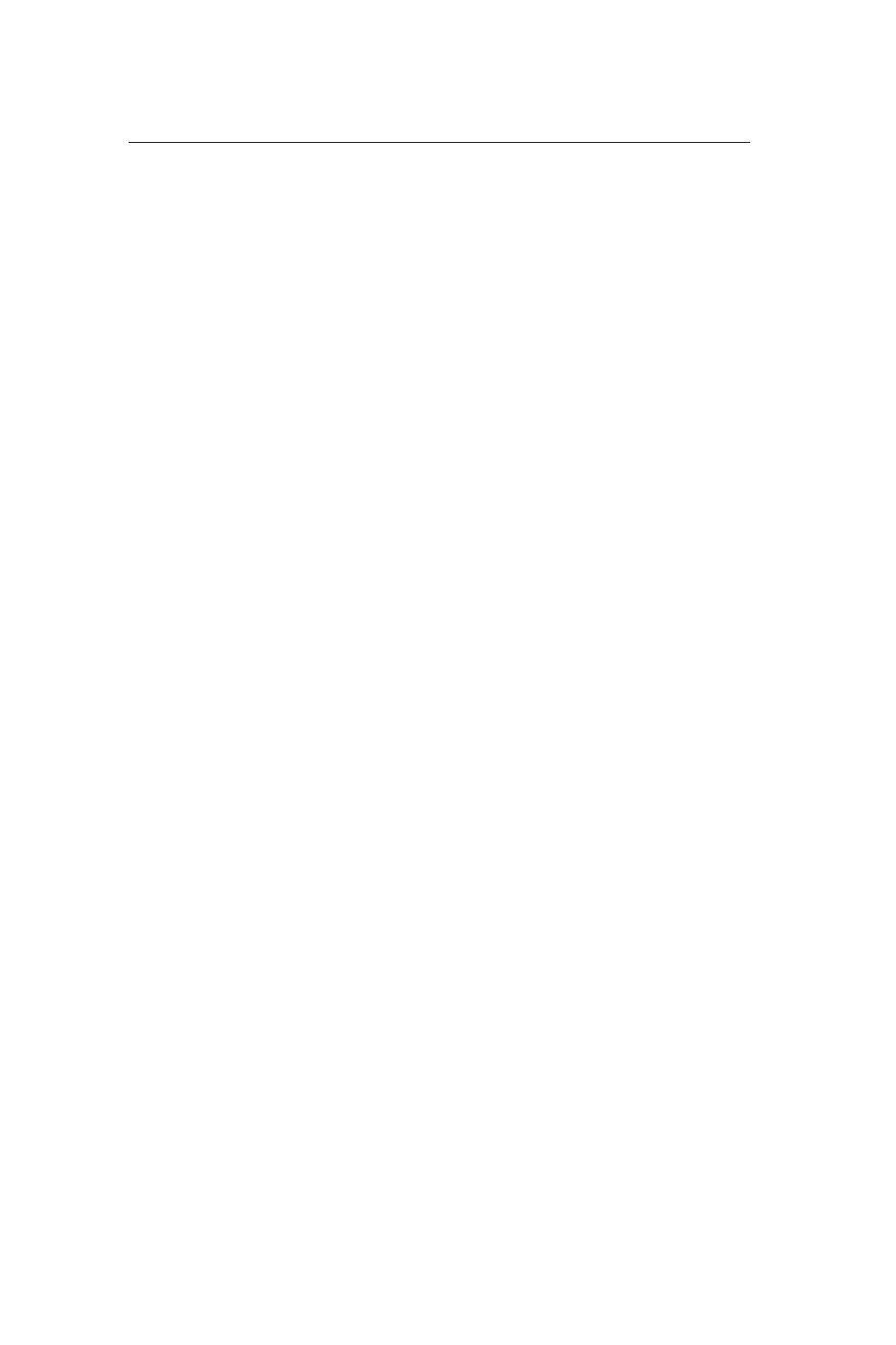
2 South Asian Journal of Human Resources Management
However, no moderating effect of gender or firm size was found in any of the
hypothesised propositions. Finally, policy implications and recommendations for
future researchers are proposed.
Keywords
Actual use, artificial intelligence, Bangladesh, human resource professionals,
intention to use, recruiting talents, UTAUT, SEM-PLS
Introduction
Human resources are considered the strategic resources in business organisations
and the ultimate source of sustainable competitive advantage (Black & Van Esch,
2020; Patel et al., 2019). However, recruiting the right talent is still a far cry
(Vardarlier & Zafer, 2020) because LinkedIn and other traditional methods to
attract and recruit the right employees have yet to be successful (Iqbal, 2018). In
this context, artificial intelligence (AI) can be useful for attracting, retaining and
empowering skilled workers for ensuring a win-win situation for both employers
and applicants (Adikaram et al., 2021; Geetha & Bhanu, 2018; Tambe et al., 2019;
Uddin et al., 2021; Vardarlier & Zafer, 2020). Compared to other methods, AI has
been proven to be beneficial and effective in recruitment (Black & Van Esch,
2020; Hemalatha et al., 2021; O’Connor, 2020; Vardarlier & Zafer, 2020).
In the area of recruitment, AI refers to intelligent machines and software which
behave and act like humans without any human intervention (Lisa & Talla Simo,
2021; Uddin et al., 2021). With the aid of simple filtering algorithms, applicants
are automatically assessed with regard to several factors, such as the university
they attended, and business and industry-wide experience. This simple AI
algorithm allows organisations such as Unilever (Vardarlier & Zafer, 2020),
L’Oréal (Sharma, 2018) and SAT Telecom (Kmail et al., 2015), among others, to
fill employee vacancies faster, economise time and effort, and source appropriate
candidates capable of addressing strategic problems and developing long-term
bonds with new changes (Bhalgat, 2019; Pan et al., 2022; Upadhyay & Khandelwal,
2018). Therefore, using AI can be justified, as the automated system can save time
and costs in hiring the right talent and solve the problems of manual sorting
(Soleimani et al., 2022; Tambe et al., 2019; Vedapradha et al., 2019).
Historically, the recruitment and selection process in Bangladesh in both public
and private sectors is traditional and politically motivated (Absar, 2012; Mahmood
& Nurul Absar, 2015). The large supply of applications to the relatively scarce
employment opportunity for managerial and non-managerial positions causes the
recruiters in the private sector fall prey to nepotism (Mahmood & Nurul Absar,
2015). However, the recruitment and selection process of MNCs in Bangladesh
happens based on the compliance of headquarters office’s directions (Mahmood,
2015). The strength of using AI to recruit in either cases prevents the organisations
from unwanted error and violations in transparent recruitment. However, there
has been minimal use of AI in Bangladesh, although together with the IoT, big