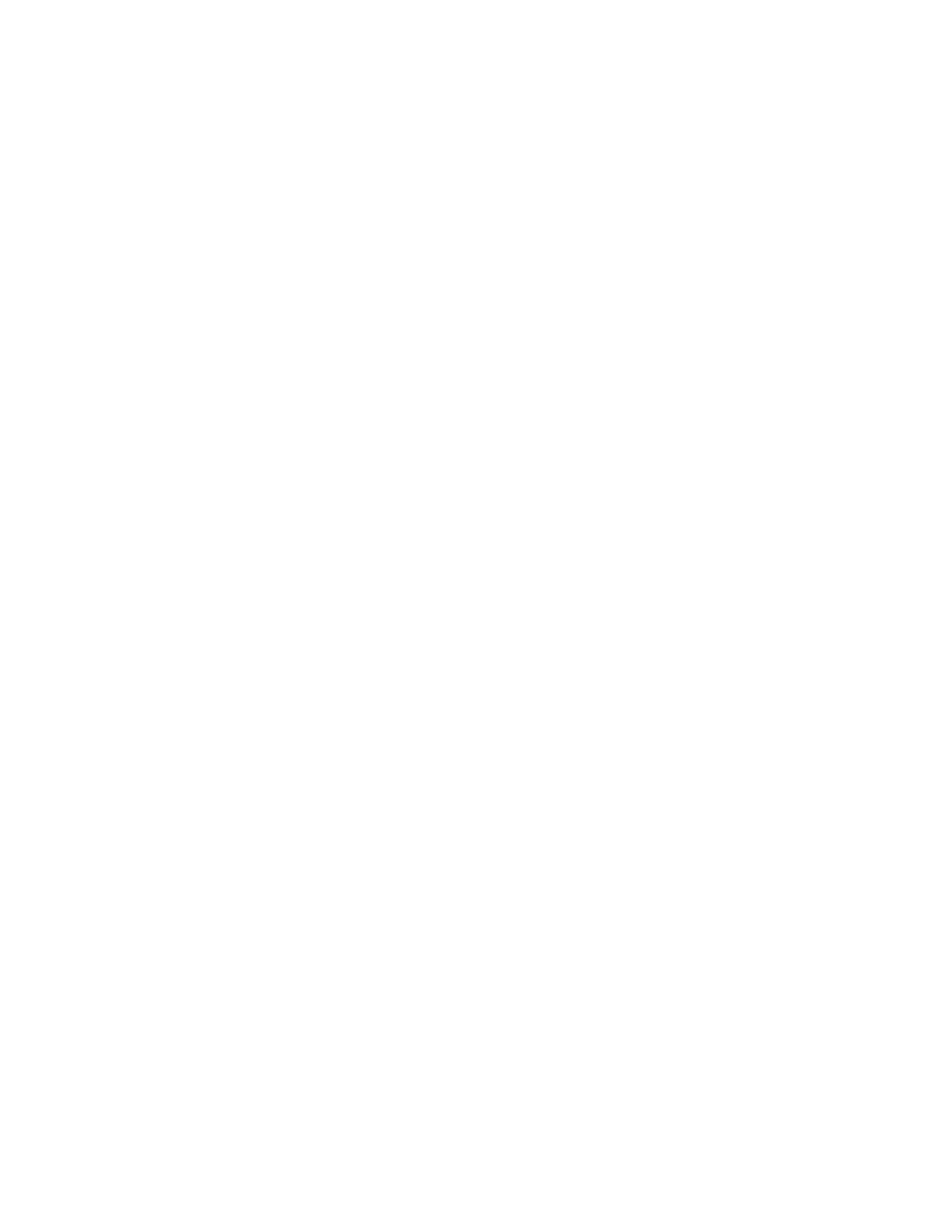
3
AGRADECIMENTOS
Aos meus pais, pelo amor e apoio incondicional durante mais essa etapa. A coragem e
determinação que sempre demonstram é meu maior exemplo. Obrigado pela educação e
auxílio em todos os momentos, esta conquista pertence a vocês.
A Lia Mara Gomes Paim, é ela que compartilha o dia-a-dia comigo, que compartilha a
paixão pela ciência, que compartilha os dias difíceis e os momentos felizes. E por ser o motivo
de meu sorriso ao início de cada dia.
A toda a minha família, pelas risadas, churrascos e apoio.
Aos amigos de Boston, Florianópolis, Marau, Passo Fundo, Porto Alegre, Vienna. Tenho
muita sorte em possuir tantos amigos queridos em tantos lugares. Obrigado por todos os
momentos compartilhados. Vocês também são partes fundamentais dessa conquista
Ao governo do Brasil e as agências de fomento, que forneceram os recursos estruturais
e financeiros para que este trabalho fosse realizado. Ao governo dos Estados Unidos, por
disponibilizar de maneira gratuita bancos de dados e o PubMed, fundamentais para esse
trabalho.
Aos amigos e professores da UFCSPA e da UFRGS pelos ensinamentos.
Ao pessoal do instituto de informática da UFRGS, especialmente ao Bernardo Henz pelo
trabalho em conjunto e sem o qual este trabalho não teria sido possível e, ao meu co-
orientador, Manuel Menezes de Oliveira Neto pelos ensinamentos e disponibilidade em aceitar
esse desafio em conjunto conosco.
Ao meu orientador Guido Lenz, por todos os ensinamentos e paciência, por acreditar em
minha capacidade e corrigir minhas (não tão infrequentes) crises de autocrítica. Sua paixão pela
ciência e seu conhecimento são os dois aspectos que almejo reproduzir durante minha carreira
científica. Também a sua esposa Márcia Rosângela Wink que muito me ajudou durante a
faculdade e também sempre me apoiou.
A todos os amigos do laboratório de sinalização celular, incluindo aqueles que não mais
trabalham ali, mas os quais eu já tive o prazer de conviver. Obrigado por tornarem esse um
ambiente de trabalho incrível e do qual me orgulho em fazer parte. E, especialmente, por
aguentar todo dia o meu mau humor matinal.
A Lia Mara Gomes Paim, Mardja Manssur Bueno e Silva e Pitia Flores Ledur pela leitura
crítica do manuscrito e sugestões.
Aos grandes nomes da ciência sobre os ombros os quais me apoio e a todas as pessoas
que apoiam o progresso da ciência.