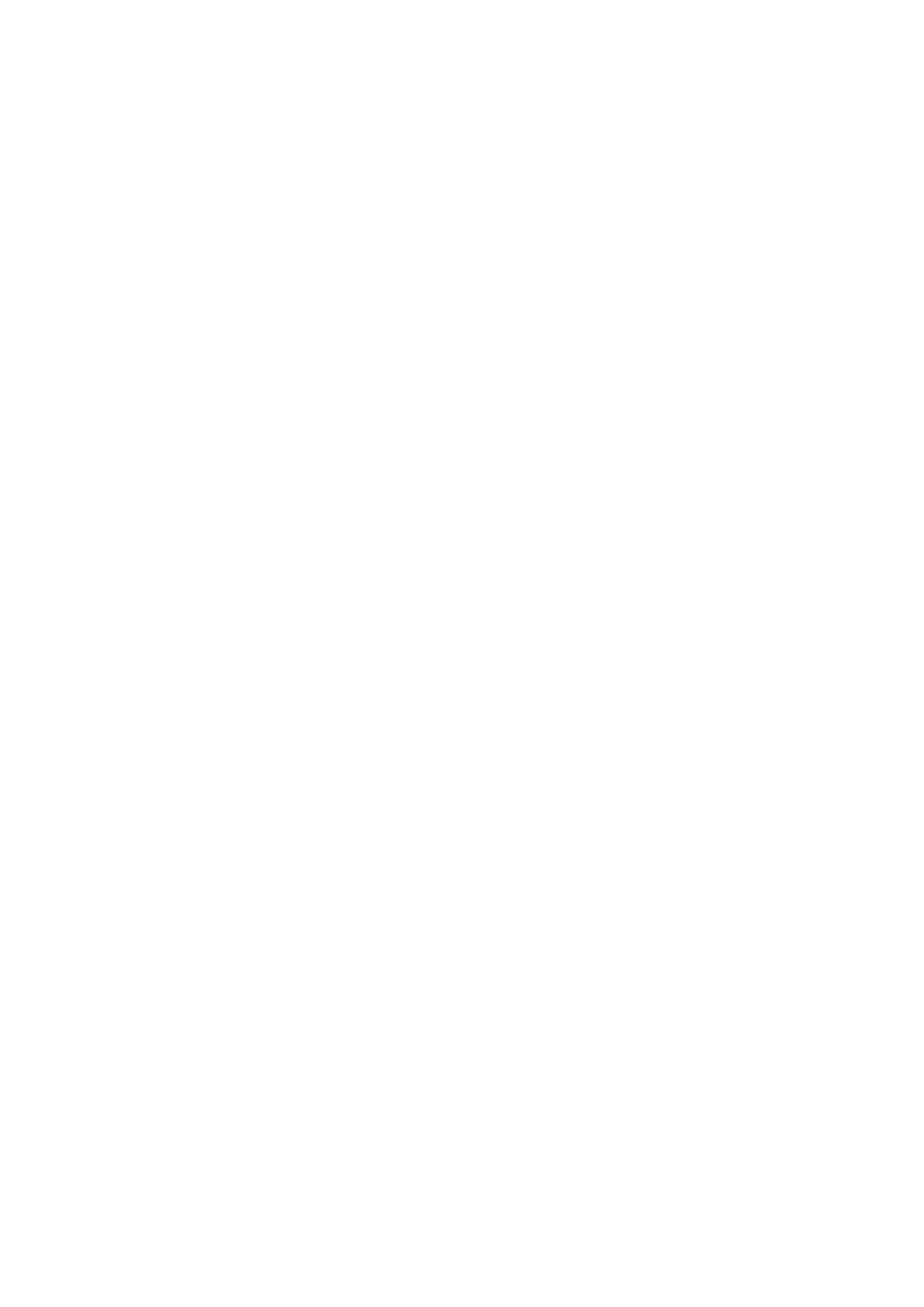
Remerciements
Je tiens à adresser en premier lieu mes remerciements à mon
directeur de thèse, Monsieur le Docteur Pierre-Alain Jayet, de m’avoir
fait confiance et laissé une large autonomie. J’ai pu apprécier non
seulement sa dimension scientifique, mais aussi sa dimension humaine.
J’en profite pour lui exprimer ici ma plus profonde gratitude.
Je tiens à remercier les membres du jury qui me font l’honneur
d’avoir consacré leur temps précieux à examiner mon travail.
Je souhaite également adresser mes remerciements à Monsieur
le Docteur Stephan Marette et Monsieur le Docteur Jean Christophe
Bureau, directeurs de l’Unité UMR 210 INRA Economie Publique,
pour m’avoir accueillie dans son laboratoire.
Mes sincères remerciements s’adressent à toute l’équipe de
l’INRA - UMR Economie Publique, Grignon, votre chaleur et votre
générosité m’ont aidé bien plus que vous ne l’imaginez. J’adresse
mes vifs remerciements à Sylvie La Mantia, Celine Lecoq, Delphine
Leconte-Demarsy, Elvire Petel, Athanasios Petsakos, Pierre Humblot,
Anne Fournier, Anna Lungarska et Elisabeth Maltese qui ont largement
enrichi ces années de thèse.
iii