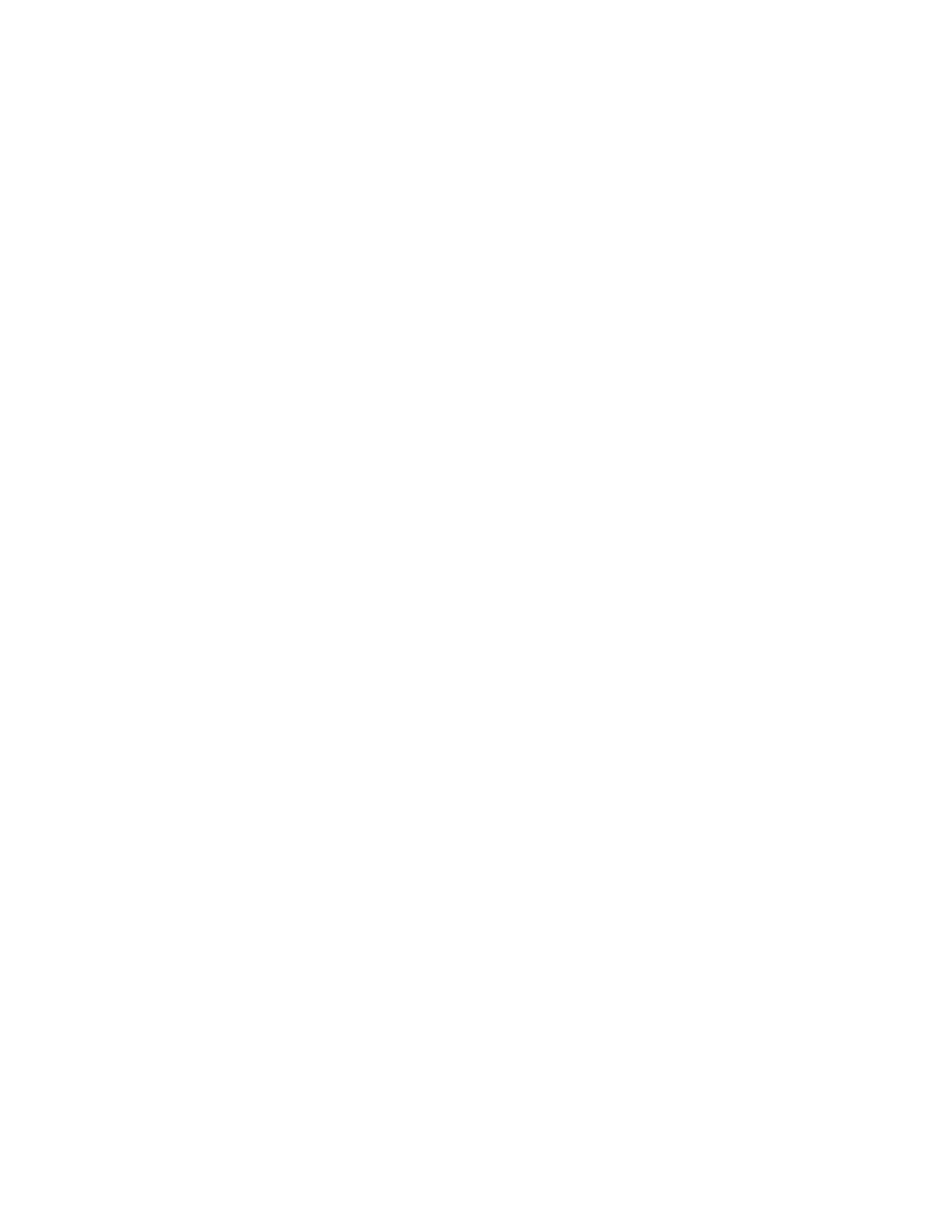
prediction. The development of high speed electronic computers has had a profound impact on
the methods of classification in biological fields. The rapidity of the computer’s operation has
made it possible for the first time to consider large numbers of characteristics in classifying
microbes. Most approaches to the problems of microbial taxonomy have arisen from either of the
two viewpoints, one derived from phylogenetic and other from practical consideration. The
former viewpoint too frequently arises from some major premise, which has little practical
connotation. The latter view point often leads to the submergence of large groups of organisms
not known to be of economic importance, because of an attitude of impatience towards any
system which does not reflect the methods used in the specialized laboratory where steps in the
identification of an unknown organisms must be measured in terms of utility and speed. It must,
therefore, be realized that the precise delineation of species cannot be the primary aim of the
microbial taxonomy at present. It is seldom possible and often it may not even be desirable to
compromise by recognizing the necessity for the organization within a taxonomic system of a
selected body of knowledge of important differential characters which may be applied when
practical consideration that demands that phylogenetically related organisms be distinguished
one from the other. This implies that taxonomic systems must undergo periodic revision with the
advent of new knowledge.
Classification means the act of arranging a number of objects (of any sort) into groups (or
taxa) in relation to attributes possessed by those objects. The word classification is also applied
to the result of any such arrangement. Taxonomy is concerned, inter alia, with definition of the
aims of classification, the design of rules by which arrangements may be achieved, and with the
evaluation of the end results. In biological classifications, the primary objects (microorganisms,
plants, animals) are usually arranged in groups which are themselves members of larger groups
(and so on) in such a way that any item or any group appears as a member of only one larger
grouping, i.e., the groups are non-overlapping. This method of classification is the familiar
hierarchical system which can be conveniently represented by a 'family tree' or dendrogram.
The units at each level (taxonomic rank) of a hierarchical system are given distinctive names or
label a branch of taxonomy known as nomenclature. In biology, the system of nomenclature is
normally used for living organisms, which is derived from that used by the great eighteenth-
century taxonomist Linnaeus (Carl von Linne). In this system, the basic unit (the species) is
given two names one denoting its membership of a taxon at the rank that we label genus (generic
name) followed by a second denoting the particular species (specific name). These names are
written in a latinized form and constitute a so called latinized binomial (e.g., Aspergillus niger,
Bacillus subtilis, Clostridium tetani). Taxa of higher rank (families, orders, etc.) are given single
latinized names with characteristic endings (e.g., Pseudomonadaceae, family; Pseudomonadales,
order). The naming of newly discovered organisms or of newly proposed taxa of higher ranks is
governed by rigid rules standardized by international agreement. It is perhaps worth emphasizing
that it is by no means the only possible one. The simplest system would be merely to label the
different types of organism with some sort of catalogue number which referred to a listed
description. A much more useful approach might be similar to one proposed for the naming of
viruses, viz., the virus is given a group name (probably latinized) which is followed by a
descriptive formula akin to that used by botanists in floral diagrams or to the antigenic formula
of a Salmonella species. The naming of the units defined and delineated by the classification.
This latter method is, in fact, reminiscent of that often used by Linnaeus, who sometimes
followed his latinized generic name with up to a dozen descriptive 'specific' epithets. Ideally, the
coining of new names is contrived to convey as much information as possible about the organism