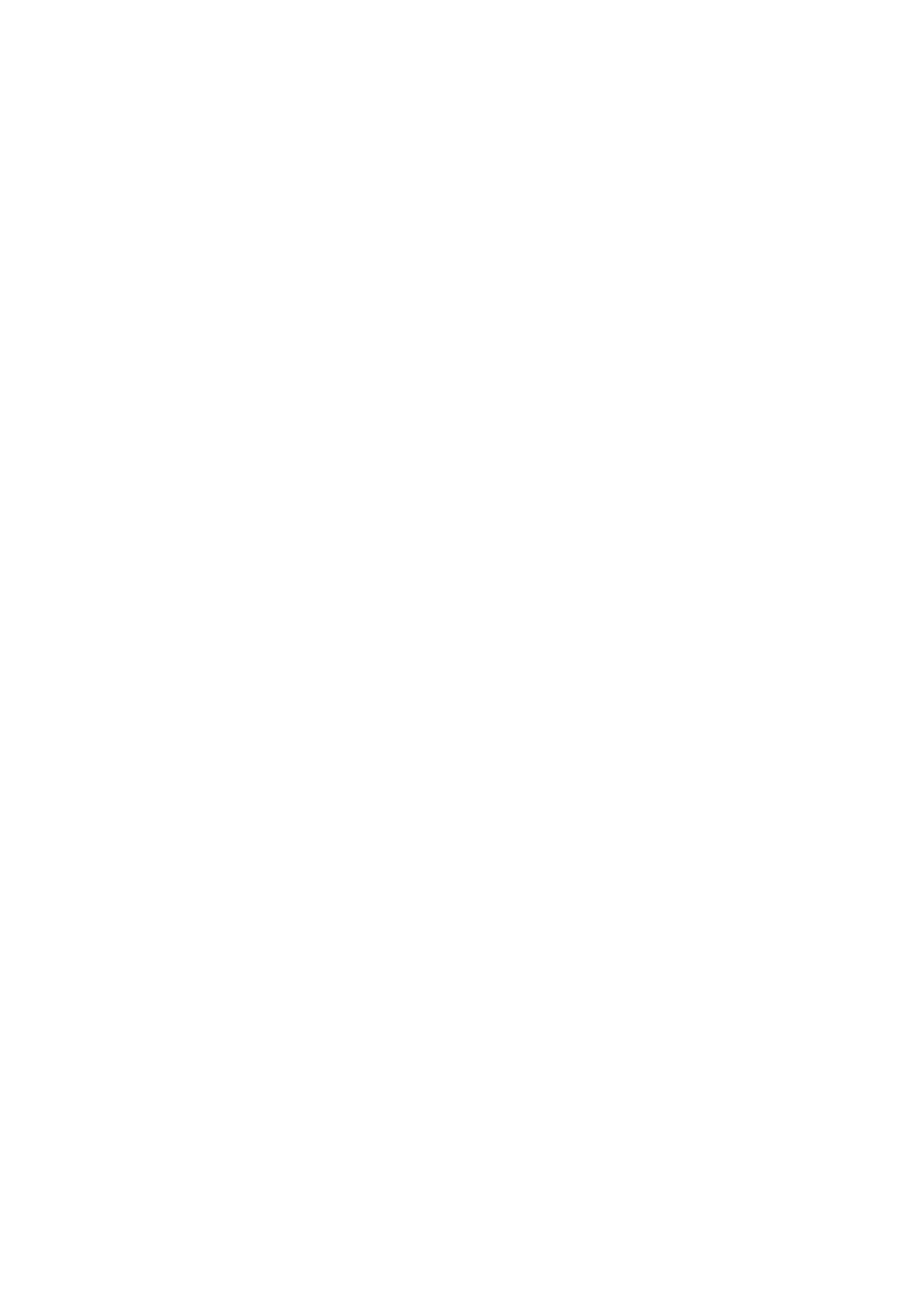
AGRADECIMIENTOS
Esta sección de la tesis es, generalmente, la primera (o única) que se lee pero la última
que se escribe. O por lo menos en mi caso ha sido así. Mientras redactaba esta tesis
me iba dando cuenta de la cantidad de personas que han colaborado, contribuido,
aconsejado… o simplemente estado a mi lado o dejado huella en algún punto de mi
desarrollo como científica y como persona. Esta lista es probablemente demasiado larga
como para nombrarlos a todos, pero intentaré hacerlo lo mejor que pueda.
Por supuesto, la primera alusión ha de ser para mis directores. A vosotros, gracias por
creer en mí y por darme la oportunidad y los medios necesarios para llevar a cabo este
proyecto.
Me gustaría hacer una mención especial para Marina, que además guiarme en
cuestiones de ciencia me ha dado infinitas lecciones de optimismo y de motivación para
hacer un trabajo bien hecho.
Tampoco me puedo olvidar del equipo de clínicos del Servicio de Urología del Hospital
Vall d’Hebron, así como de los patólogos, auxiliares y enfermeras, que han hecho
posible el contacto con los pacientes y la recogida de muestras.
Como cualquiera que se dedique a la investigación sabrá, durante la realización de una
tesis doctoral el laboratorio pasa a convertirse en tu segunda casa (¡y a veces casi
parece que en la única!). Por eso, por hacer de estas cuatro paredes un lugar en el que
me gusta estar, por haber compartido penas y glorias, lágrimas, frustraciones y muchas
risas, quiero darle las gracias a las chicas que me han acompañado en este camino:
Tati, Lucía, Irene, Elena, Blanca, Laura, Melánia, Eva, Mireia… También a mis ex-
compis de oficina Marta y Núria, ¡os he echado de menos! Y a Tuğçe, que aunque hace
tiempo que cambió de rumbo, nada de esto habría sido lo mismo sin ella.
Gracias al Dr. Michiel Pegtel, por acogerme como parte de su equipo y guiarme durante
mi estancia. Gracias también a Rubina, Frederik, Niala, Monique y Danijela, por
hacerme sentir como en casa, por vuestros consejos, y por todos los buenos ratos
dentro y fuera del laboratorio.
También a todos los demás colaboradores que han participado en este proyecto.
Especialmente a Alex Campos y Cristina Chiva por la gran labor de análisis de datos
con la que han contribuido a la realización de esta tesis.