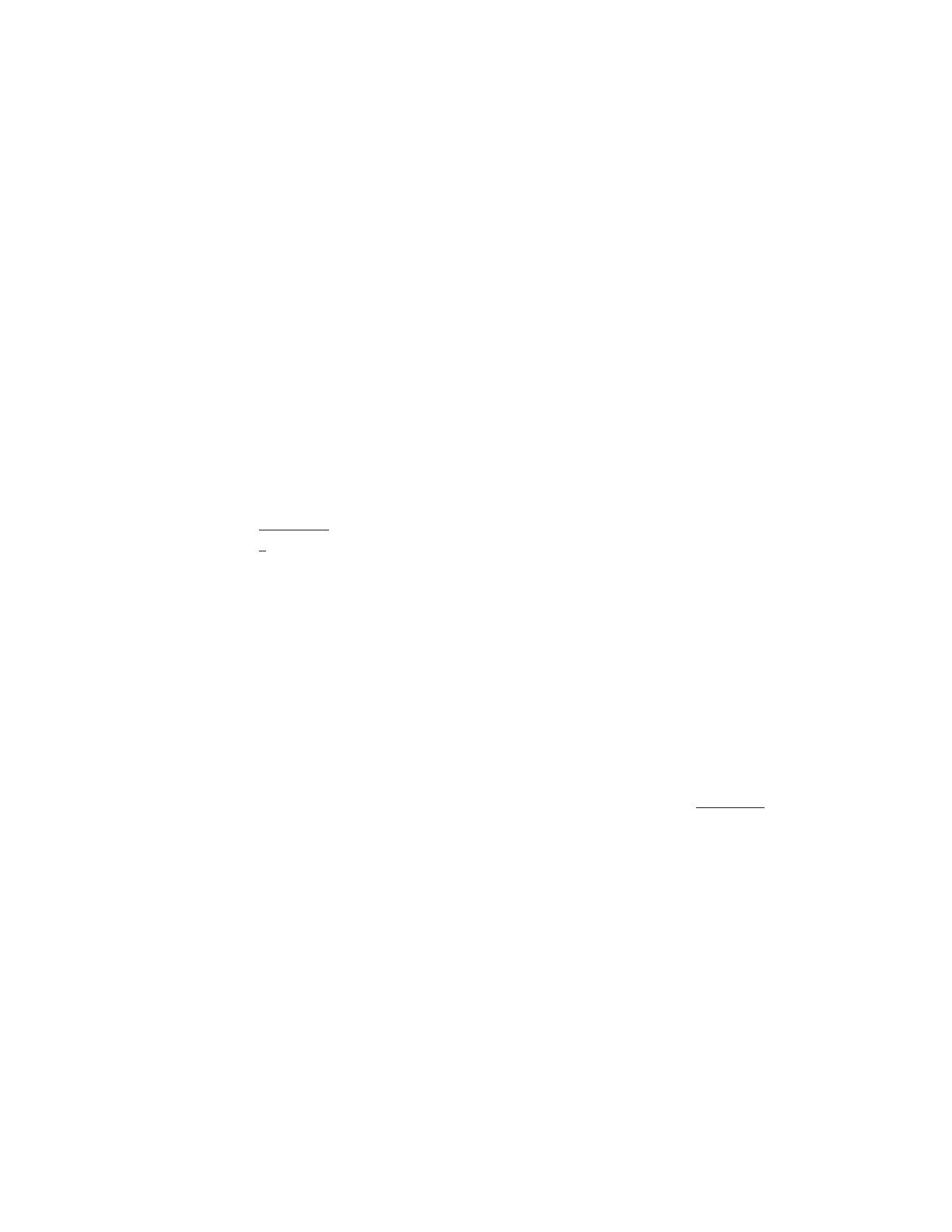
perature, humidity, ambient light, etc.); contamination of sample
or equipment (e.g., carryover and ambient contamination); vari-
ations in purity of solvent, reagent, catalyst, etc.; stability and
age of sample, analyte, or matrix; and warm-up or cool-down
effects (tendency to drift over time). The simplest strategy for
estimating bias is to measure a traceable (known) standard and
then compute the difference between Mand T*:
M⫺T*⫽B⫹Z
The uncertainty in this case is assumed to be small, although
in practice there may be situations in which this assumption is
inappropriate. If random uncertainty (Z) is negligible (i.e., Z⬇
0), then M⫺T* will provide an estimate of bias (B). If Zis not
negligible, it can be observed and quantified by repeatedly mea-
suring the same test specimen (if the measurement process is not
destructive). This may be part of a QA/QC procedure.
b. Repeatability: Repeatability (also called intrinsic measure-
ment variability) is the smallest amount of variation that remains
in a measurement system when repeatedly measuring the same
specimen while preventing controllable sources of variability
from affecting results. It is quantified by the repeatability stan-
dard deviation (
RPT
), which can be obtained by pooling sample
standard deviations of measurements of Jspecimens:
RPT
⫽
冑
1
J
䡠
冘
i⫽1
J
RPT,i
2
Repeatability is considered an approximate lower boundary to
the standard deviation experienced in practice. The repeatability
standard deviation sometimes is used to compute uncertainty
intervals (⫾U) (referred to as ultimate instrument variability)
based on the Student’s tdistribution (⫾U⫽⫾ks
RPT
).
Common sense and experience demonstrate that repeatability
is an overly optimistic estimate of uncertainty for routine mea-
surements, which are subject to many sources of bias and vari-
ability that are intentionally eliminated or restrained during a
repeatability study. The uncertainty in both Band Zare greater
in routine measurements.
c. Reproducibility: Reproducibility is the variation in a mea-
surement system that occurs when repeatedly measuring a sam-
ple while allowing (or requiring) selected sources of Bor Zto
affect results. It is quantified by the reproducibility standard
deviation (
RPD
), accompanied by a list of known applicable
sources of B and Z, and notes on which sources varied.
Barring statistical variation (i.e., variation in estimates of
variability, such as the noisiness in sample standard deviations),
RPD
is always greater than
RPT
because it has more compo-
nents. Typically, one or more of the following varies in a
reproducibility study: instrument, analyst, laboratory, or day.
Preferably, design a study tailored to the particular measurement
system (see 1030B.7). If the sample varies, compute
RPD
sep-
arately for each sample, then pool the homogeneous results.
Treat factors that vary as random factors and assume they are
independent normal random variables with a mean of zero.
However, this assumption can often be challenged if the sample
and possibly the target populations are small (even identical);
there may be a question of “representativeness.” Suppose, for
example, that out of 20 laboratories (or analysts or instruments)
that can do tandem mass spectrometry for a particular analyte
and matrix, only six report usable measurements. It is hard to
know how representative these six are— especially after a post-
study ranking and exclusion process—and whether the Bsofthe
20 are normally distributed (probably not discernible from six
measurements, even if they are representative).
It may be more appropriate to treat each factor with few
known values (e.g., laboratories) as fixed factors, which have
fixed effects. In other words, each laboratory, analyst, instru-
ment, or day has a different bias, but its distribution is assumed
to be unknown (or unknowable), so a small sample cannot be
used to estimate distribution parameters, particularly standard
deviation. For example, assuming that variables are random, are
normal, and have a mean of zero may be inappropriate in an
interlaboratory round-robin study. Every laboratory has some B,
but it is difficult to characterize because of laboratory anonymity,
the small number of laboratories contributing usable data, etc.
Because of these concerns about assumptions and the potential
ambiguity of its definition, do not report reproducibility unless it
is accompanied with the study design, a list of known sources of
Band Z, and notes on which sources varied.
7.
Gage Repeatability and Reproducibility, and the
Measurement Capability Study
The Gage repeatability and reproducibility (Gage R&R) ap-
proach combines repeatability and reproducibility.
4
It treats all
factors (including B) as random and is based on the simplest
nontrivial model:
Z⫽Z
RPT
⫹Z
L
where:
Z
RPT
⫽normally distributed random variable with mean equal to
zero and variance equal to
RPT
2
, and
Z
L
⫽normally distributed random variable with mean equal to
zero and with the variance of the factor (e.g., interlabora-
tory) biases,
L
2
.
The overall measurement variation then is quantified by
E
⫽
RPD
⫽
冑
RPT
2
⫹
L
2
Estimates for
RPT
and
RPD
usually are obtained by conduct-
ing a nested designed study and analyzing the components of the
results’ variance. This approach can be generalized to reflect
good practice in conducting experiments. The following mea-
surement capability study (MCS) procedure is recommended.
The goal is not necessarily to quantify the contribution of every
source of Band Z, but rather to study those considered important
via systematic error budgeting.
When performing an MCS to assess Uvia systematic error
budgeting, begin by identifying sources of Band Zthat affect E.
This can be done with a cause-and-effect diagram—perhaps with
source categories of equipment, analyst, method (procedure and
algorithm), material (aspects of test specimens), and environment.
Select sources to study either empirically or theoretically.
Typically, study sources that are influential, that can be varied
during the MCS, and that cannot be eliminated during routine
DATA QUALITY (1030)/Measurement Uncertainty
3
DATA QUALITY (1030)/Measurement Uncertainty