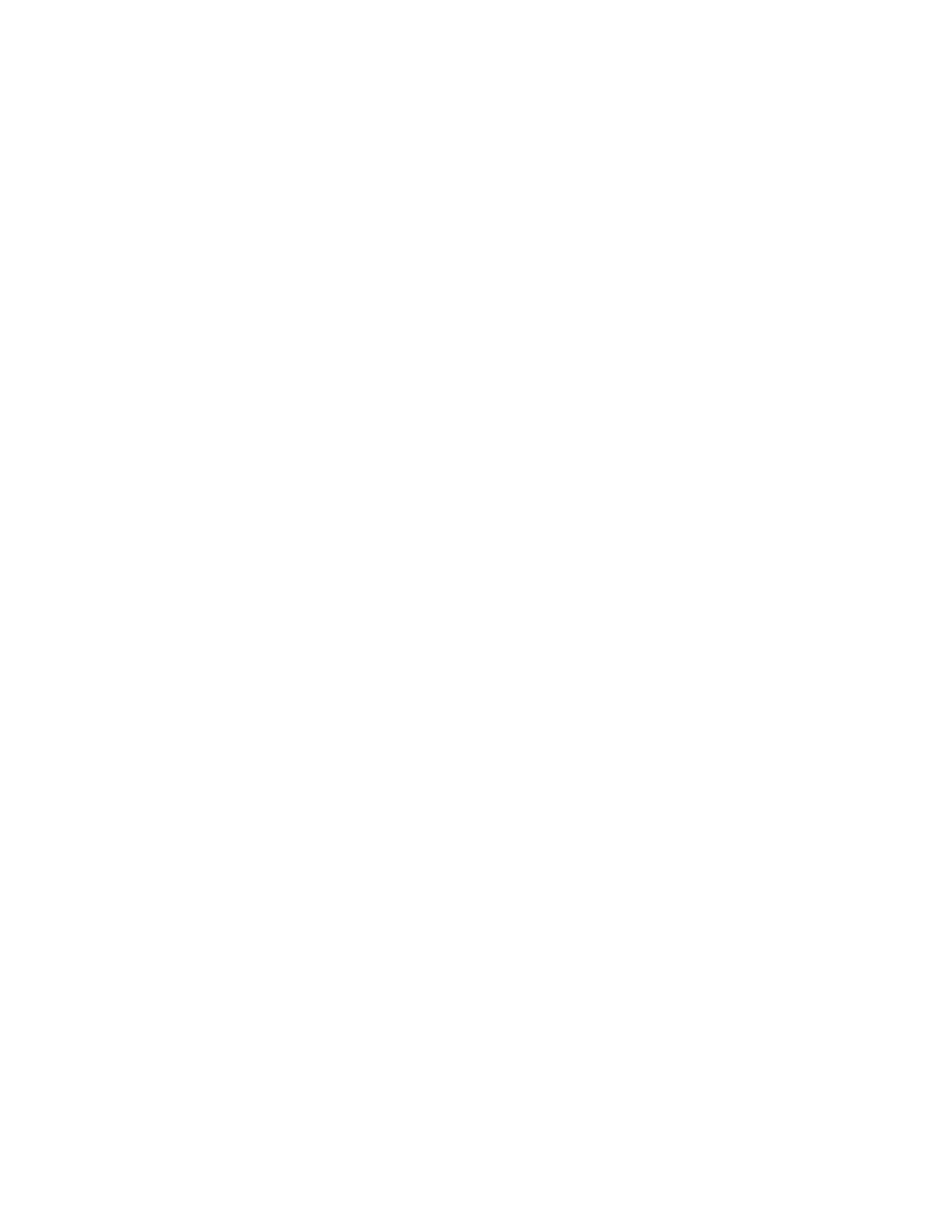
Stochastic Model Checking 5
The atomic propositions used to label states are taken from the set AP =
{try,fail,succ}. Here, the DTMC models a simple process which tries to send
a message. After one time-step, it enters the state s1from which, with proba-
bility 0.01 it waits another time-step, with probability 0.98 it successfully sends
the message, and with probability 0.01 it tries but fails to send the message. In
the latter case, the process restarts. The labelling function allows us to assign
meaningful names to states of the DTMC:
L1(s0) = ∅, L1(s1) = {try}, L1(s2) = {fail}and L1(s3) = {succ}.
3.1 Paths and Probability Measures
An execution of a DTMC D= (S, ¯s, P, L) is represented by a path. Formally, a
path ωis a non-empty sequence of states s0s1s2. . . where si∈Sand P(si, si+1)>
0 for all i>0. A path can be either finite or infinite. We denote by ω(i) the ith
state of a path ω,|ω|the length of ω(number of transitions) and for a finite path
ωfin , the last state by last(ωfin ). We say that a finite path ωfin of length nis a
prefix of the infinite path ωif ωfin (i) = ω(i) for 0 6i6n. The sets of all infinite
and finite paths of Dstarting in state sare denoted PathD(s) and PathD
fin (s),
respectively. Unless stated explicitly, we always deal with infinite paths.
In order to reason about the probabilistic behaviour of the DTMC, we need
to determine the probability that certain paths are taken. This is achieved by
defining, for each state s∈S, a probability measure Prsover the set of infi-
nite paths PathD(s). Below, we give an outline of this construction. For further
details, see [41]. The probability measure is induced by the transition proba-
bility matrix Pas follows. For any finite path ωfin ∈PathD
fin (s), we define the
probability Ps(ωfin ):
Ps(ωfin )def
=1 if n= 0
P(ω(0), ω(1)) · · · P(ω(n−1), ω(n)) otherwise
where n=|ωfin |. Next, we define the cylinder set C(ωfin )⊆PathD(s) as:
C(ωfin )def
={ω∈PathD(s)|ωfin is a prefix of ω}
that is, the set of all infinite paths with prefix ωfin . Then, let ΣPathD(s)be
the smallest σ-algebra (see Section 2) on PathD(s) which contains all the sets
C(ωfin ), where ωfin ranges over the finite paths PathD
fin (s). As the set of cylinders
form a semi-ring over (PathD(s), ΣPathD(s)), we can apply Theorem 1 and define
Prson (PathD(s), ΣPathD(s)) as the unique measure such that:
Prs(C(ωfin )) = Ps(ωfin ) for all ωfin ∈PathD
fin (s).
Note that, since C(s) = PathD(s) and Ps(s) = 1, it follows that Prsis a
probability measure. We can now quantify the probability that, starting from a
state s∈S, the DTMC Dbehaves in a specified fashion by identifying the set of
paths which satisfy this specification and, assuming that this set is measurable,
using the measure Prs.