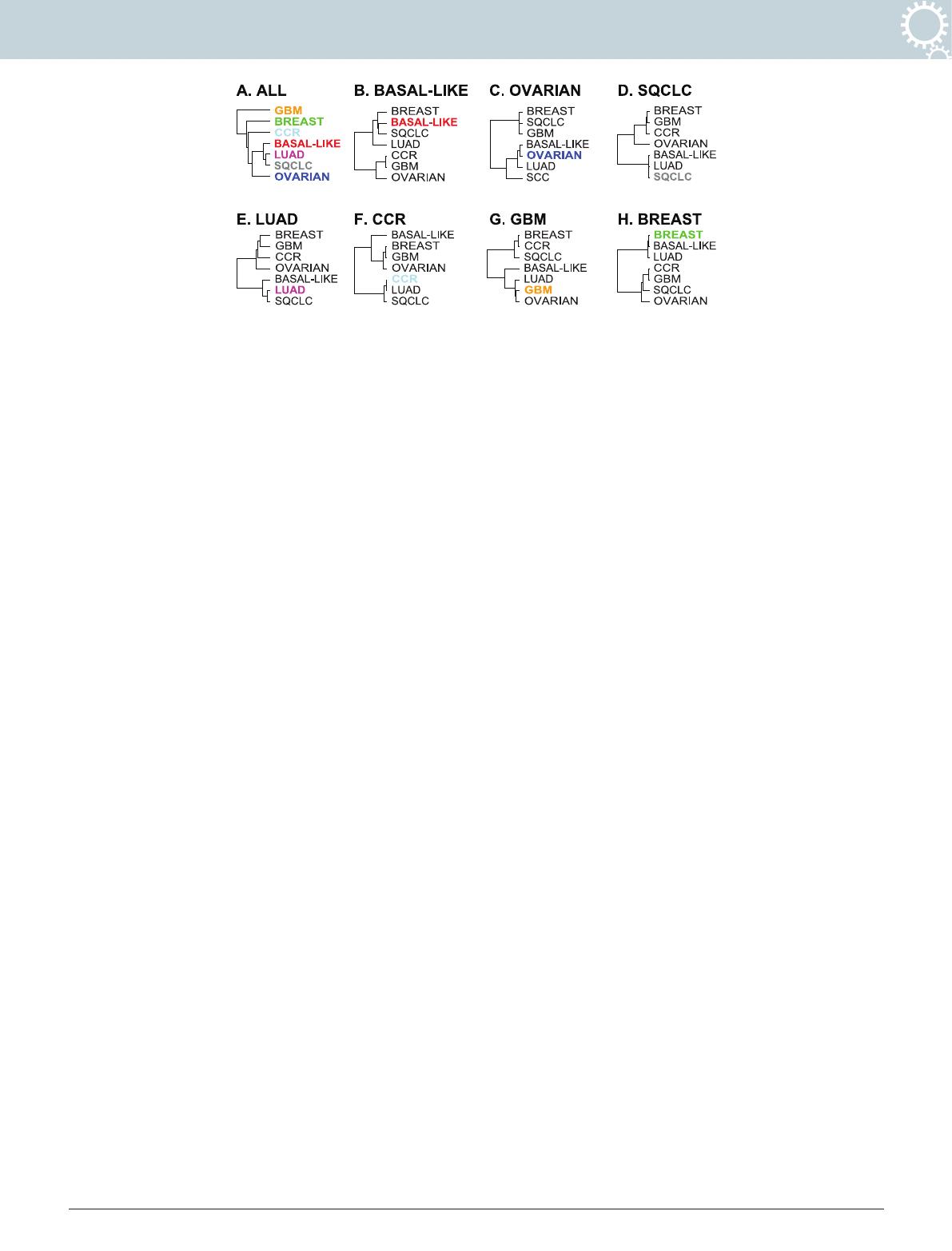
breast cancer (Fig. 3B). Concordant with this, 55% of Basal-like
breast tumors were found more similar (i.e. lower distances) to
SQCLCs than to non-Basal-like breast cancers. When compared to
the different intrinsic subtypes of breast cancer, 76%, 72% and 17% of
Basal-like breast tumors were found more similar to SQCLC than to
Luminal A, Luminal B and HER2-enriched breast tumors, respect-
ively. Interestingly, Basal-like breast tumors were found more similar
to both lung cancer types and to non-Basal-like breast cancers than to
OVARIAN tumors (Fig. 3B).
To determine the biological processes in common between Basal-
like breast cancers and SQCLC, we identified genes whose expression
is found significantly expressed in both cancer types compared to
luminal cancers (Luminal A and B tumors combined). Among the
top 300 up-regulated genes (False Discover Rate 50%) in Basal-like
breast cancer and SQCLC, we identified genes involved in ectoder-
mal differentiation (e.g. keratin 5, 14 and 17), inflammatory response
(i.e. chemokine [C-X-C motif] ligand 1 [CXCL1] and CXCL3) and
cell cycle (e.g. cyclin E1 [CCNE1] and centromere protein A
[CENPA]). Among the top 300 down-regulated genes, we identified
genes involved in the response to hormone stimulus (e.g. estrogen
receptor [ESR1] and GATA3), mammary gland development (e.g.
prolactin receptor [PRLR] and ERBB4) and microtubule-based pro-
cess (e.g. kinesin family member 12 [KIF12] and microtubule-assoc-
iated protein tau [MAPT]). This data is concordant with the
histological appearance and the immunohistochemical expression
of ER, keratins 5/6 and the proliferation-related biomarker Ki67 in
a Basal-like breast tumor, a SQCLC with a Basal-like profile and a
breast Luminal A tumor (Fig. 4).
Multiclass tumor prediction.To identify genes that are distinctive of
each cancer type, including Basal-like breast cancer, we performed
ClaNC, a nearest centroid-based classifier that balances the number
of genes per class (Fig. 5A). A 126-gene signature (18 genes per cancer
type) was established from the smallest gene set with the lowest cross
validation and prediction error (2.0%) (Fig. 5B). Among the various
cancer types, Basal-like breast cancers and SQCLCs showed the
highest prediction error (7.1% and 15.6%), and the majority of
misclassified SQCLCs (n 55, 71.4%) were identified as Basal-like
breast cancer. Of note, two previously identified diagnostic biomar-
kers of serous ovarian cancer (Wilm’s tumor [WT]-1)
18
and lung
adenocarcinoma (thyroid nuclear factor 1 [TITF-1])
19
were found
in the 18-gene list of these two cancer types (Fig. 5C).
Common patterns of gene expression across cancer types.Although
each cancer type is molecularly distinct, we sought to identify groups
of genes (i.e. gene signatures) with independent patterns of variation.
To accomplish this, we clustered all samples with the 3,486 most
variable genes (Fig. 6) and identified 19 gene clusters of at least 10
genes and an intraclass correlation coefficient .0.70 (Supplemental
Data). Among them, we identified gene signatures tracking lympho-
cyte activation/infiltration (e.g. CD8A and CD2), ectodermal
development (e.g. keratin 6B and 15), interleukin-8 pathway (e.g.
IL8 and CXCL1), tight junctions (e.g. claudin-3 and occludin),
proliferation (e.g. budding uninhibited by benzimidazoles 1 homo-
log [BUB1] and CENPA) and interferon-response pathways (e.g.
STAT1 and interferon-induced protein with tetratricopeptide repeats
1 [IFIT1]) (Fig. 6).
Common patterns of gene signature expression across cancer
types.Similar to the previous analysis, we determined the expres-
sion scores of 329 gene signatures (or modules)
20
in all samples,
including 115 previously published signatures, and then performed
an unsupervised hierarchical clustering (Fig. 7). Thirteen clusters of
at least 5 signatures and an intraclass correlation coefficient .0.70
were identified. These groups of gene signatures were found to track
various types of biological processes/features likely coming from the
tumor cell, the microenvironment or both. Interestingly, the expres-
sion of signatures tracking microenvironment-related (e.g. lympho-
cyte activation/infiltration) biological processes were found to be less
cancer type specific than the expression of gene signatures tracking
tumor-related biological processes (e.g. proliferation).
To illustrate the overlap among cancer types regarding the
expression of a single signature, we evaluated 6 previously identified
gene signatures that are known to track various cancer-related and
stromal/microenviroment-related biological processes related to
breast cancer biology
21,47–51
. The results showed that high expression
of these signatures (i.e. the top 20% expressers in the unified dataset)
occurs across all cancer types, albeit with different proportions
(Fig. 8). Of note, the TP53 signature
21
, which was trained in a prev-
iously reported breast cancer dataset, predicted TP53 somatic muta-
tions in the combined TCGA dataset (area under the receiver
operating characteristic curve 50.782; Suppl. Fig. 3). Moreover,
the scores of the previously reported PTEN-loss signature were
found correlated with INPP4B (correlation coefficient 520.424,
p-value ,0.0001) and phospho-4E-BP1 (correlation coefficient 5
0.368, p-value ,0.0001) protein expression in the TCGA breast
cancer dataset (Suppl. Fig. 4).
Breast cancer intrinsic subtyping of non-breast tumors.To evalu-
ate if the breast cancer ‘intrinsic’ profiles (Luminal A, Luminal B,
Figure 3
|
Transcriptomic relationships among cancer types. Relationships have been determined by calculating the Euclidean distances of each sample
to each of the 7 centroids, which represent each cancer type, using all genes of the unified dataset. Clustering has been performed after median
centering the Euclidean distances of each sample. The following genomic relationships among cancer types are shown based on the following subsets of
patients: (A) all patients (ALL); (B) basal-like breast cancer (BASAL-LIKE); (C) ovarian cancer (OVARIAN); (D) squamous cell lung cancer (SQCLC);
(E) lung adenocarcinoma (LUAD); (F) colorectal adenocarcinoma (CCR); (G) glioblastoma multiforme (GBM); (H) non-Basal-like breast cancer
(BREAST).
www.nature.com/scientificreports
SCIENTIFIC REPORTS | 3 : 3544 | DOI: 10.1038/srep03544 4