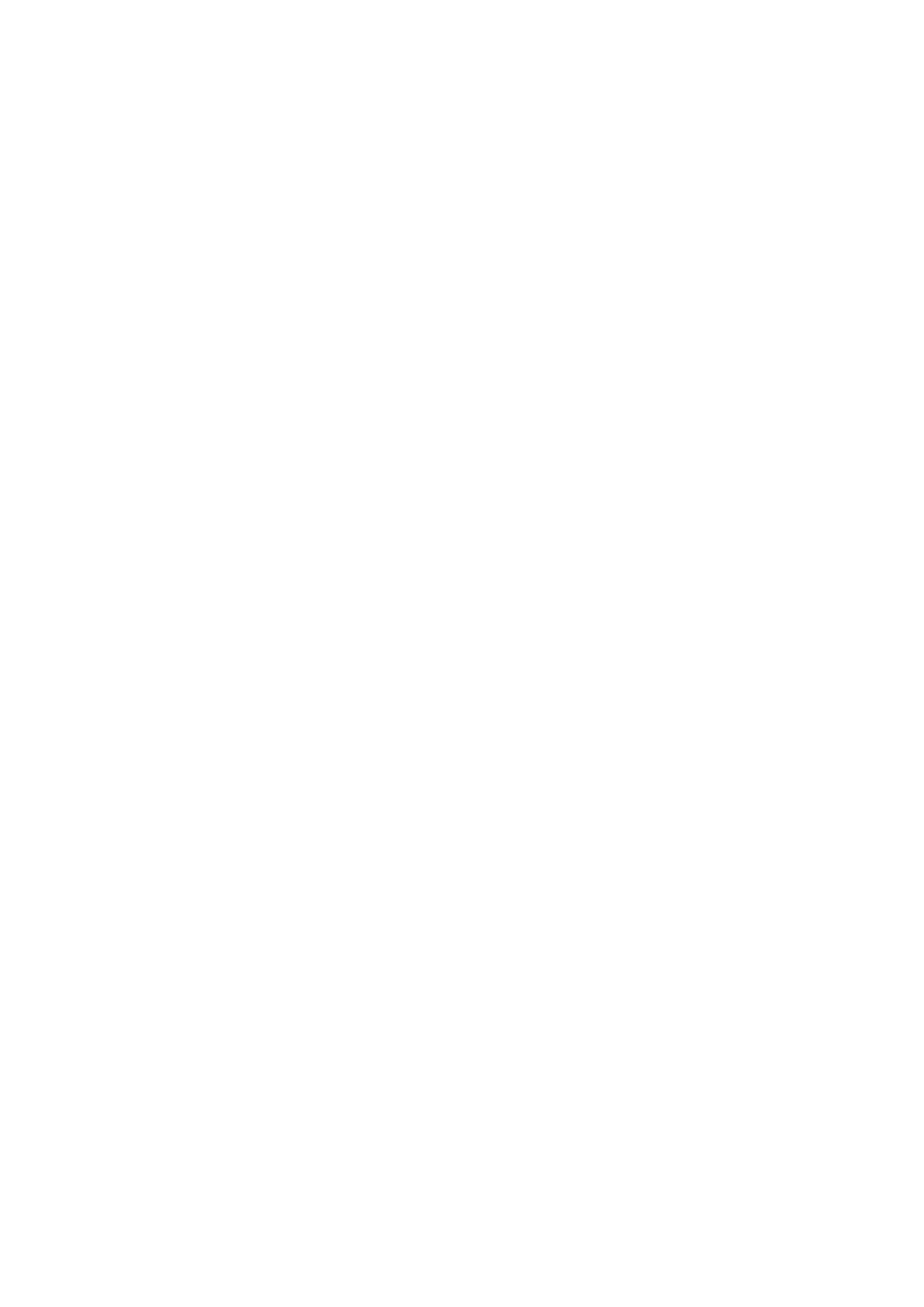
measured in parallel, thus it can’t realize real-time position in WIFI scenario. Experimental results
show that the 80% accuracy is about 2 m and 90% accuracy is about 3 m, with three APs being taken
part in. CUPID [21] transforms the CSI to time domain and measures the range by considering the first
bar of the energy distribution graph to be the line-of-sight. This will result in large errors in real
environment, because there exists an uncertain time lag within the shortest delay. Experimental results
show that the 80% accuracy is about 3m and 90% accuracy is about 4m, with five APs being taken part
in. In [22-23], a DeepFi system is proposed, in which the fingerprinting is the weights of a network
trained with a greedy learning algorithm in the offline training stage, and a probabilistic method is
used to locate the target’s position in the online positioning stage. However, the phase information of
CSI is not used for the building of fingerprinting. In [24-25], a PhaseFi system is proposed where the
phase information is used to build the fingerprinting database and a greedy learning algorithm is used
to train the weights, however, it doesn’t use the amplitude information.
In this paper, we propose a fingerprinting-based Amp-Phi indoor positioning system, in which the
amplitude and phase information are used at the same time to build the fingerprinting database. The
basic ideas can be concluded as: Firstly, according to the characteristics of the raw CSI information
collected at different positions under different environments, we build a NLOS mitigation model and
phase error mitigation model respectively to process the amplitude and phase of CSI. Secondly, we
analyze the statistical characteristics of CSI carefully, including maximum, minimum, mean and
variance. After being processed with the models, the CSI features can be used to distinguish different
positions clearly, which provides a theoretical basis for the indoor positioning based on fingerprinting.
Finally, we build a fingerprinting database based on the amplitude and phase features of CSI and
realize the target’s localization with the K-Nearest Neighbor (KNN) matching algorithm. We perform
some experiments in two typical scenarios: one is the complex computer laboratory where exist a lot of
NLOS due to the block of furniture and computers, the other is the relative simple living room, there is
little NLOS for no large things exist. Both in the two environments, we compare the Amp-Phi system
with the newest method PhaseFi proposed in 2016 [25], the most classical methods Horus [26] and ML
[27] proposed in 2005. In the laboratory, the position accuracy of the four system is 4m,4.2m,5.4m and
6m respectively, and in the living room, the position accuracy of the four system is 1.7m,1.8m,2.5m
and 3.7m respectively. That is to say, among the four systems, Amp-Phi performs best and the PhaseFi
system takes the second place, this is because both of them use CSI which has much more fine-grained
subcarriers information than RSSI, for Amp-Phi fuse the amplitude and phase information together, its
position accuracy is better than that of PhaseFi which only use the phase information.
The rest of the paper is organized as follows. In section 2, we collect the raw CSI information and
build the CSI range model. In Section 3, we build a NLOS mitigation model and a phase error
mitigation model respectively to process the amplitude and phase of CSI, and analyze the
characteristics of CSI. In section 4, we build a fingerprinting database based on amplitude and phase at
the same time and realize to locate the target’s position in indoors, and do some experiments in two
typical scenarios. Finally, in section5 we conclude our work.
2 Collection of CSI Data and Establishment of Range Model
2.1 Collection of CSI Data
In the wireless communication protocol, CSI belongs to the physical layer information and can’t
be obtained directly. TNS-CSI Tool, which is an integrated installation tool based on Linux 802.11n
ACCEPTED MANUSCRIPT
Accepted manuscript to appear in IJPRAI
Int. J. Patt. Recogn. Artif. Intell. Downloaded from www.worldscientific.com
by MCMASTER UNIVERSITY on 03/20/18. For personal use only.