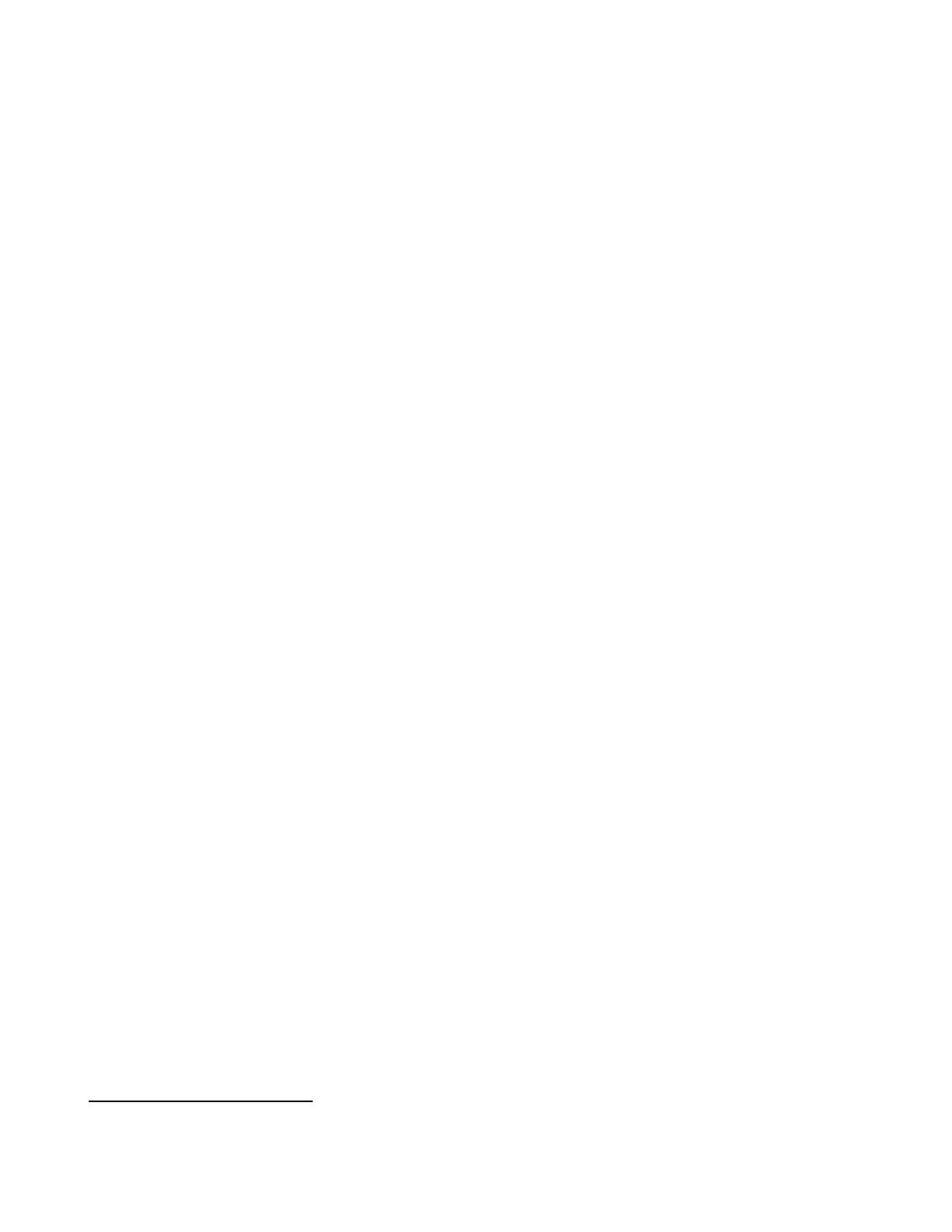
econometric techniques allow to overcome this problem and each of the described FDI motivations has
a different implication in term of spatial relations
.
Following Blonigen et al. (2007) in this section we fill focus on the correlation between the level of FDI
in a country and in its neighbors, and how the surrounding market potential influences the level of FDI
in a country. However, our estimation will reveal the importance of other sources of externalities.
Horizontal FDI arise with the objective to avoid high trade costs for the parent companies, which are
usually due to import barrier imposed by host countries. In pure horizontal models the only trade off
faced by companies when deciding to establish foreign affiliates is whether the trade cost avoided from
operating directly in the host market is greater than the set-up costs. In this framework, surrounding
market potential or FDI level in neighbor countries will not play any role in the final decision outcome.
For this theoretical prediction to be realistic, however, one needs to assume that all destination markets
have sufficiently high trade protection against imports from other destination markets.
When this is not the case, and the commercial protection among destination countries is lower
compared to trade costs incurred by the parent to export in those markets, it is likely that MNE will opt
for a “export-platform” type of FDI.
Establishing one affiliate in a strategic position will bring the same benefits coming from horizontal FDI
in every destination country, while saving the high set-up costs of multiple plants, since it allows the
company to rich simultaneously all the neighbor markets without incurring in important trade costs.
For this reason, it is plausible to assume that the surrounding market potential will have a positive effect
on the level of FDI in the host country of interest. At the same moment, since fixed costs coming from
setting-up multiple plants are much higher than trade costs between neighbor countries, the decision
of a parent company to establish an affiliate in a country will likely come at the cost of not establishing
in the neighbors, which translates in a negative sign of the spatial lag on the FDI.
Vertical FDI model implies that companies choose to invest in the host country which offers the lowest
production costs for the activity which needs to be relocated. Analogously to what happens for export-
platform, the decision of a pure vertical MNE to invest in a country comes at the expense of neighboring
countries. On the other hand, since in this kind of MNE the final destination of products remains the
home market, the surrounding market potential is supposed to have an insignificant effect on the
volume of FDI in the host country.
In complex-vertical MNEs the production process is “fragmented” across different countries.
Consequently, a dense supplier network in a region can decrease the costs of separating productive
activities. Moreover, there might be other agglomeration forces (e.g. location of immobile resources
as mines, IT districts) which, as long they concern the production process of parent companies, can
attract FDI. In this sense, it is plausible to expect that both spatial lag on FDI and on market potential
can have a positive impact on the FDI inflow into a host country.
3. Data and methodology
The analysis to understand the determinants and pattern of US investment abroad is performed over
85 host countries for the year 2013. The database includes 14 Asian and Pacific (AP) countries, 29
It exists a multitude of spatial relations which will be presented in the following sections. Details on the econometric model and
techniques will be provided in section 5.