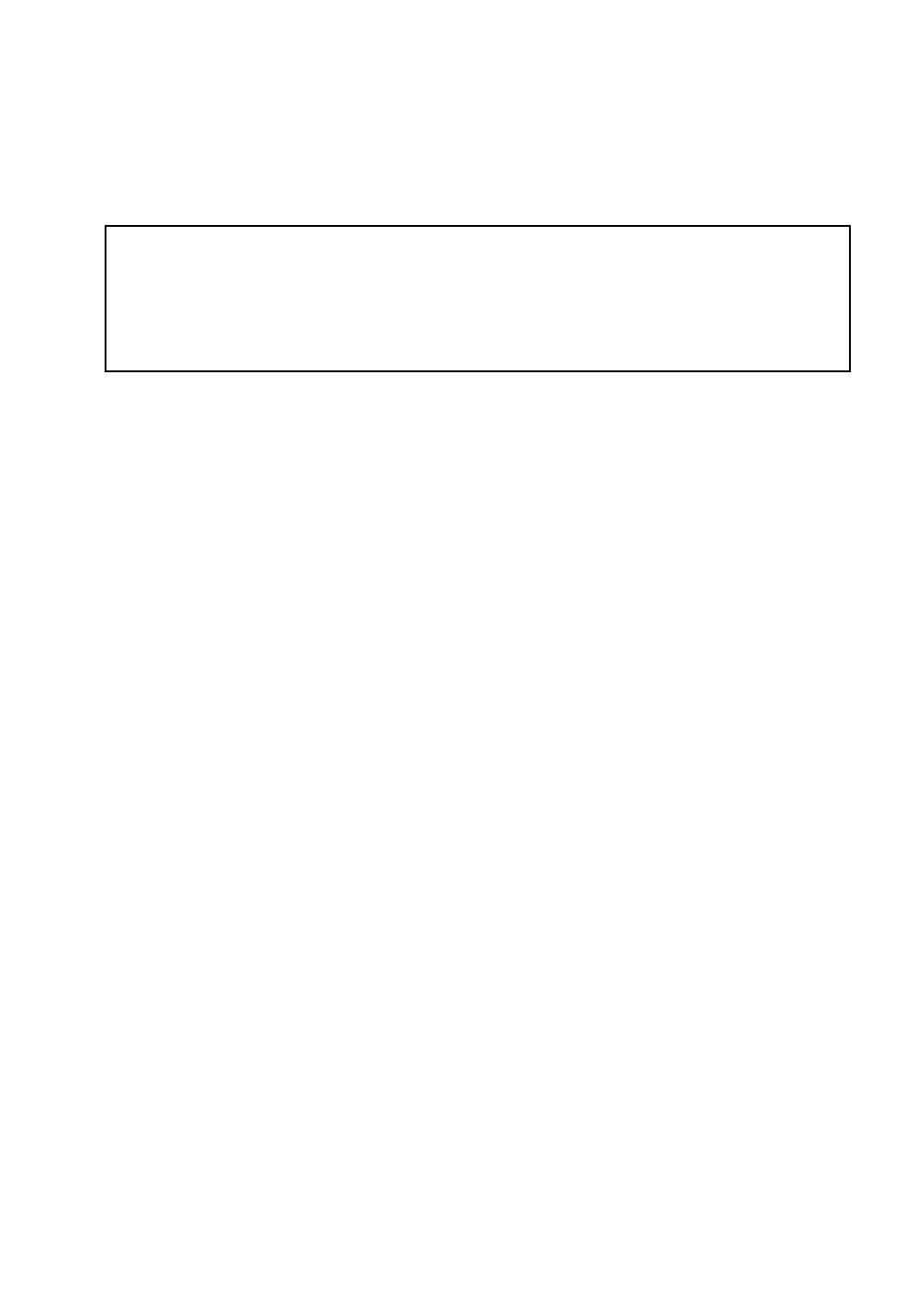
REMERCIEMENTS
Je voudrais tout d’abord exprimer mes plus profonds remerciements à Ghislain sans qui
cette thèse n’aurait pas eu lieu, et qui en plus d’un encadrement sans faille depuis le début
a été une source de conseils tout au long de cette thèse. Je remercie de même François
pour toute l’aide qu’il a pu m’apporter, pour la rapidité, la justesse de ses réponses et ses
corrections à toutes heures. Je voudrais également remercier pour les conseils qu’elles m’ont
apportées, toutes les personnes avec qui j’ai collaboré pour cette thèse : Daniel, Pascal,
Arnaud, Sabrina, Raphaële, Renaud.
Je tiens également à exprimer mes plus sincères remerciements à tous les membres de la
plateforme de Bioinformatique Intégrative du
CRCM
qui m’ont supporté malgré eux tout le
long de ma thèse et de mon écriture : Samuel, Olivier, Alexandre, Fanny, Claire, Quentin,
Guillaume. Je voudrais également inclure dans ces remerciements l’ensemble du CRCM :
Françoise et Jean-Paul qui ont soutenu ce projet dès le début, Caroline pour avoir été un
soutien moral sans faille, Sébastien, Will, Sébastien, Marie-José, Yves, Khedidja, Avais, MLA,
MLT, Vincent, Javier, Émilie, Marion, Julien, Armelle, Axelle, Pascale, Bernard, Myriam,
Julie, François, Laurence D, Laurence L et je présente mes excuses à tous ceux que j’aurais
très probablement oublié...
Je souhaite aussi adresser mes remerciements à tous mes amis qui m’ont soutenu ces
dernières années, mais qui sont trop nombreux pour les citer tous ici.. .
J’exprime toute ma gratitude à mes parents Claude et Marie-Claude, mon frère Florent,
Karine et leur trois enfants Robin, Thibaud et Tristan ainsi que tout le reste de la famille.. .
Je conclurai en remerciant de tout mon cœur Célia pour son aide et son soutien.
iv