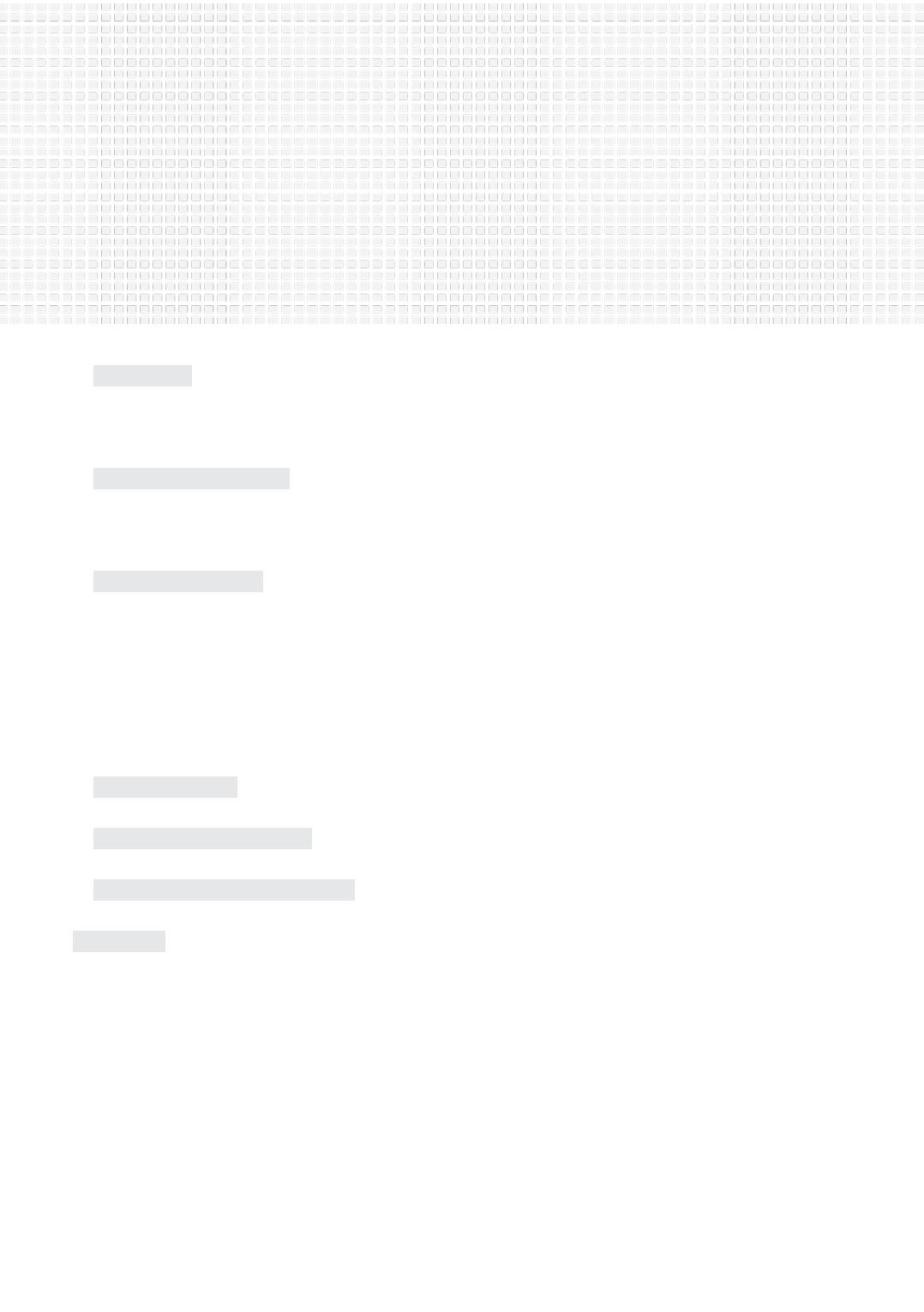
1_Introduction 6
>>1.1 Overview and Goals 6
>>1.2 Procedure and Criteria of Admission 8
2_The Program of Studies 10
>>2.1 Program Structure 10
>>2.2 Indicative Program of Studies 11
3_Course Descriptions 12
>>3.1 Core Courses 12
>>3.2 Elective Courses of Specializations 13
>3.2.1 Computer Science Track 13
>3.2.2 Statistics Track 16
>3.2.3 Business Analytics Track 17
>3.3 Free Elective Courses 19
4_Capstone Project 20
5_Optional Research Project 22
6_Gender Balance in the Program 24
Contact Us 26