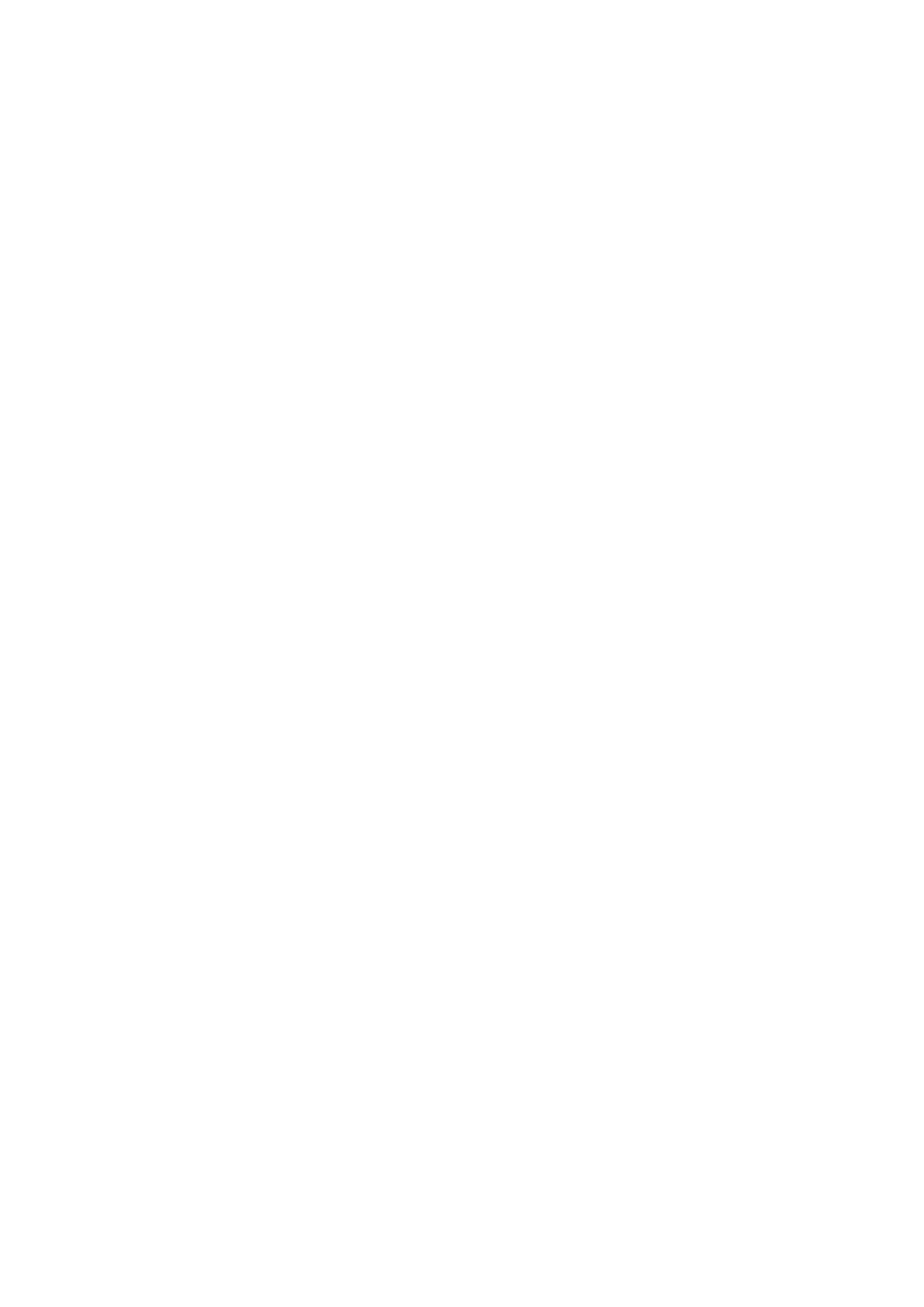
Abstract
Non-orthogonal multiple access (NOMA) has been proposed as a viable multiple access (MA)
technique to meet the demanding requirements in fifth-Generation (5G) and beyond wireless
networks. Unlike conventional orthogonal multiple access (OMA) techniques, NOMA si-
multaneously sends signals to multiple users in the same resource block (RB) in time and
frequency domains using power-domain superposition coding (SC) at transmitter. Therefore,
NOMA has the potential capabilities to serve a large number of devices while significantly
improving spectrum efficiency (SE) compared to the conventional MA techniques, which
supports massive connectivity of Internet-of-Things (IoT) networks.
To introduce additional degrees of freedom, and hence facilitate implementing NOMA
in ultra-dense networks, NOMA has been integrated with different key technologies in-
cluding multiple antenna techniques and conventional OMA techniques. In particular, the
combination between multiple-input single-output (MISO) and NOMA, referred to as MISO-
NOMA, is firstly considered in this thesis. In which, different beamforming designs have
been proposed for MISO-NOMA system, including global energy efficiency maximization
(GEE-Max) design and EE fairness-based designs. In addition, different multi-performance
metrics have been also considered in the designs including GEE-SE design and fairness-sum
rate design. Due to non-convexity of the formulated optimization problems, different convex
relaxation and approximation techniques have been exploited throughout the thesis to ap-
proximate the original non-convex problems with convex problems. The performance of the
proposed designs has been evaluated through drawing comparisons with that of the existing
beamforming designs in the literature.
Secondly, the combination of NOMA with OMA scheme has been investigated, partic-
ularly, energy harvesting (EH) capabilities of time division multiple access (TDMA) and
NOMA system has been considered. In this hybrid TDMA-NOMA system, simultaneous
wireless information and power transfer (SWIPT) technique is integrated such that user has
the capability to harvest energy and decode information, simultaneously. Simulation results
show that EH capabilities of the TDMA-NOMA system outperform that of the conventional
TDMA system.