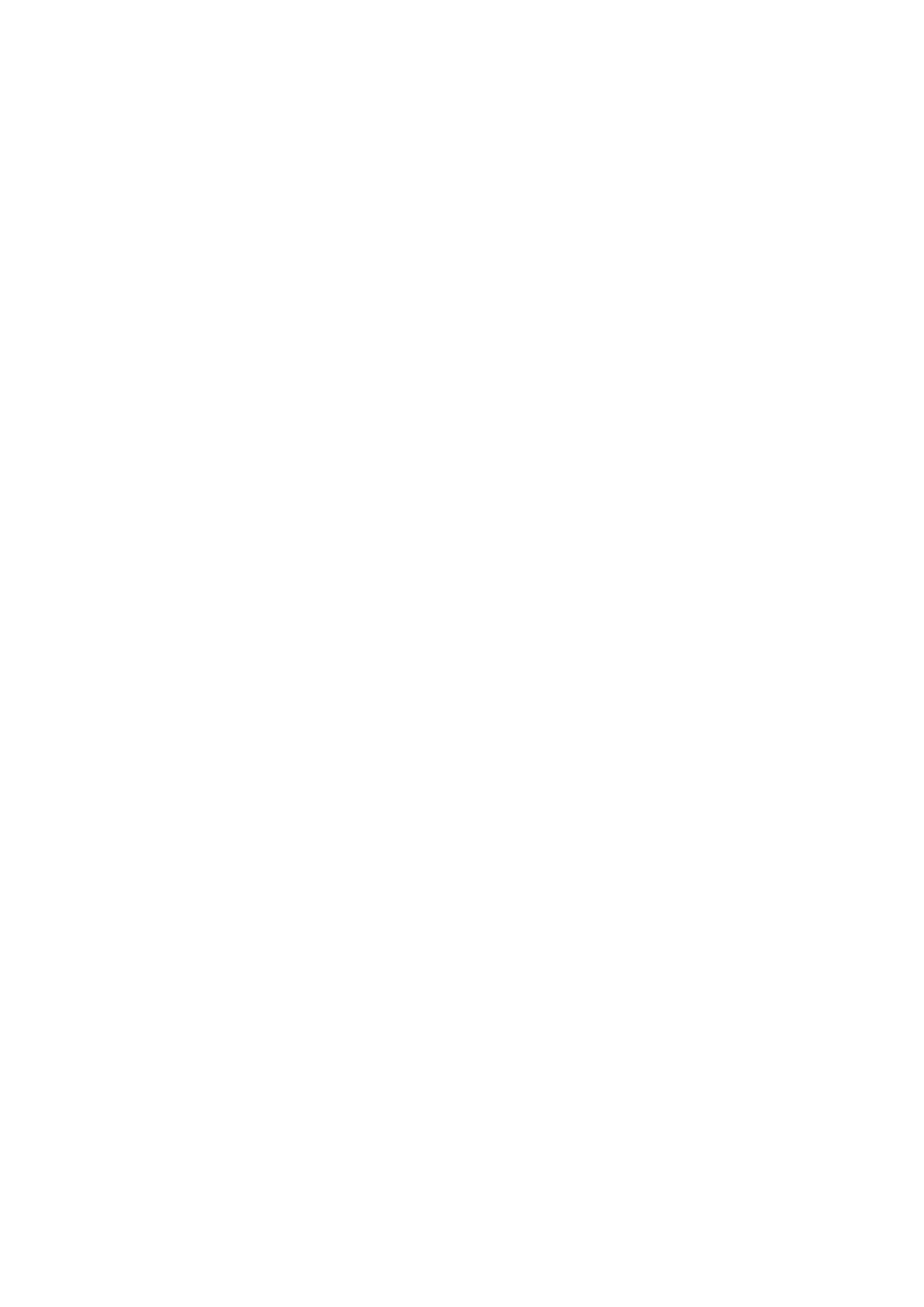
Abstract
The purpose of this short paper is to present the new results of experi-
ments led by the Physical Microfluidics and Bioengineering team in Institut
Pasteur. The results are obtained with an original code for the software
ImageJ®. It automatically analyzes photographs to detect as much cells as
possible without being disturbed by the flaws present on the photograph.
This allows the quick analysis of a large number of cells (up to 150 000 per
experiment), which then gives access to really quantitative results in only a
few minutes, which isn’t possible by hand. After having presented the ex-
periments and the objectives of this project, we will go through the ImageJ
code, which we will call the macro, to see how it works and deals with the
difficulties it encounters. This allows other people to use it on similar expe-
riments, or to adjust it depending on what results they are precisely looking
for.
1 Experiments and objectives
1.1 The experiments
The experiments led by the lab in Institut Pasteur are experiments on
DNA reparation. The principle is to introduce a repeated sequence of nucleo-
tides (called micro-satellites) in the DNA of yeast cells, and then see how
good different endonucleases can repair it. Every experiment is done with
thousands of cells. To observe them all, they are placed in a microfluidic
device where they are trapped in small wells (each well is a 100 µm by 100
µm square). Once the cells are in the microfluidic trap, we put the trap in a
microscope where they are regularly photographed. In order to have a better
monitoring of the development of the cells, we introduce the micro-satellites
in the middle of the GFP gene, which is expressed through the fluorescence
of the cell. Therefore, the microscope takes two pictures every time : one pic-
ture in the bright field, and one picture that only detects the fluorescence of
the cells. This allows to check if the GFP gene has been successfully repaired
by the endonuclease.
1.2 Objective of the macro
After multiple experiments, we can observe some anomalies with some
conditions. One of the main concerns is the GAA-SpCas9 case (GAA is
the microsatellite introduced and SpCas9 is the endonuclease used). In this
case, we can observe that some cells are malformed : they are either not
round, significantly bigger than the others or both. The difficulty to evaluate
the proportion of this kind of malformation by hand is what motivated the
creation of a macro that could efficiently analyze all the cells of an experiment
2