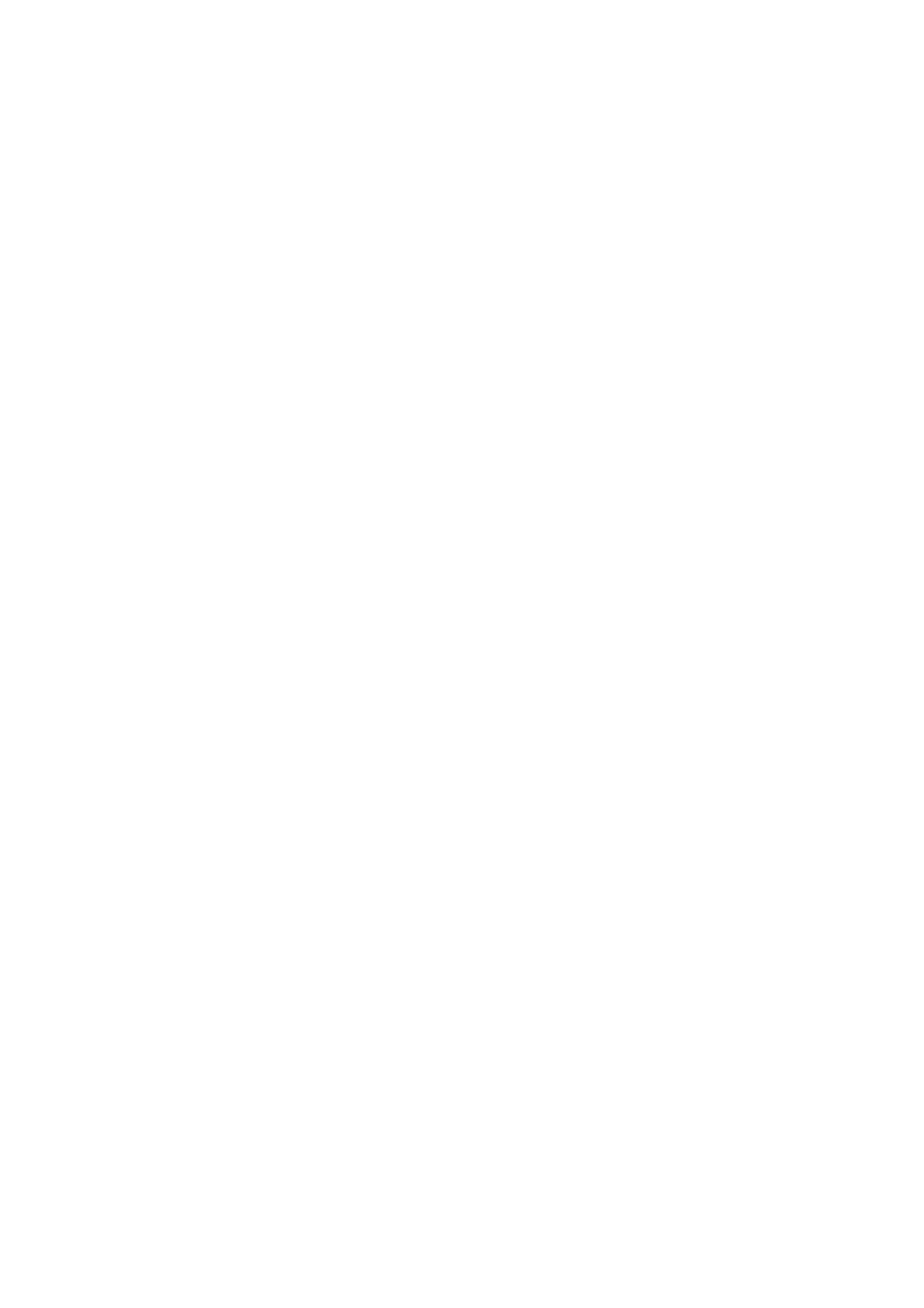
Résumé
Ce travail de thèse a porté sur différents aspects de la prédiction des éruptions, à
différentes échelles de temps et d’espace. Dans un premier temps, une analyse descriptive
des propriétés statistiques de la dynamique éruptive à l’échelle mondiale et l’apparition de
lois puissances à différentes échelles nous ont permis de relier la dynamique des éruptions
à celle d’un système critique auto-organisé, justifiant de la difficulté rencontrée à prédire
les éruptions de façon déterministe et de l’intérêt de recourir à des méthodes statistiques.
Dans une deuxième partie, l’étude à l’échelle mondiale des lois d’Omori retrouvées pour
la sismicité autour des éruptions, ainsi que l’analyse des propriétés de ces lois d’Omori
en fonction de la taille des éruptions, suggèrent que l’énergie d’endommagement et de
relaxation dans la croûte est distribuée en loi puissance autour des éruptions, mais avec
des coefficients de chargement et de relaxation différents de la sismicité tectonique clas-
sique, et donc dépendant des processus qui génèrent l’endommagement. En zoomant sur
trois observables du Piton de la Fournaise (sismicité, déformation et variations de vitesse
sismique), nous avons ensuite montré que les comportements respectifs des observables
imagent les différentes étapes du processus éruptif, pour différentes échelles de temps et
d’espace. L’analyse des comportements pré-éruptifs et l’utilisation de méthodes de "pat-
tern recognition" ont permis de mettre en évidence l’apport de la combinaison des infor-
mations des différents précurseurs pour l’optimisation des objectifs de prédiction. Enfin,
l’analyse plus spécifique et déterministe des mécanismes source de la sismicité du Piton
de la Fournaise, à partir d’une méthode développée pour le contexte particulier d’une
3