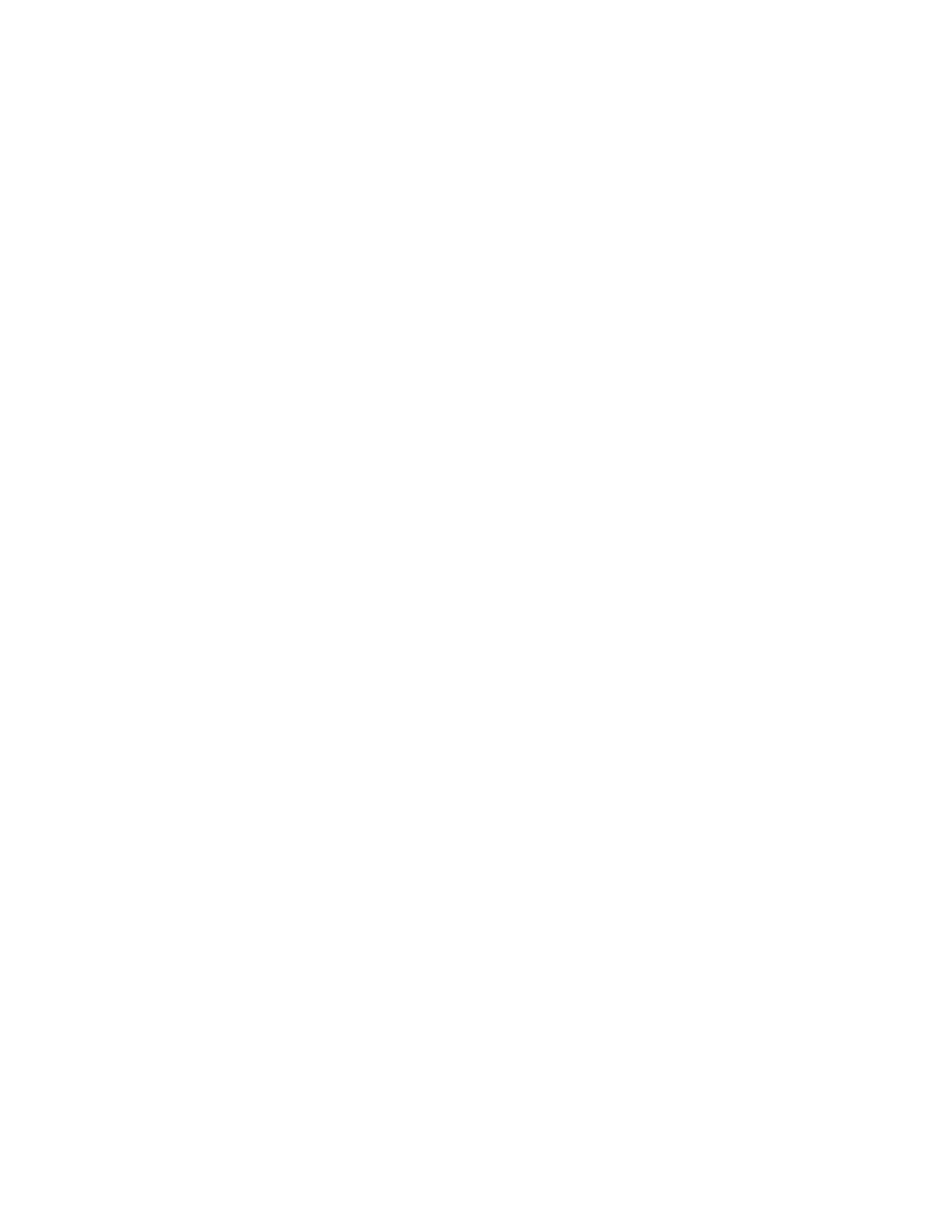
4
Introduction
Breast cancer is a very heterogeneous disease regarding the underlying
molecular alterations, the cellular composition of tumors, and the different
clinical outcomes (1), which hampers the design of effective treatment strategies
(2). To account for this intrinsic diversity, drug discovery efforts have shifted
towards mechanism-based and target-oriented strategies, particularly aiming at
modulating specific molecular pathways, patient-specific genetic alterations and
the tumor microenvironment (3,4).
Despite the expanding repertoire of new anti-cancer agents, treatment failure
remains a major challenge in the management of most advanced solid cancers,
including breast cancer (5,6). Multiple compensatory mechanisms are known to
counterbalance therapeutic effects, eventually leading to treatment failure (6).
One of the most promising strategies for better clinical outcomes is the use of
combinatorial therapy to target the distinct adaptive response mechanisms (7,8),
which may also help to overcome toxicity associated with higher doses of single
drugs. In addition, synergistic drug combinations are often more specific and
therefore improve the therapeutically relevant selectivity (9). However, although
becoming the standard care in (breast) cancer treatment, most approved drug