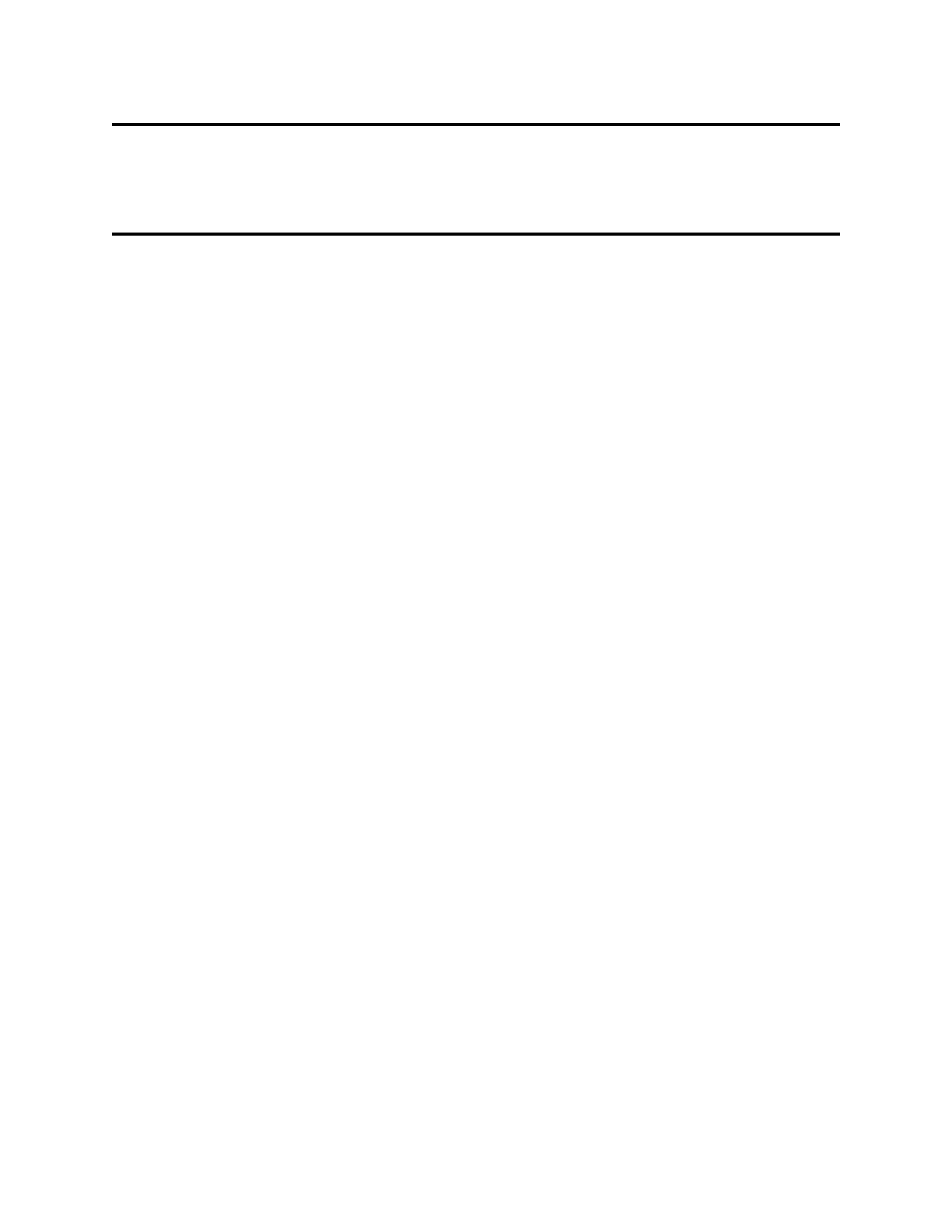
FAIR COINS TEND TO LAND ON THE SAME SIDE THEY STARTED:
EVIDENCE FROM 350,757 FLIPS
František Bartoš1∗, Alexandra Sarafoglou1, Henrik R. Godmann1, Amir Sahrani1, David Klein Leunk2,
Pierre Y. Gui2, David Voss2, Kaleem Ullah2, Malte J. Zoubek3, Franziska Nippold,
Frederik Aust1, Felipe F. Vieira4, Chris-Gabriel Islam5,6, Anton J. Zoubek7, Sara Shabani8,
Jonas Petter1, Ingeborg B. Roos9, Adam Finnemann1,10, Aaron B. Lob2,11, Madlen F. Hoffstadt1,
Jason Nak2, Jill de Ron2, Koen Derks12, Karoline Huth2,13, Sjoerd Terpstra14,
Thomas Bastelica15,16, Magda Matetovici2,17, Vincent L. Ott2, Andreea S. Zetea2, Katharina Karnbach2,
Michelle C. Donzallaz1, Arne John2, Roy M. Moore2, Franziska Assion1,8, Riet van Bork2,
Theresa E. Leidinger2, Xiaochang Zhao2, Adrian Karami Motaghi2, Ting Pan1,9, Hannah Armstrong2,
Tianqi Peng2, Mara Bialas20, Joyce Y.-C. Pang2, Bohan Fu2, Shujun Yang2,
Xiaoyi Lin2, Dana Sleiffer2, Miklos Bognar21, Balazs Aczel22, and Eric-Jan Wagenmakers1
1Department of Psychological Methods, University of Amsterdam,
2Department of Psychology, University of Amsterdam,
3Institute of Economic Law, University of Kassel,
4Faculty of Psychology and Educational Sciences, KU Leuven,
5Chairs of Statistics and Econometrics, Georg August University of Göttingen,
6Centre for Environmental Sciences, Hasselt University,
7Gutenberg School Wiesbaden,
8Department of Psychology, Justus Liebig University,
9Department of Adult Psychiatry, Amsterdam University Medical Centre,
10Centre for Urban Mental Health, University of Amsterdam,
11Cognitive and Behavioral Decision Research, University of Zurich,
12Center of Accounting, Auditing & Control, Nyenrode Business University,
13Department of Psychiatry, Amsterdam UMC, University of Amsterdam,
14Institute for Marine and Atmospheric Research Utrecht, Utrecht University,
15Centre Hospitalier Le Vinatier,
16INSERM, U1028; CNRS, UMR5292, Lyon Neuroscience Research Center,
Psychiatric Disorders: from Resistance to Response Team,
17Research Institute of Child Development and Education, University of Amsterdam,
18Faculty of Behavioral and Movement Sciences, Vrije Universiteit Amsterdam,
19Department of Develomental Psychology, University of Amsterdam,
20Behavioural Science Institute, Radboud University,
21Doctoral School of Psychology, ELTE Eotvos Lorand University,
22Institute of Psychology, ELTE Eotvos Lorand University
*Correspondence concerning this article should be addressed to František Bartoš at
[email protected].
For all but the last four positions, the authorship order aligns with the number of coin flips contributed.
arXiv:2310.04153v2 [math.HO] 10 Oct 2023