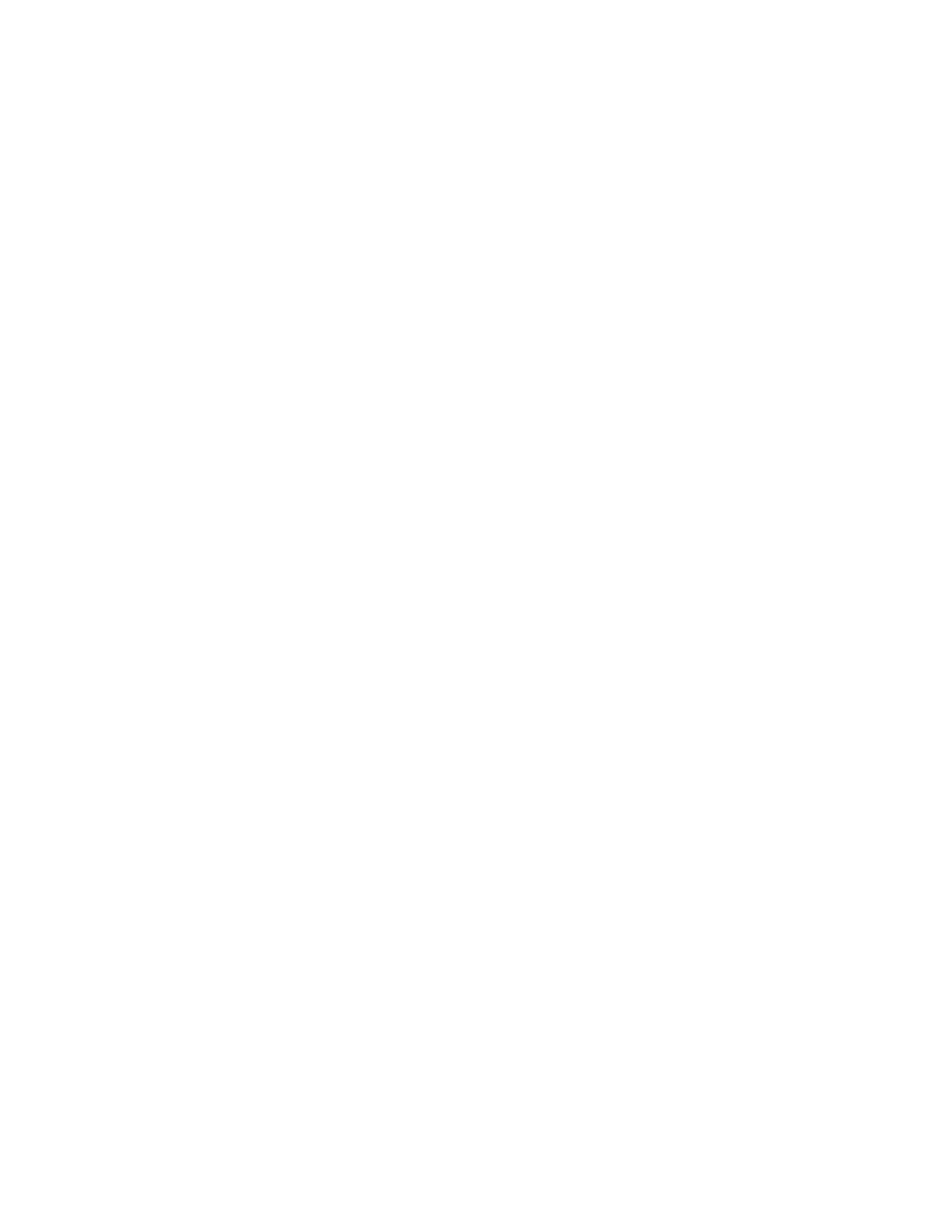
iii
Thesis advisor Author
Richard Wilson Francesco Pompei
Cancer Turnover at Old Age
Abstract
It has been commonly assumed for a half century that if a person lives long
enough, he or she will eventually develop cancer. Cancer age-specific incidence data
does not support that assumption, showing instead that incidence flattens at about age 80
and declines thereafter, approaching zero where there is data at about age 100. Previous
cancer models of long standing have been unable to explain the turnover in incidence
beyond age 80, and investigators have tended to ascribe under-reporting of cancers, or
that the oldest persons are somehow not susceptible, as explanations for the data. This
work presents the first model of cancer incidence incorporating the biological process of
cellular replicative senescence. The model provides good fits to human cancer age
distribution data for 40 organ sites from databases from the U.S., Holland, and Hong
Kong. Newly developed mice data from a 24,000 mice study has also been found to be
well fit by the model, confirming that cancers peak at about 80% of lifespan and actually
reach zero for the oldest mice. The model, mathematically a form of Beta function, is
derived by adding to standard multi-stage or clonal expansion models the observation
that in vitro data show aging cells lose their proliferative ability with age. Since
senescent cells cannot produce cancer, the pool of cells available to produce cancer
declines, thus lowering the incidence, reaching zero when all cells are senescent.
Further tests of the model performed against data on interventions that might alter