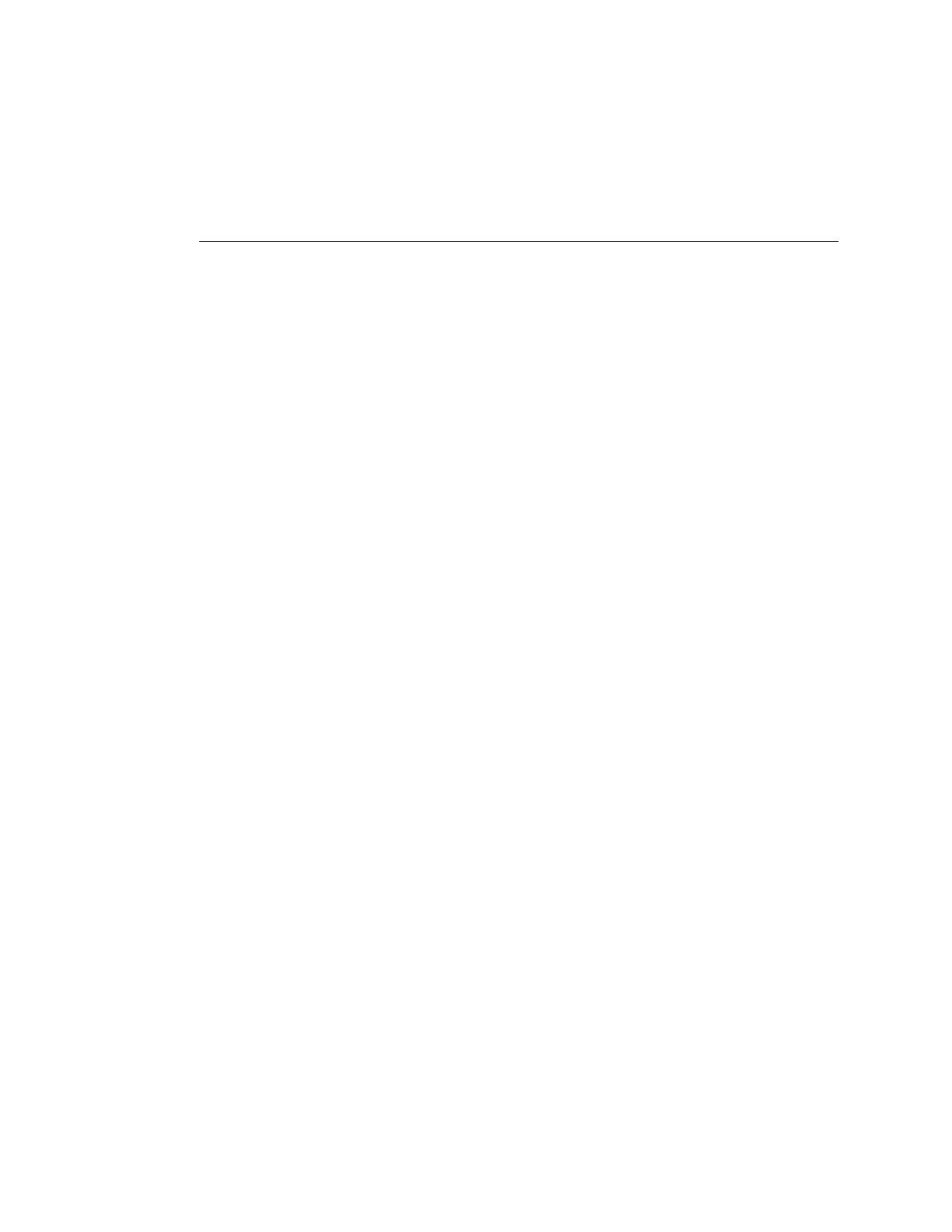
v
SUMMARY
Markov Chain Monte Carlo (MCMC) algorithms are methods that are used
for sampling from probability distributions. These tools are based on the path
of a Markov chain whose stationary distribution is the distribution to be sam-
pled. Given their relative ease of application, they are one of the most popular
approaches in the statistical community, especially in Bayesian analysis. These
methods are very popular for sampling from complex and/or high dimensional
probability distributions.
Since the appearance of the first MCMC method in 1953 (the Metropolis algo-
rithm, see [10]), the interest for these methods, as well as the range of algorithms
available, continue to increase from one year to another.
Although the Metropolis-Hastings algorithm (see [8]) can be considered as
one of the most general Markov chain Monte Carlo algorithms, it is also one of
the easiest to understand and explain, making it an ideal algorithm for beginners.
As such, it has been studied by several researchers. The multiple-try Metropolis
(MTM) algorithm , proposed by [9], is considered as one interesting development
in this field, but unfortunately its implementation is quite expensive (in terms of
time).
Recently, a new algorithm was developed by [1]. This method is named the re-
visited multiple-try Metropolis algorithm (MTM revisited), which is obtained by
expressing the MTM method as a Metropolis-Hastings algorithm on an extended
space.
The objective of this work is to first present MCMC methods, and subse-
quently study and analyze the Metropolis-Hastings and standard MTM algo-
rithms to allow readers a better perspective on the implementation of these me-
thods. A second objective is to explore the opportunities and disadvantages of
the revisited MTM algorithm to see if it meets the expectations of the statistical
community. We finally attempt to fight the sedentarity of the revisited MTM al-
gorithm, which leads to a new algorithm. The latter performs efficiently when the
number of generated candidates in a given iteration is small, but the performance