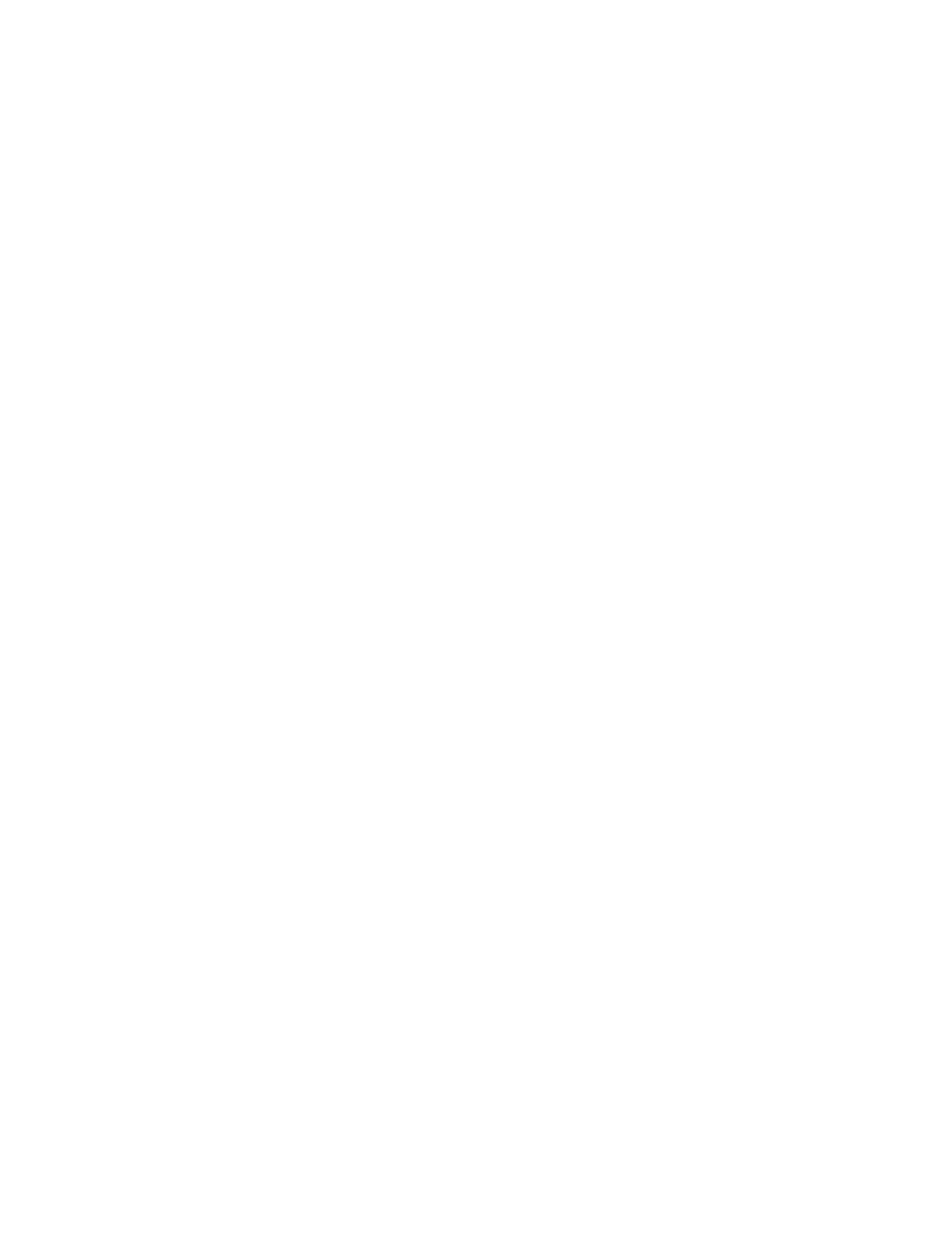
EDITORIALS
World Psychiatry 20:2 - June 2021 151
Rediscovering the mental health of populations
e principles of prevention espoused by G. Rose1 have under-
pinned many modern successes in health care. In areas such as
cardiometabolic diseases, injuries and violence, and substance
abuse, shifting the community distribution of risk factors has
become the primary strategy. e ensuing reductions in disease
burden have been striking.
Psychiatry remains an outlier. Over decades, the quality of clini-
cal care has been improved, greater funding has been attracted,
more and better trained mental health professionals have been
grown, and the governance of mental health care has been up-
graded2. However, the emphasis in recent initiatives in high-in-
come countries has been overwhelmingly a further extension of
treatment: early clinical intervention has been the dominant ini-
tiative taken up in government investments into the mental health
of young people3.
Yet, this continuing expansion of government expenditure,
prescribing of antidepressants and availability of psychologi-
cal services has still not been accompanied by reductions in
the prevalence of common mental disorders3. While it remains
possible that this in part reects a continuing failure to scale
minimally-sucient treatments, the evidence from other elds
of medicine suggests that a more likely explanation is the lack of
scalable risk-focused prevention strategies.
is failure to embrace population-based approaches to pre-
vention in psychiatry is understandable. Most clinicians find
the endorsement of population perspectives dicult. For them,
the individual is the unit of study1. For psychiatry, the opacity
of pathophysiological processes has supported the tendency to
focus on interventions directed at the individual. Recent excite-
ment about progress in genetics and neuroscience has reinforced
this tendency, with both major research funding agencies and
the pharmaceutical industry emphasizing the individual over
the social context.
In this scenario, the paper by Fusar-Poli et al in this issue of
the journal4 raises questions around the optimal strategies for
prevention in psychiatry. e overwhelming emphasis to date
across common mental disorders, psychosis and bipolar disor-
der has been on individuals at high risk by virtue of early clini-
cal symptoms or genetic predisposition. These selective and
indicated approaches to prevention have targeted subjects at the
tail of the distribution, with an aim of reducing the likelihood of
transition to clinical caseness. However, this emphasis on indi-
viduals has been accompanied by a failure to address structural
and social determinants.
E. Durkheim’s work, well over a century ago, drew the conclu-
sion that suicide rates are stable and distinctive characteristics of
populations. He viewed suicide as a collective phenomenon in
which personal factors are less important than the social context.
Similarly, strategies focused on the social, economic and regula-
tory context that bring a reduction in average alcohol consump-
tion have been far more successful in reducing levels of alcohol
use disorders than individually targeted interventions5. This
principle that actions to reduce modest risks in a large group will
generate greater benets than targeting conspicuous risks in a
small number should guide the prevention of mental disorders.
One challenge is that most risks for mental disorders lie out-
side the direct inuence of the health sector. For young people,
social determinants of mental health derive from inequitable
gender norms, shifts in family structure and function, culture
and religion, economic development and its consequences, digi-
tal technology, urbanization and planetary change. ese social
and structural determinants shape peer, family and community
relationships, accessibility of service systems, the likelihood of ex-
periencing major external events, as well as risks related to life-
style and individual behaviour. For mental disorders, as for the
physiological processes underpinning physical health, there are
also sensitive periods in which risks are more likely to become
embedded and when prevention will be more eective.
e COVID-19 pandemic illustrates the inuence of social
and structural factors on the mental health of all age groups, but
particularly the young. It also illustrates areas where psychiatry
should be acting. e eects of lifestyle risk factors for mental
disorders, including physical inactivity, screen time, irregular
sleep and poor diets, have been enhanced. Even more profound
have been the shifts in relationships, with disruption to friendships
and peer interactions, heightened worries about and sometimes
conict with family members, connement to home and loss of
the social milieu of schools, including extracurricular activities.
In taking prevention in psychiatry forward, there are further
lessons to be drawn from other areas of medicine1. Epidemiol-
ogy remains the underpinning discipline of public health, and,
for psychiatry, epidemiology should adopt both life-course and
population perspectives. However, psychiatric epidemiology
remains in a parlous state, particularly for children and young
people. Global coverage for even basic estimates of prevalence
lies under seven percent, with rates in low- and middle-income
countries substantially lower, and 124 countries having absolute-
ly no data6. Coverage of risk factors is even weaker.
As noted by Fusar-Poli et al, a life-course perspective on men-
tal health is essential4. Yet, a life-course perspective would ideal-
ly extend across generations, given that familial clustering is the
clearest of all risk factors. Beyond genetics, there are malleable in-
tergenerational risk factors for mental disorders, ranging from the
biological (e.g., epigenetic) through to the structural (e.g., inequi-
table gender norms), including those risks that become embed-
ded prior to conception7. Longer-term perspectives derived from
prospective life-course studies have the potential to guide pre-
vention research and policy, particularly when combined with
powerful new analytic tools for causal inference.
Recent intervention trials provide grounds for optimism.
Schools will be one important context for prevention. Chil-
dren and young people spend close to half their waking hours in
school and education. Policy-makers increasingly understand
that poor student mental health aects learning and academic