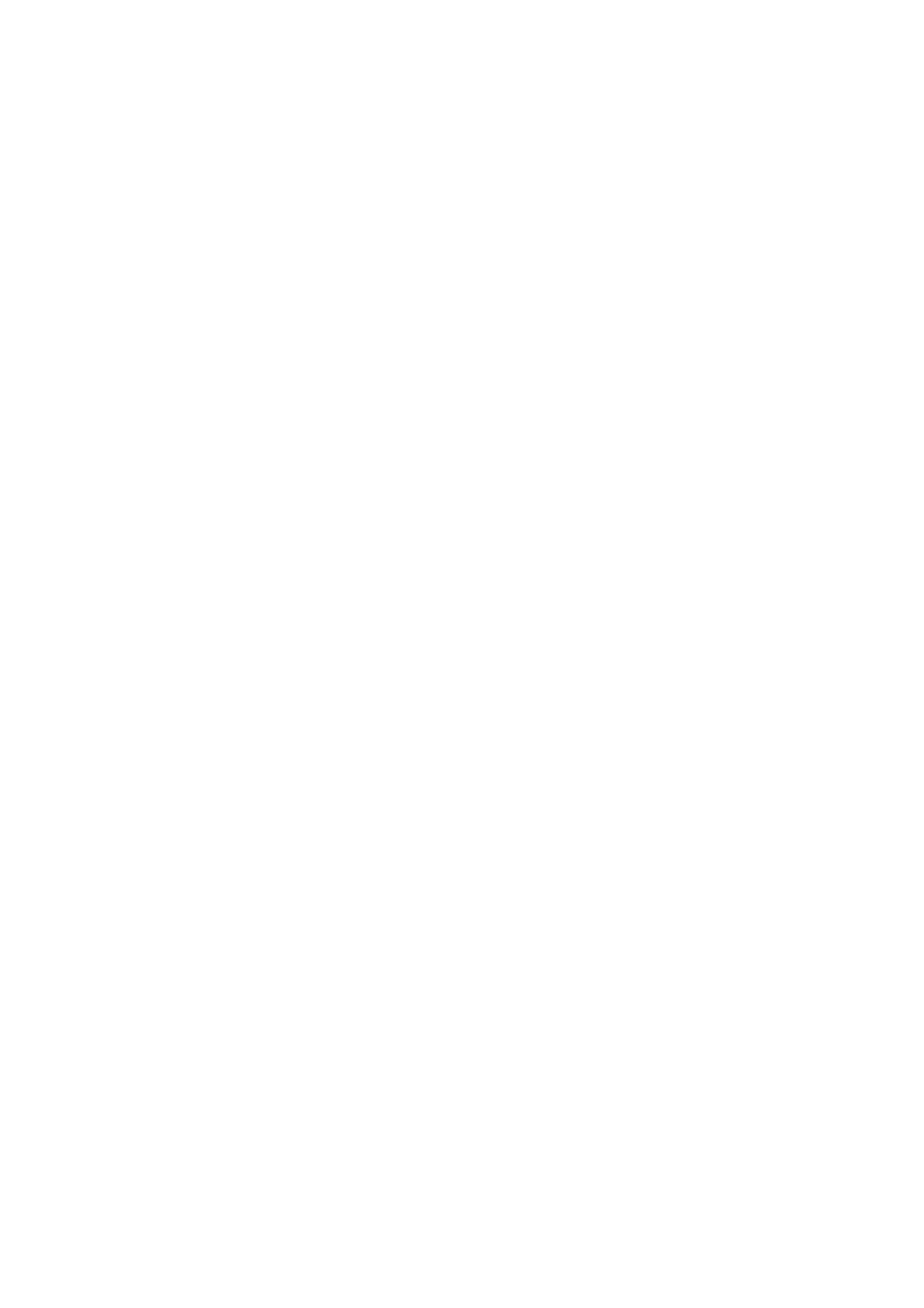
DEPARTMENT OF BIOCHEMISTRY AND MOLECULAR BIOLOGY
UNIVERSITAT AUTÒNOMA DE BARCELONA
DOCTORAL THESIS, 2013
Clinical Value of Massive Parallel HIV-1 Sequencing
in Antiretroviral Treatment-Experienced Subjects
CHRISTIAN POU GONZÀLEZ
irsiCaixa Institute for AIDS Research
Molecular Epidemiology Group
Thesis to obtain the PhD degree in Biochemistry, Molecular Biology and
Biomedicine of the Universitat Autònoma de Barcelona
Director: Roger Paredes i Deiros, MD, PhD
IrsiCaixa Institute for AIDS Research
Senior Investigator of Molecular Epidemiology Group
Tutor: Jaume Farrés Vicén, PhD
Catedràtic d'Universitat
Departament de Bioquímica i Biologia Molecular UAB