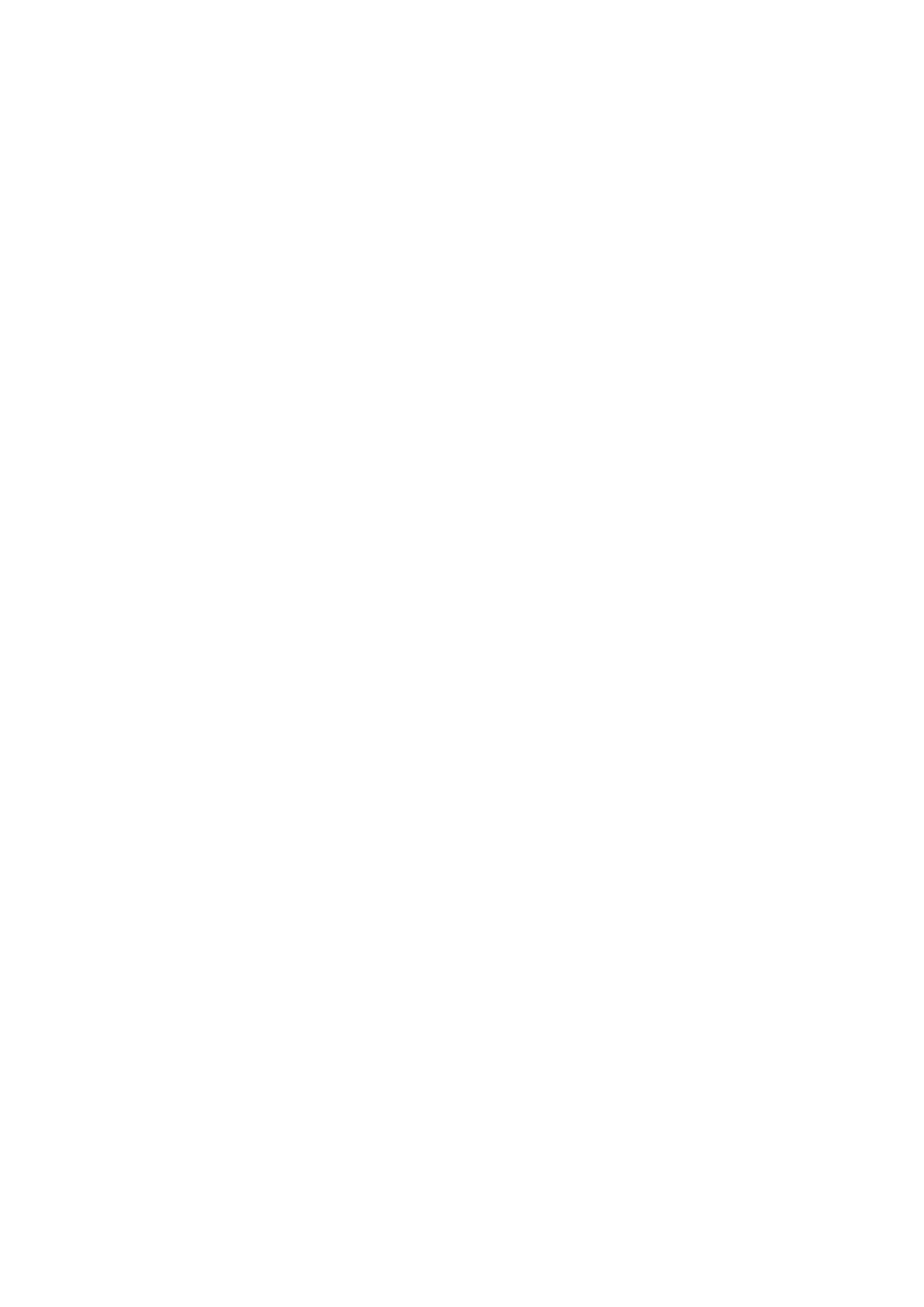
Résumé
La performance d’une méthode de classification est d’un grand intérêt pour le choix, la
comparaison et la validation des algorithmes de classification. La lisibilité des résultats et la
réduction du cout d’échec total de la classification sont d’une importance cruciale pour
l’amélioration de la performance des classifieurs. Dans ce mémoire de magister, nous
proposons principalement deux approches de résolution à travers la description d’un modèle
d’optimisation métaheuristique baptisé ProSadm-HBA (ProSadm : Programmation d’un
Système d’Aide au Diagnostic Médical, en conjonction avec la métaheuristique HBA :
Homogeneity Based-Algorithm) et F-HBA ( Fuzzy Homogeneity Based-Algorithm). Nous
avons validé nos résultats expérimentaux sur des bases de données médicales connues : Pima
(Diabètes), TH (Troubles Hépatiques), AP (Appendicite). Les approches développées
permettent de minimiser le nombre total d’échecs de la classification (ProSadm-HBA) tout en
respectant la contrainte d’interprétabilité des classifieurs (F-HBA). Ces contributions peuvent
être d’un grand intérêt pour les experts dans le domaine médical.
Mots clés: Performances, Sur-Apprentissage, Sur-Généralisation, Métaheuristique, ProSadm,
ANFIS, HBA, Optimisation, Interprétabilité.
Abstract
Performance of a classification method is of great interest for choosing, comparing and
validation of classification algorithms. The transparency of results and the reduction of the total
misclassification cost are of vital importance as well as the improvement of the performance of
classifiers. In this magister report, we propose two solving approaches through the description
of a meta-heuristic optimization model denoted ProSadm-HBA (ProSadm in conjunction with
HBA: Homogeneity Based-Algorithm) and F-HBA ( Fuzzy Homogeneity Based-Algorithm)
.we validated our experimental results based on three well known medical datasets: PID (Pima
Indian Diabetes), LD (Liver Disorders) and AP (Appendicitis) . The developed approaches
allow minimizing the total number of misclassification cases (ProSadm-HBA) while respecting
the interpretability constraint of classifiers (F-HBA). These contributions can be of great
interest for experts in the medical field.
Keywords: Performance, Overfitting, Overgeneralization, Metaheuristic, ProSadm, ANFIS,
HBA, Optimization, Interpretability.
Overfitting, Overgeneralization, Metaheuristic, ProSadm, ANFIS
HBA