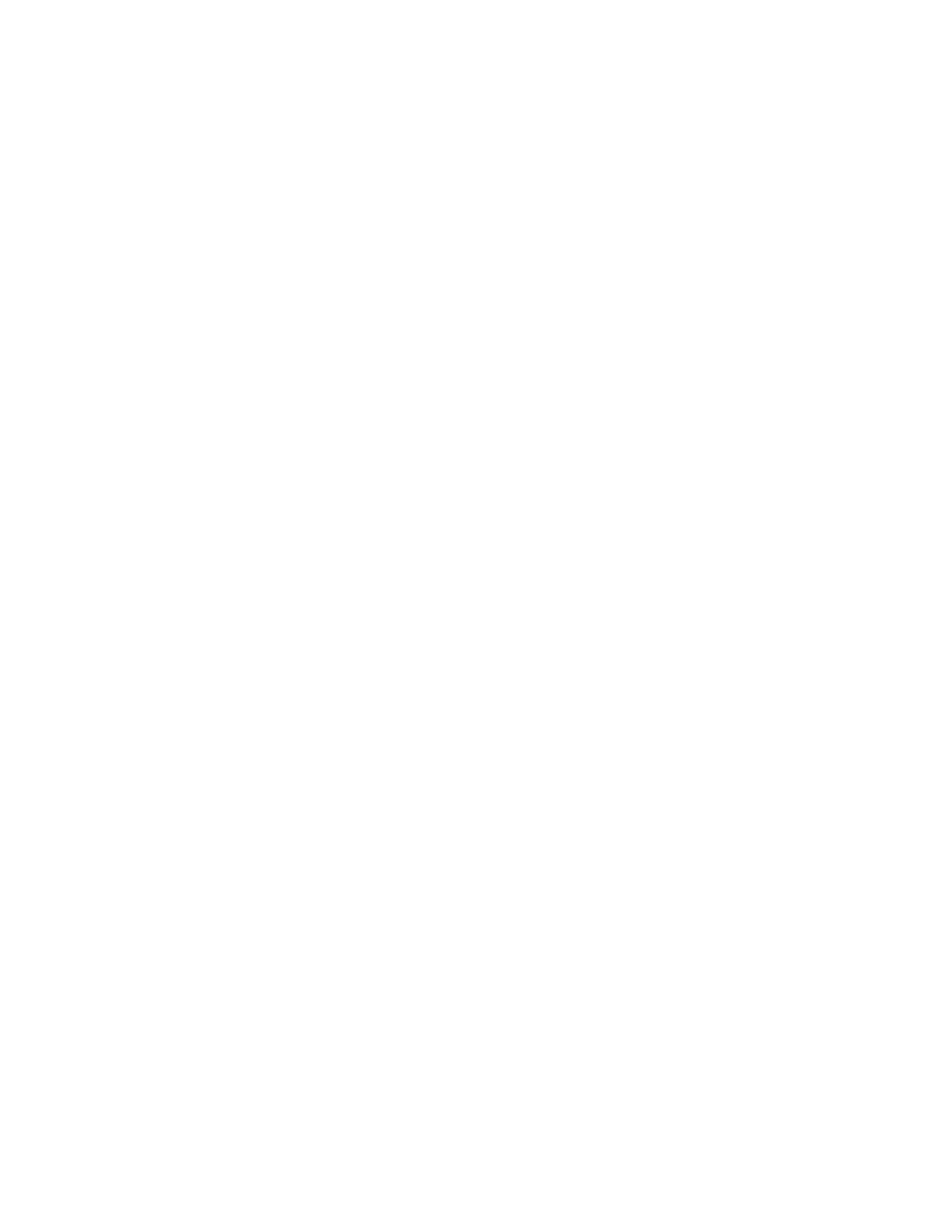
A/R continu memory-based 5
A terme, nous étendrons notre algorithme dans un cadre multi-critères, afin de
gérer efficacement les divers types de récompenses et punitions qu’un agent plongé
dans un jeu vidéo est susceptible de recevoir de son environnement.
7. Conclusion
Dans cette contribution, nous avons appliqué l’algorithme case-based de Santa-
maria, Sutton et Ram au problème Mountain-Car, et nous avons comparé ses perfor-
mances à l’algorithme instance-based de Smart et Kaelbling.
Nos résultats ont montré que le premier convergeait plus vite que le second, ce
qui répond à nos attentes pour appliquer ce type de techniques à des problèmes de
navigation dans les jeux vidéo.
8. Bibliographie
[ATK 91] ATKESON C. G., « Memory-Based Control Learning », Proceedings of the 1991
American Control Conference, New York, NY, 1991.
[BAI 94] BAIRD L. C., « Reinforcement Learning in Continuous Time : Advantage Upda-
ting », Proceedings of the International Conference on Neural Networks, Orlando, FL,
1994.
[HAR 95] HARMON M. E., BAIRD L. C., « Residual Advantage Learning Applied to a Dif-
ferential Game », Proceedings of the International Conference on Neural Networks, Wa-
shington, D.C., 1995.
[MOO 94] MOORE A. W., « The Parti-Game Algorithm for Variable Resolution Reinforce-
ment Learning in Multidimensional State-Spaces », HANSON S. J., COWAN J. D., GILES
C. L., Eds., Advances in neural information processing systems, Morgan Kaufmann, San
Mateo, CA, 1994.
[MUN 01] MUNOS R., MOORE A., « Variable Resolution Discretization in Optimal Control »,
Machine Learning, vol. 49, 2001, p. 291-323.
[SAN 97] SANTAMARIA J.-C., SUTTON R., RAM A., « Experiments with Reinforcement
Learning in Problems with Continuous State and Action Spaces », Adaptive Behavior,
vol. 6, no2, 1997, p. 163–218.
[SMA 00] SMART W. D., KAELBLING L. P., « Practical Reinforcement Learning in Conti-
nuous Spaces », 17th International Conference on Machine Learning, 2000.